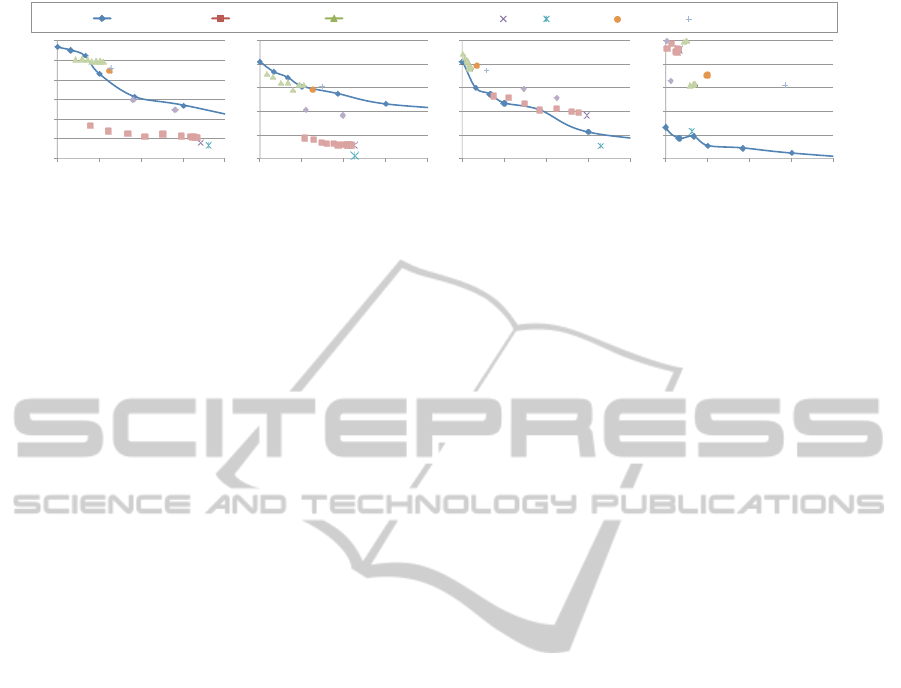
0"
10"
20"
30"
40"
50"
60"
0" 0,1" 0,2" 0,3" 0,4" 0,5" 0,6"
Error$(m)$
Sent$/$sec$
Simple"Sampling" Space"Sampling" Space"Sampling"con"Stop" MB" MB"Stop" MB"Turn" MB"stop"Turn"
0"
10"
20"
30"
40"
50"
60"
0" 0,1" 0,2" 0,3" 0,4" 0,5" 0,6"
Error$(m)$
Sent$/$sec$
Simple"Sampling" Space"Sampling" Space"Sampling"con"Stop" MB" MB"Stop" MB"Turn" MB"stop"Turn"
5"
10"
15"
20"
25"
30"
35"
0,05" 0,1" 0,15" 0,2" 0,25"
Error$(%)$
Sent$/$sec$
5"
15"
25"
35"
45"
55"
0,05" 0,1" 0,15" 0,2" 0,25"
Error$(%)$
Sent$/$sec$
5"
15"
25"
35"
45"
55"
0,05" 0,1" 0,15" 0,2" 0,25"
Error$(%)$
Sent$/$sec$
20"
40"
60"
80"
100"
120"
0,05" 0,1" 0,15" 0,2" 0,25"
Error$(%)$
Sent$/$sec$
Figure 3: Performance detail graphs for data reduction techniques in the four scenarios
on the detailed topography, traffic volumes, vehicle
speeds and flows representing typical traffic condi-
tions in such environments.
In order to evaluate the actual effectiveness of
each technique, we will consider the communication
cost (messages sent per second) together with the av-
erage error produced by the CC in computing the av-
erage speeds. The error is computed as a mean of
the ”distances” between the expected values and the
actual values (as available from the complete simu-
lation data). We plotted the achieved results in Fig-
ure 3. All techniques were tested with a large variety
of values for their distinctive parameters, producing a
series of points in the graph, and in all their respec-
tive variants (including the “stop” ones, sending stop
and restart information, and the “turn” ones, send-
ing the needed data each time a turn is identified in
the vehicle trajectory). Note that, since space sam-
pling achieved worse results than simple sampling for
all scenarios, for a better readability space sampling
results are not reported in the graphs. Starting our
analysis from the BO and RO scenario, we can see
that the flow-based technique (without turn option) is
able to produce the most satisfying performances, sig-
nificantly decreasing the simple sampling error level.
Indeed, the regularity/predictability of the traffic and
the infrequent stops performed by the vehicles are
well suited to such kind of techniques, while in TP
the higher number of stops makes their effectiveness
less evident. Finally, in BJ, which is a very complex
scenario, with very irregular traffic flows (also due to
the conspicuous presence of different vehicle types),
the error levels of most advanced techniques are quite
high: in this case, simple sampling appears the sim-
plest and most effective option.
7 CONCLUSIONS
In this paper we presented a technological framework
to efficiently support data management in modern In-
telligent Transportation Systems (ITSs). Data is col-
lected from various sources and stored in an Extended
DBMS (EDBMS) that guarantees efficient storage
and access to a variety of recent/historical/static data.
To the authors’ knowledge, no proposal exists that
merges natively DBMS and DSMS paradigms.
The EDBMS’s transactional storage manager ex-
ploits a new type of table, called streaming table, to
store live as well as past streamed data. Streaming
tables are subjected to continuous writes and support
both CQs and OTQs. The EDBMS provides query
capabilities that span streaming tables as well as tra-
ditional relational tables in a transparent way. As to
scalability issues related to the management of huge
amounts of incoming data, we experienced the em-
ployment of V2I data reduction techniques in the data
acquisition phase. A preliminary experimental evalu-
ation on the standard Linear Road ITS benchmark and
along various simulated traffic scenarios has shown
the storage/query efficiency offered by the streaming
table implementation and the effectiveness of the em-
ployed data reduction techniques.
REFERENCES
Abadi et. al., D. (2003). Aurora: a new model and architec-
ture for data stream management. VLDBJ, 12(2):120–
139.
Arasu, A., Babu, S., and Widom, J. (2006). The CQL
continuous query language: semantic foundations and
query execution”. VLDB J., 15(2):121–142.
Arasu, A., Cherniack, M., Galvez, E., Maier, D., Maskey,
A., Ryvkina, E., Stonebraker, M., and Tibbetts, R.
(2004). Linear Road: A Stream Data Management
Benchmark. In Proc. of VLDB, pages 480–491.
Ayala, D., Lin, J., Wolfson, O., Rishe, N., and Tanizaki, M.
(2010). Communication Reduction for Floating Car
Data-based Traffic Information Systems. In Proc. of
GEOPROCESSING, pages 44–51.
Botan, I., Alonso, G., Fischer, P., Kossmann, D., and Tat-
bul, N. (2009). Flexible and scalable storage manage-
ment for data-intensive stream processing. In Proc. of
EDBT, pages 934–945.
Carafoli, L., Mandreoli, F., Martoglia, R., and Penzo, W.
(2012). Evaluation of data reduction techniques for
vehicle to infrastructure communication saving pur-
poses. In Proc. of IDEAS, pages 61–70.
Cotton, B. (2011). Moving citizens in the smarter city -
SMARTGREENS2013-2ndInternationalConferenceonSmartGridsandGreenITSystems
220