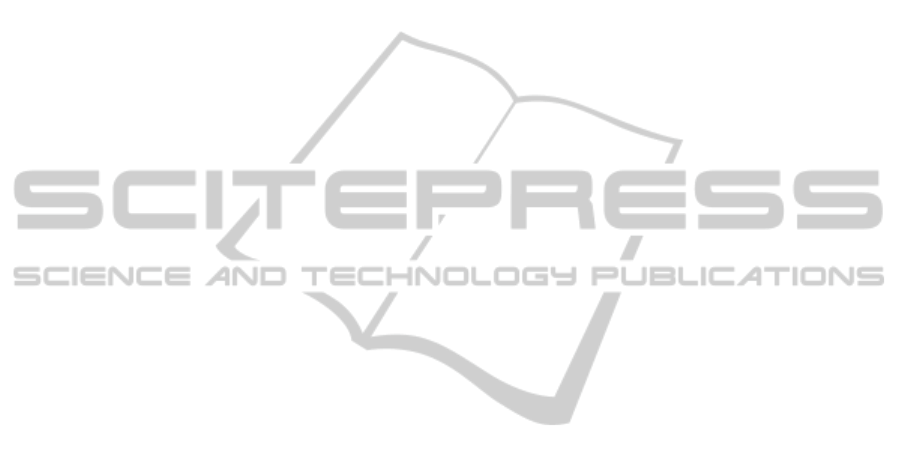
value range, where a clear distinction between base-
line noise and signal during muscle contraction can
be achieved. Without the minimum the normalization
factor could degrade to values lower than the baseline
noise values. This would lead to a value of close to 1.0
after the normalization in ranges where no movement
is performed.
The normalization needs to be calibrated, i.e.,
there has to be a contraction of each muscle to ob-
tain meaningful values from the function. The control
signals for the orthosis are obtained using a threshold
algorithm similar to the on used in (DiCicco et al.,
2004). Two thresholds are used for determining the on
and off phases of each muscle. The on threshold was
set to 0.4 and the off threshold to 0.3. This hystere-
sis is used to prevent continuous on and off switch-
ing if the normalized EMG signal has a value close
to the threshold. For the off threshold an additional
time constrain is used. The mode is only switch from
on to off, if the signal is continuously below the off
threshold for 20 ms. Further a resting time of 500 ms
in between a direction switch has to be maintained.
As a last step we had to decide which of the two mea-
sured muscles should be preferred, in case that both
were active, we decide to set both signals to 0 and
therefore to decide for none of the muscle or move-
ment directions.
With this preprocessing in combination with the
threshold algorithm, we are able to create three dif-
ferent control commands for the orthosis: (1) to flex
the arm (M. biceps brachii is active), (2) to stretch
the arm (M triceps brachii is active), and (3) to relax
(none of both muscles is active). The control signals
are derived on the computer acquiring the EMG data
and send to the orthosis via USART. All described pa-
rameters were chosen by empirical testing on EMG
data recorded with the orthosis attached to the sub-
jects arm running in free-runing-mode see Section 4.
In Figure 6 the processing of EMG data, and the
thresholding result for an arm flexion and extension
are shown. In the top, the raw EMG signals from the
biceps (blue) and triceps (green) are shown, the mid-
dle illustrates the filtered and normalized EMG sig-
nals, and finally in the bottom, the outcome from the
thresholding is shown. The three vertical lines, dot-
ted, dashed, and solid denote time points, where the
subject was asked to stretch, flex, and relax the arm re-
spectively. The stimuli for those three actions where
presented on a monitor and marked in the EMG data.
The obtained control signals can be used directly to
operate the orthosis, e.g., support the users arm move-
ment.
6 CONCLUSIONS AND
OUTLOOK
In this work we presented an active orthosis, its pos-
sible application, design and mechanics, and control.
To summarize, the system allows to support self ini-
tiated movement that are normally executed by both
upper arm muscles M. biceps brachii or M. triceps
brachii. Patients that are not able to effectively control
both muscles can be supported for individual move-
ments. This can be achieved by detecting EMG onset
activity. If EMG activity onset is detected, the or-
thosis actively executes the directed movement corre-
sponding to the active muscle.
The next developmental step is to adapt the
strength of support with respect to the strength of
muscle activity. To allow this, different methods
for EMG analysis have to be applied. These meth-
ods (Ajiboye and Weir, 2005) allow to correlate mus-
cle activity strength with the recorded signal and thus,
enable to adapt the support of the orthosis to the needs
of the patient and state of therapy.
Further, we established a collaboration with a clin-
ical partner. Supported by the medical specialists, the
design of the orthosis will be improved in order to
follow further sanitary and medical guidelines and to
define proper application scenarios. From the con-
trol side, it is important to find a way to measure the
resistance of the human-robot interaction in order to
recognize unvoluntary muscle activity due to spastic-
ity. Further, the possibility of defining torque curves
will be analysed. In specific, the development of the
applied torque by the user depending on the angular
position of the joint can be observed and defined as a
mathematical function. Using the inverse of this curve
may lead to a more natural movement.
Finally, user-friendliness elements have to be con-
sidered in the future. The possibility for therapist (and
patients) to adapt important parameters online in an
easy way, by means of some sort of intuitive user in-
terface is the goal.
ACKNOWLEDGEMENTS
This work was supported by the German Bundesmin-
isterium fur Bildung und Forschung (BMBF,grant
FKZ 01IW10001).
REFERENCES
Ajiboye, A. and Weir, R. (2005). A heuristic fuzzy logic ap-
proach to emg pattern recognition for multifunctional
AnEMG-basedAssistiveOrthosisforUpperLimbRehabilitation
327