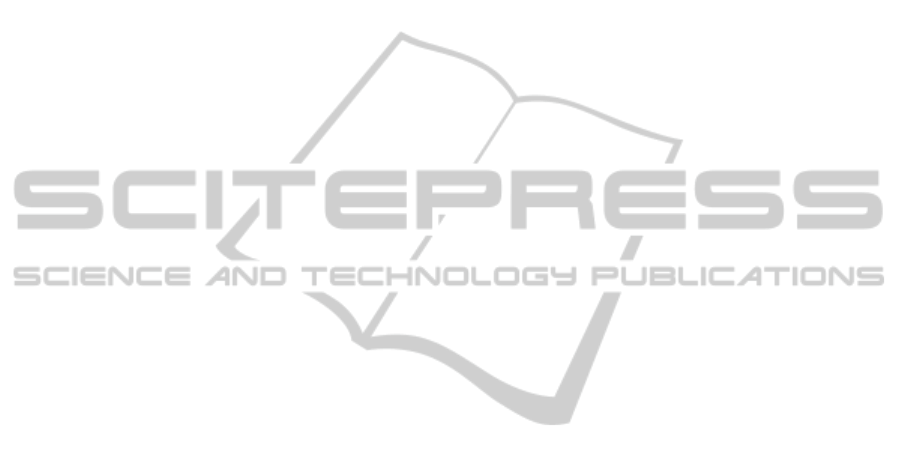
Bostrom, R. P. and Huber, M. 2010. End-User Learning
Methods: What we know, Need to Know, The
DATABASE for Advances in Information Systems
41(4), pp. 9-39.
Bostrom, R. P., Olfman, L. and Sein, M. K. 1990. The
Importance of Learning Style in End-User Learning,
MIS Quarterly 14(1), pp. 101-119.
Davis, F. D. 1989. Perceived usefulness, Perceived ease
of use and user acceptance of information technology,
Management Information Systems Quarterly, 13(3),
pp. 319-340.
Gupta, S. and Bostrom, R. P. 2009, Technology-Mediated
Learning: A Comprehensive Theoretical Model,
Journal of the Association for Information Systems
10(9), pp. 686-714.
Gupta, S., Bostrom, R.P. and Anson, R. 2010, Do I
matter? The Impact of Individual Differences on
Learning Process, Proceedings of the 2010
SpecialInterest Group on MIS’s 48
th
Annual
Conference on Computer Personnel Research, pp. 112-
120.
Lee, B. C., Yoon, J.O., Lee, I. (2009). Learner’s
Acceptance of e-Learning in South Korea: Theories
and results, Computers and Education (53), pp. 1320-
1329.
McGill. T. J. and Klobas, J.E. (2009). A task-technology
fit view of learning management system impact,
Computers and Education (52), pp. 496-508.
Noguera, J. H. and Watson, E.F. 2004. Effectiveness of
Using an Enterprise System to teach process centered
concepts in business education. Journal of Enterprise
Information Management 17(1), pp. 56-74.
Piccoli, G., Ahmed, R. and Ives, B. (2001). Web-Based
Virtual Learning Environments: A Research
Framework and a Preliminary Assessment of
Effectiveness in Basic IT Skills Training, MIS
Quarterly 25 (4), pp. 401-426.
Pituch, K. and Lee,Y. (2006). The influence of system
characteristics on eLearning use, Computers and
Education 47(2), pp. 222-244.
Roca, J. C. and Gagne, M. (2008). Understanding
eLearning continuance intention in the workplace, A
self-determination theory perspective, Computers in
Human Behavior 24(4), pp. 1585-1604.
Selim,H. M. (2003). An Empirical Investigation of
Student Acceptance of Course Websites, Computers
and Education 53(3), pp. 588-598.
Sun, P. C., Tsai, R. J., Finger, G., Chen, Y. Y., and Yeh,
D. (2008). What drives a successful eLearning? An
Emperical investigation of the critical factors
influencing learner satisfaction, Computers and
Education 50(4), pp. 1183-1202.
Szajna, B. and Mackay, J. M. 1995. Predictors of Learning
Performance in a Computer-User Learning
Environment: A Path-Analytic Study, International
Journal of Human-Computer Interaction 7 (2), pp.
167-185.
Venkatesh, V., Thong, J. Y. L. and Xu, X. 2012,
Consumer Acceptance and Use of Information
Technology: Extending the Unified Theory of
Acceptance and Use of Technology, MIS Quarterly
36(1), pp. 157-178.
Yi, M. Y. and David, F. D. 2003. Developing an
Validating an Observational Learning Model of
Computer Software Learning and Skill Acquisition,
Information Systems Research 14 (2), pp. 146-170.
APPENDIX
The Survey Instrument is below:
Excel Usage Experience (in years) ____Years of Education _____
Access Usage Experience (in years) _______ Gender: M F
How many hours/week on average, did you use MyITLab?___
LO1-I understand how I can navigate Excel and Access
LO2-I am confident I can finish an assigned task with office
LO3-I can use features of Excel and Access to solve problems
UB1-I used all of the available features of MyITLab.
UB2-I used MyITLab a lot compared to other learning system
FC1-I had the resources necessary to use MyITLab
FC2-I had the knowledge necessary to use MyITLab.
FC3-I had all the support necessary to use MyITLab
BI1-I had a favourable attitude towards using MyITLab.
BI2-I never disliked using MyITLab
BI3-I am satisfied with the guidance provided by my
instructor in the learning process.
LC1-I would use MyITLab to learn another application.
LC2-The learning materials provided me with enough
details.
LC3-I am satisfied with the documentation of MyITLab
EE1-It was very easy for me to learn to use MyITLab.
EE2-It was easy to find information about MyITLab
EE3-I found MyITLab to be very easy to use.
LSF1-The output from MYITLab was presented in a
useful format.
LSF2-The information from MyITLab is accurate.
LSF3-MyITLab allowed me to take control of my learning
process
PE1-Using MyITLab enhanced my effectiveness in
learning.
PE2-Using MyITLab increased my productivity in the
course
PE3-I found MyITLab to be very useful
LS1-I am satisfied with the facilities and equipment that
were available for my use in the learning process.
LS2-MyITLab system fits well with the way I like to learn
LS3-I understood the policies around using MyITLab.
IC1-I was motivated to learn as much as I can from this
class.
IC2-I was very interested to take this class.
IC3-I was excited about learning the skills that were
covered
CSEDU2013-5thInternationalConferenceonComputerSupportedEducation
100