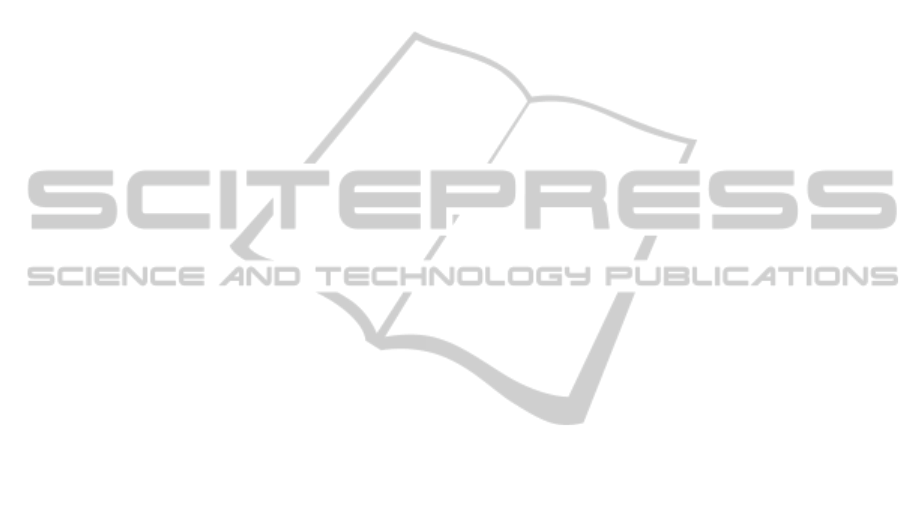
7 CONCLUSIONS
Our results identified factors that promote e-
inclusion. We concluded that a higher rate of
knowledge flow acceleration predicts a higher use
possibility of newly acquired digital skills by
vocational teachers after e-course completion. The
results confirmed the importance of designing
quality e-learning materials and e-environment to
attract e-excluded individuals. Other important
factors that promote e-inclusion are an instructor's
capacity to share knowledge and student's
knowledge level upon course entry. The
implications
of the research should encourage organizations and
enterprises that are responsible for e-course design
to take these factors into account in their future
development efforts.
This study addressed the issues concerned with
the second digital divide. It focused on identifying
relevant factors for narrowing the second digital
divide that inhibited vocational teachers from
applying digital skills in a meaningful way and
showed ways to remove these obstacles so that these
teachers could meaningfully participate in their
professions and enrich their personal lives. In our
study knowledge flow acceleration served as a
potential predictor for the effectiveness of e-course
delivery for the various topics we had assigned.
Moreover, we developed a linear regression model
for predicting practical use probability for designing
post-course surveys that can measure the long-range
impact of a delivery e-course.
ACKNOWLEDGEMENTS
The travel costs and participation fee to conference
was supported by the European Regional
Development Fund project «Development of
international cooperation projects and capacity in
science and technology Riga Technical University»,
Nr. 2DP/2.1.1.2.0/10/APIA/VIAA/003.
REFERENCES
Abrantes, J., Seabra, C. and Lages, L. (2007). Pedagogical
affect, student interest, and learning performance.
Journal of Business Research, 60(9), 960-964.
Cort, P., Härkönen, A. and Volmari, K. (2004). PROFF –
Professionalisation of VET Teachers for the Future.
Luxembourg: Office for Official Publications of the
European Communities.
Deursen, A. J. A. M. v. and Dijk, J. A. G. M. v. (2009).
Improving digital skills for the use of online public
information and services. Government
Information Quarterly, 26, 333-340.
European Commission. (2010). Digital agenda for
Europe: what would it do for me? Retrieved March
15, 2012, from http://europa.eu
European Commission. (2011). Digital agenda
scoreboard 2011. Retrieved March 15, 2012, from
http://ec.europa.eu
FreshMinds and UK Online Centres (2007). Digital
Inclusion. A Discussion of the Evidence Base,
Retrieved March 15, 2012, from
http://www.ukonlinecentres.com
Hargittai, E. (2000). Second-level Digital Divide:
Differences in People’s Online. Retrieved September
1, 2012, from Skillschnm.gmu.edu
Hu, L. and Wang, X. (2008). Studies on the Knowledge
Energy of Learning Organization and its Movement.
Paper presented at the 2008 International Conference
on Wireless Communications, Networking and Mobile
Computing, WiCOM 2008. Retrieved March 15, 2012,
from www.scopus.com
Lerchner, A., Camera, G., L. and Richmond, B. (2007).
Knowing Without Doing. Nature Neuroscience, 10(1).
Retrieved September 1, 2012, from
http://ebookbrowse.com
McLean, R. (2006). A tale of two e-citizens: a
consideration of engagement in the e-society in two
contexts. In Proceedings of the 14th European
Conference on Information Systems (ECIS) 12th-14th
of June. Retrieved September 1, 2012, from
http://csrc.lse.ac.uk/asp/aspecis/20060173.pdf
Nissen, M. E. (2006). Harnessing Knowledge Dynamics:
Principled Organizational Knowing & Learning.
London: IRM Press.
Sulčič, V. and Lesjak, D. (2009). E-learning and study
effectiveness. Journal Of Computer Information
Systems, 49(3), 40-47
Uzunboylu, H. and Tuncay, N. (2010). Divergence of
digital world of teachers. Journal of Educational
Technology & Society, 13(1), 186-194.
Vitolina, I. and Kapenieks, A. (2012). e-Inclusion
measurement by e-learning course delivery. In Annual
Proceedings of Vidzeme of Applied Sciences “ICTE in
Regional Development”, (to be published).
Zhuge, H., Guo, W. and Li, X. (2007). The Potential
Energy of Knowledge Flow. Concurrency &
Computation: Practice & Experience, 19(15), 2067-
2090.
CSEDU2013-5thInternationalConferenceonComputerSupportedEducation
422