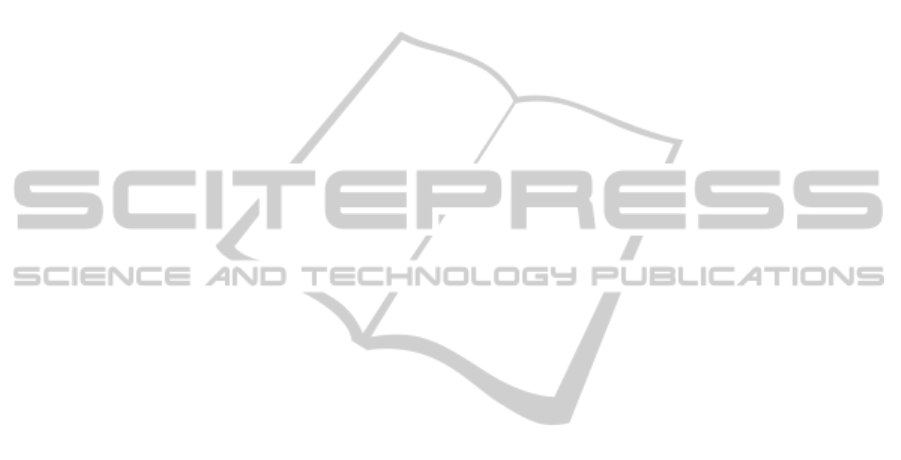
caches are needed to satisfy the demand and regional
differences (Brodersen et al., 2012). Our methodol-
ogy and analysis could be used to help design, con-
figure, and deploy any category specific UGC site.
As future work, we are in the process of building a
complete workload generator that encompasses more
aspects of user-generated content video requests. In
particular, we will incorporate category-specific in-
troduction of new content over time to drive simula-
tions and/or prototype content distribution networks
to evaluate different design policies for storing and
delivering videos.
REFERENCES
Abhari, A. and Soraya, M. (2010). Workload Generation
for YouTube. Multimedia Tools and Applications,
46(1):91–118.
Borghol, Y., Mitra, S., Ardon, S., Carlsson, N., Eager, D.,
and Mahanti, A. (2011). Characterizing and Mod-
elling Popularity of User-Generated Videos. Perfor-
mance Evaluation, 68:1037–1055.
Brodersen, A., Scellato, S., and Wattenhofer, M. (2012).
YouTube Around the World: Geographic Popularity
of Videos. In World-Wide Web 2012, pages 241–250,
Lyon, France.
Broxton, T., Interian, Y., Vaver, J., and Wattenhofer, M.
(2010). Catching a viral video. In IEEE Data Min-
ing Workshops, pages 296–304, Sydney, Australia.
Cha, M., Kwok, H., Rodriguez, P., Ahn, Y., and Moon, S.
(2009). Analyzing the Video Popularity Characteris-
tics of Large-Scale User Generated Content Systems.
IEEE/ACM Trans. Netw., 17(5):1357–1370.
Cheng, X., Dale, C., and Liu, J. (2007). Understanding
the Characteristics of Internet Short Video Sharing:
YouTube as a Case Study. Technical report, Cornell
University, arXiv e-prints.
Chu, K. K. W. and Wong, M. H. (1999). Fast time-series
searching with scaling and shifting. In ACM PODS
1999, pages 237–248, Philadelphia, PA.
Ding, Y., Du, Y., Hu, Y., Liu, Z., Wang, L., Ross, K., and
Ghose, A. (2011). Broadcast Yourself: Understanding
YouTube Uploaders. In ACM IMC 2011, pages 361–
370, Berlin, Germany.
Figueiredo, F., Benevenuto, F., and Almeida, J. (2011). The
Tube over Time: Characterizing Popularity Growth of
Youtube Videos. In ACM WSDM 2011, pages 745–
754, Hong Kong, China.
Gember, A., Anand, A., and Akella, A. (2011). A Compar-
ative Study of Handheld and Non-handheld Traffic in
Campus Wi-Fi Networks. In PAM 2011, pages 173–
183, Atlanta, GA.
Gill, P., Arlitt, M., Li, Z., and Mahanti, A. (2007). Youtube
Traffic Characterization: A View From the Edge. In
ACM IMC 2007, pages 15–28, San Diego, CA.
Gummadi, K. P., Dunn, R. J., Saroiu, S., Gribble, S. D.,
Levy, H. M., and Zahorjan, J. (2003). Measure-
ment, modeling, and analysis of a peer-to-peer file-
sharing workload. In ACM SOSP 2003, pages 314–
329, Bolton Landing, NY.
Khemmarat, S., Zhou, R., Gao, L., and Zink, M. (2011).
Watching User Generated Videos with Prefetching. In
ACM MMSYS 2011, pages 187–198, San Jose, CA.
Labovitz, C., Iekel-Johnson, S., McPherson, D., Oberheide,
J., and Jahanian, F. (2010). Internet Inter-Domain
Traffic. In ACM SIGCOMM 2010, pages 75–86, New
Delhi, India.
Maier, G., Schneider, F., and Feldmann, A. (2010). A First
Look at Mobile Hand-held Device Traffic. In PAM
2010, pages 161–170, Zurich, Switzerland.
Siersdorfer, S., Chelaru, S., Nejdl, W., and Pedro, J. S.
(2010). How Useful are Your Comments?: Analyz-
ing and Predicting YouTube Comments and Comment
Ratings. In World-Wide Web 2010, pages 891–900,
Raleigh, NC.
Szabo, G. and Huberman, B. (2010). Predicting the popu-
larity of online content. CACM, 53(8):80–88.
Yang, J. and Leskovec, J. (2011). Patterns of temporal vari-
ation in online media. In ACM WSDM 2011, pages
177–186, Hong Kong, China.
Zink, M., Suh, K., Gu, Y., and Kurose, J. (2009). Char-
acteristics of YouTube Network Traffic at a Campus
Network - Measurements, Models, and Implications.
Computer Networks, 53(4):501–514.
WEBIST2013-9thInternationalConferenceonWebInformationSystemsandTechnologies
242