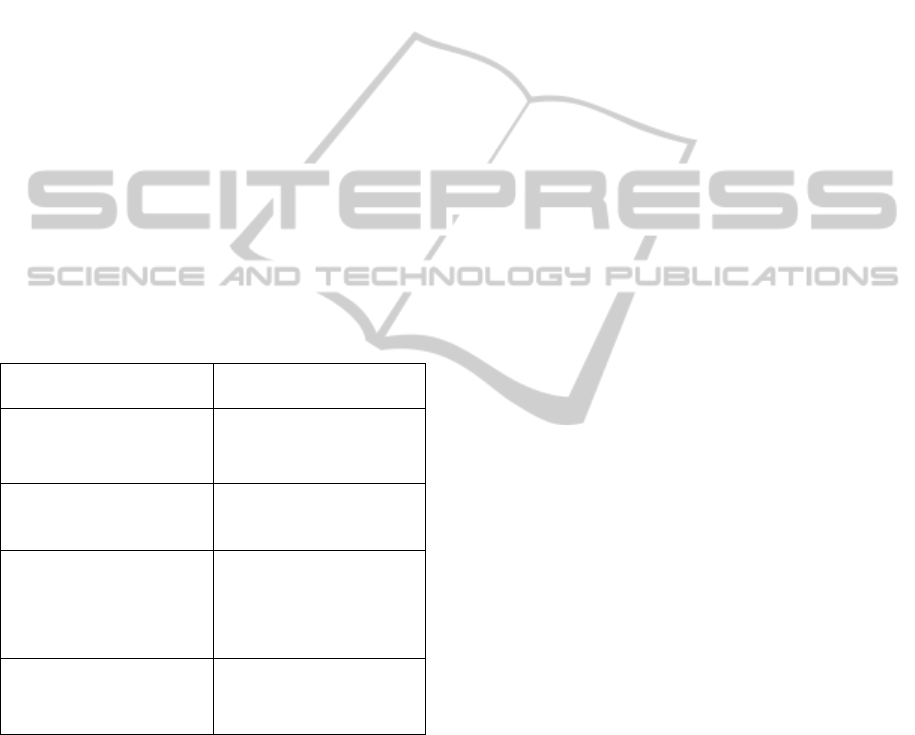
improvement are 0.261 in the intention stage, and
0.235 in the adoption stage. In the multiple-stage
perspective, the perceived behavioral control
2
()D ,
featuring the largest gap value of 0.296 in intention
stage, and the trust-related behaviors
3
()D featuring
the largest gap value of 0.295 in adoption stage,
which should be the first priority for improvement if
decision makers wish to achieve the desired level.
For long-term improvement, the decision makers
should manage internal motivation carefully, as
mentioned above. Given these empirical findings,
our results, as holistically formulated in Table 5,
fulfil the purpose of this research. Evaluating the
mobile learning user behaviour model provided by
this study can extend to most higher education using
mobile learning user behaviour. However, school
administrators should be cautious when applying
this model. The importance of the 9 criteria may
vary according to the situation, and administrators
should compare the mobile learning user behavior
and define the gap in that stage before making
decision on optimal technology use.
Table 5: Sequence of improvement priority for mobile
learning user behaviour.
Formula Sequence of
improvement priority
F1:Influential network of
dimensions
2
()
,
1
()
,
3
()
1
()D
:
1
()C
,
2
()C
,
3
()C
2
()D
:
4
()C
,
5
()C
,
6
()C
F2:Influential network of
criteria within individual
dimensions
3
()
,
2
()
,
1
()
F3:Sequence of
dimension to rise to
aspired/desired level (by
gap value, from high to
low)
1
()D
:
3
()C
,
2
()C
,
1
()C
2
()D
:
5
()C
,
6
()C
,
4
()C
3
()D
:
7
()C
,
9
()C
,
8
()C
F1:Influential network of
dimensions
2
()D
,
1
()D
,
3
()D
1
()D
:
1
()C
,
2
()C
,
3
()C
()D
:
()C
,
()C
,
()C
4 CONCLUSIONS
Mobile learning service has an important role in the
training of higher education. Its decisions are
complicated by the fact that various criteria are
uncertainty and may vary across the different
product categories and use situations. Based on the
export and literature review, we developed the three
dimensions and 9 criteria that align with the mobile
learning service of environment. So we applied the
methodology of hybrid MCDM model combining
DANP with VIKOR in empirical case. The main
reason is among the numerous approaches that are
available for conflict management, hybrid MCDM is
one of the most prevalent. VIKOR is a method
within MCDM; it is based on an aggregating
function representing closeness to the ideal
(aspiration level), which can be viewed as a
derivative of compromise programming for avoiding
“choose the best among inferior alternatives (i.e.,
pick the best apple among a barrel of rotten apples)”.
In a decision-making process, we used the global
and local weights into alternatives performance,
such as that in Table 5, to allow school leader to
select the best mobile learning adoption factor. We
haven't only selected the best factor, but also found
how to improve the gaps to achieve the aspiration
level in mobile learning service performances.
REFERENCES
Liu, J. J. H., Tzeng, G, H., 2012. Comments on “Multiple
criteria decision making (MCDM) methods in
economics: an overview, Technological and Economic
Development of Economy, 18(4), 672-695.
Opricovic, S., Tzeng, G. H., 2004. Compromise solution
by MCDM methods: A comparative analysis of
VIKOR and TOPSIS, European Journal of
Operational Research, 156(2), 445-455.
Opricovic, S., Tzeng, G. H., 2007. Extended VIKOR
method in comparison with outranking methods,
European Journal of Research, 178(2), 514-529.
Saaty, T. L., 1996. Decision Making with Dependence and
Feedback: Analytic Network Process, RWS
Publications, Pittsburgh, New York.
Taylor, S., Todd, P., 1995. Decomposition and crossover
effects in the theory of planned behavior: a study of
consumer adoption intentions, International Journal of
Research in Marketing, 12(2), 137-155.
Tzeng, G. H., Tsaur, S. H., Laiw, Y. D., Opricovic, S.,
2002a. Multicriteria analysis of environmental quality
in Taipei: Public preferences and improvement
strategies. Journal of Environmental Management,
65(2), 109-120.
Tzeng, G. H., Teng, M. H., Chen, J. J., Opricovic, S.,
2002b. Multicriteria selection for a restaurant location
in Taipei. International Journal of Hospitality
Management, 21(2), 171-187.
Tzeng, G. H., Lin, C. W., Opricovic S., 2005. Multi-
criteria analysis of alternative-fuel buses for public
transportation. Energy Policy, 33(11), 1373-1383.
Yu, P. L., 1973. A class of solutions for group decision
problems. Management Science, 19(8), 936-946.
EvaluatingMobileLearningAdoptioninHigherEducationbasedonNewHybridMCDMModels
271