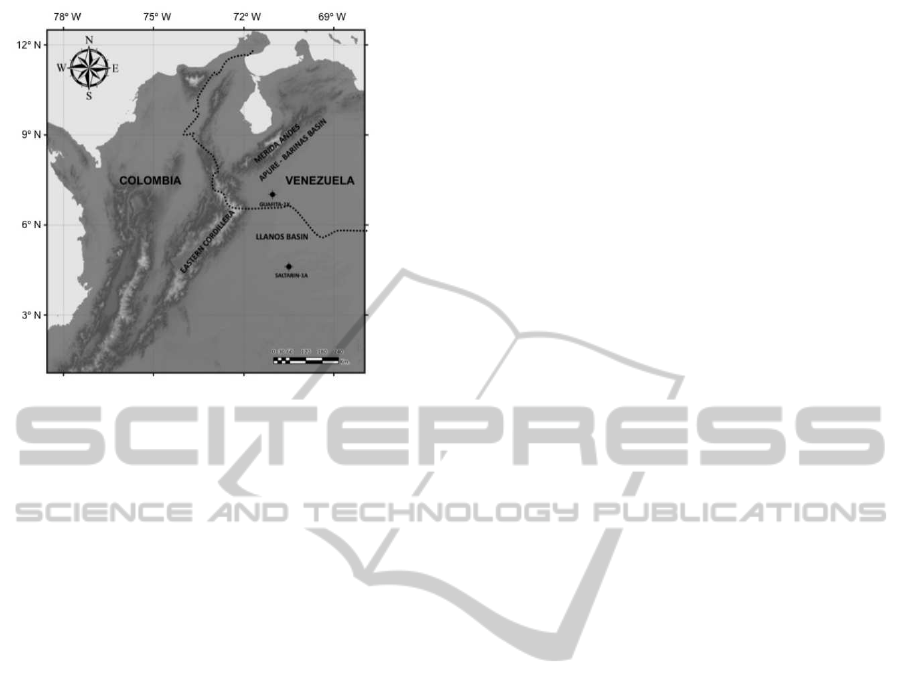
Figure 1: Geographical setting of the stratigraphic well
Saltar´ın 1A in Colombia (Llanos Foreland Basin)
2 STRATIGRAPHIC SETTING
The deepest formation studied in stratigraphic well
Saltar´ın 1A is Carbonera that includes a lower sand-
stone unit (654.6 to 670 m) accumulated in a flu-
vial system, a middle mudstone unit accumulated in
a lacustrine system (608.2 to 654.6 m), and an upper
sandstone unit that records sedimentation in a fluvial-
deltaic system (546.9 to 608.2 m). Overlying Car-
bonera is the Le´on Formation (441.8 to 546.9 m), a
muddy sequence of sediments from a fresh-water la-
custrine system.
On top of Le´on lies the Guayabo formation that
was divided in 6 lithological units (Bayona et al.,
2008): G1 (388 to 441.8 m) and G2 (312.9 to 388
m), the two lower units, consist of green-colored lam-
inated mudstones grading to sandstones interbedded
with light-colored massive mudstones with ferrugi-
nous nodules. These lithologies were interpreted as
the sedimentation from a fluvio-deltaic system chang-
ing to more continental sediment accumulation in flu-
vial floodplains. G3 (271.5 to 312.9 m) and G4
(205.5 to 271.5 m), the overlying units, are domi-
nantly mudstones and siltstones that accumulated in
fluvial flood plains. The unit G3 has more evidence
of subaerial exposure (light-colored mudstones, for-
mation of ferruginous nodules), whereas preservation
of coal beds and laminated mudstones in unit G4 in-
dicates less subaerial exposure of the flood plains. G5
(81.6 to 205.5 m) consists of feldspar-rich sandstones
that record the filling of fluvial channels. G6 (0 to
81.6 m), the uppermost unit of the Guayabo forma-
tion, records a change to floodplain with evidence of
subaerial exposure.
3 METHODS
For the characterization of the different lithostrati-
graphic units, through the inference of S − ratio,
we used a hybrid Adaptive Neuro Fuzzy Inference
System (ANFIS) with five layers that can be in-
terpreted as a neural network with fuzzy parame-
ters. ANFIS is equivalent, under some constrains,
to a Takagi, Sugeno, Kang (TSK) model (Finol and
Jing, 2002). To train our ANFIS we used S − ratio
(IRM
−0.03T
/SIRM
+3T
), as output and χ as input vari-
able.
We introduced the χ training data in either
semilogarithmic or direct form (i.e. log(χ) or χ re-
spectively). Tests were also carried out using different
combinations and numbers of fuzzy rules. Member-
ship functions employed in all the trials were either
linear, triangular, bell, pi, or gaussian. In each case
inferred S− ratio values were compared with their ex-
perimental counterparts. To quantify the performance
of the inference, we applied the R
2
between inferred
and experimental S − ratio data, and the Root Mean-
Square Error (RMSE) values.
4 RESULTS
For to ANFIS training we use a Gaussian membership
function, the fuzzy rules was adjusted from 2 to 4, in
order to monitor a possible improvement of the in-
ference. Also we pruve that non-linear mathematical
form of the function that relates the value of S-ratio
to the magnetite weight percentage for a array of syn-
thetic samples systematically mixed from magnetite
and hematite (Heslop, 2009; Frank and Nowaczyk,
2008).
We trained the ANFIS separately with the χ and
S− ratio experimental values from Guayabo, Len and
Carbonera and assessed the set of fuzzy rules obtained
in each case in all the three formations involved (see
Table 1). The figure 2 shows the results of some of
these inferences, for the first case in which the AN-
FIS was trained using the experimental data from the
Guayabo sandstones only. The qualitative examina-
tion of figure 2a shows that the fuzzy rules, obtained
by training the ANFIS with Guayabo’s data, give a
reasonably good inference upon this Formation itself.
However, that is not true when these same rules are
applied to Le´on and Carbonera (figures 2b and c re-
spectively). The RMSE and R
2
values (Table 2) con-
firm this observation.
Similar tests were repeated by training the net only
with experimental data from the mudstones of Le´on
and Carbonera .These results are summarized in Ta-
CharacterizationofLithostratigraphicUnitsusingNeuroFuzzySystemAnalysesAppliedtoRockMagneticData
687