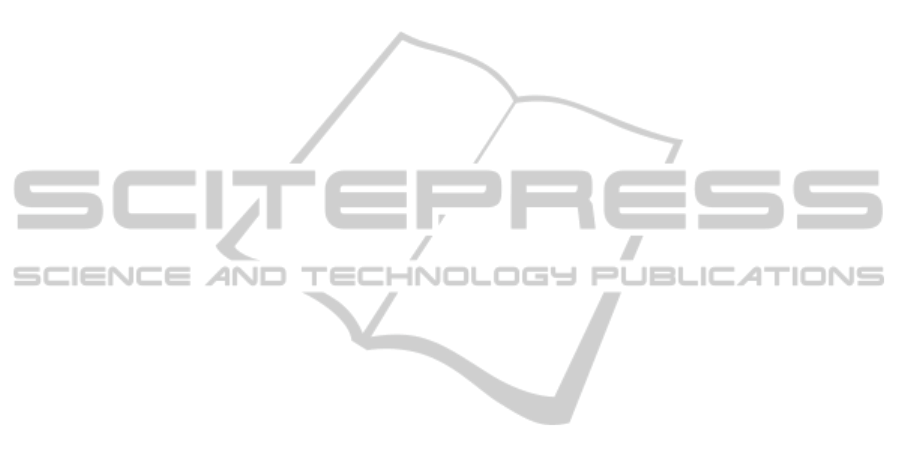
from everyday life and automatically annotated us-
ing the additional data of submeters. Since we aim
for a fully automated approach we additionally have
to automate the decision whether an event originated
from the state change of an appliance or from noise
in the power signal. We incorporated this decision
into the classification problem by adding additional
classes for noise events.
We addressed privacy indirectly by designing our
system in a way that allows us to train classifiers on
a per household basis. This means that all personal
data is processed in-house and never uploaded or pro-
cessed anywhere else.
One drawback of our system is, that all classifiers
have to be retrained if a new appliance is added to the
household. In such a case the data of most appliances
can be reused, but during a short setup phase patterns
of the new appliance must be gathered. So at least
one submeter should permanently be available in the
household whereas most other submeters can be re-
moved after the initial setup.
ACKNOWLEDGEMENTS
This work has been supported by funds of the
Federal Ministry of Economy and Technology in
the E-Energy project eTelligence, project number
01MR08007A.
REFERENCES
Baranski, M. (2006). Energie-Monitoring im privaten
Haushalt. PhD thesis, University of Paderborn.
Hart, G. (1992). Nonintrusive appliance load monitoring.
Proceedings of the IEEE, 80(12):1870 –1891.
Jiang, L., Luo, S., and Li, J. (2012). An Approach of House-
hold Power Appliance Monitoring Based on Machine
Learning. 2012 Fifth International Conference on
Intelligent Computation Technology and Automation,
pages 577–580.
Klingenberg, T. (2010). Smart Submetering - Effizien-
ter Einsatz von Submetern zur Aktivit
¨
atsbestimmung
in Privathaushalten mit Hilfe adaptiver Lernverfahren.
Master’s thesis, University of Oldenburg.
Kramer, O., Wilken, O., Beenken, P., Hein, A., H
¨
uwel, A.,
Klingenberg, T., Meinecke, C., Raabe, T., and Son-
nenschein, M. (2012). On ensemble classifiers for
nonintrusive appliance load monitoring. 7th Inter-
national Conference on Hybrid Artificial Intelligence
Systems (HAIS), pages 322–331.
Leeb, S. B., Shaw, S. R., and Kirtley, J. L. (1995). Transient
Event Detection in Spectral Envelope Estimates. IEEE
Transactions on Power Delivery, 10(3):1200–1210.
Lin, Y.-h. and Tsai, M.-S. (2011). Applications of hierarchi-
cal support vector machines for identifying load oper-
ation in nonintrusive load monitoring systems. 2011
9th World Congress on Intelligent Control and Au-
tomation, pages 688–693.
Mattern, F., Staake, T., and Weiss, M. (2010). ICT for
green: how computers can help us to conserve en-
ergy. International Conference on Energy-Efficient
Computing and Networking.
Matthews, H. S., Soibelman, L., Berges, M., and Goldman,
E. (2008). Automatically disaggregating the total elec-
trical load in residential buildings: a profile of the re-
quired solution. In International Workshop on Intelli-
gent Computing in Engineering, page 381389.
Mittelsdorf, M. (2012). Evaluierung des Einflusses
von Merkmalsauswahl und Abtastrate auf die
Ger
¨
ateerkennung mit Support-Vektor-Maschinen.
Master’s thesis, University of Oldenburg.
Onoda, T., Murata, H., and Ratsch, G. (2002). Experimen-
tal analysis of support vector machines with different
kernels based on non-intrusive monitoring data. Neu-
ral Networks, 2002., pages 2186–2191.
Patel, S., Robertson, T., Kientz, J., Reynolds, M., and
Abowd, G. (2007). At the flick of a switch: De-
tecting and classifying unique electrical events on the
residential power line (nominated for the best paper
award). In Krumm, J., Abowd, G., Seneviratne, A.,
and Strang, T., editors, UbiComp 2007: Ubiquitous
Computing, volume 4717 of Lecture Notes in Com-
puter Science, pages 271–288. Springer Berlin Hei-
delberg.
Pihala, H. (1998). Non-intrusive appliance load monitor-
ing system based on a modern kWh-meter. Technical
Research Centre of Finland.
Raabe, T., Sonnenschein, M., Beenken, P., H
¨
uwel, A., and
Meinecke, C. (2012). Energieberatung in haushal-
ten auf basis des smartmetering.
¨
Okologisches
Wirtschaften, 1:46–50.
Weiss, M., Staake, T., Mattern, F., and Fleisch, E. (2012).
Powerpedia: changing energy usage with the help of
a community-based smartphone application. Personal
and Ubiquitous Computing, 16(6):655–664.
Zeifman, M., Akers, C., and Roth, K. (2011). Nonintrusive
appliance load monitoring (nialm) for energy control
in residential buildings. International Conference on
Energy Efficiency in Domestic Appliances and Light-
ing (EEDAL), Copenhagen.
Zeifman, M. and Roth, K. (2011). Nonintrusive appliance
load monitoring: Review and outlook. IEEE Transac-
tions on Consumer Electronics, 57(1):76–84.
SMARTGREENS2013-2ndInternationalConferenceonSmartGridsandGreenITSystems
158