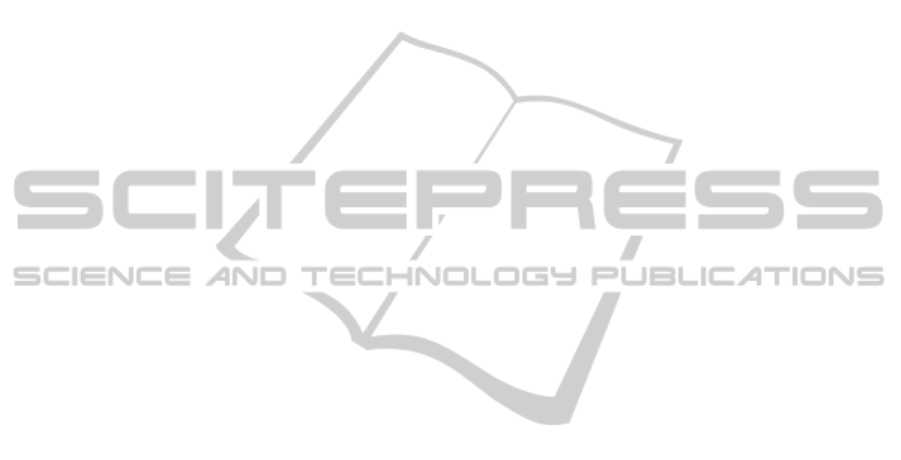
6 CONCLUSIONS
In this paper, we have provided an overview of the
core design principles and architecture of CTSiM – a
learning environment which seamlessly integrates
domain-general CT concepts with domain-specific
representational practices of a variety of STEM dis-
ciplines. Using a kinematics and and an ecology
unit, we show how CTSiM is effective in producing
learning gains for both science topics. We also ex-
plained and classified a variety of challenges (and
corresponding scaffolds) faced by a high- and a low-
performing student while they worked with CTSiM.
Our results indicate that the challenges faced by
these students generally decreased with time for se-
quences of related units, but, as expected, again in-
creased when new computational constructs or mod-
eling complexities were introduced. The decrease in
the number of challenges illustrates the combined ef-
fectiveness of our architecture, curricular unit de-
sign, and scaffolds. Further, the specific challenges
and scaffolds identified lay the groundwork for inte-
grating adaptive scaffolding in CTSiM to help stu-
dents develop a synergistic understanding of CT and
science concepts.
ACKNOWLEDGEMENTS
This work was supported by the NSF (NSF Cyber-
learning grant #1237350).
REFERENCES
Basu, S., Kinnebrew, J., Dickes, A., Farris, A.V.,
Sengupta, P., Winger, J., & Biswas, G. (2012). A Sci-
ence Learning Environment using a Computational
Thinking Approach. In Proceedings of the 20th Inter-
national Conference on Computers in Education (pp.
722-729). Singapore.
Conway, M. (1997). Alice: Easy to Learn 3D Scripting for
Novices, Technical Report, School of Engineering and
Applied Sciences, University of Virginia, Char-
lottesville, VA.
Gomm, R., Hammersley, M. and Foster, P. (2000). Case
Study Method: Key Issues, Key Texts. Sage, Thou-
sand Oaks, CA.
Guzdial M. (1995) Software-realized scaffolding to facili-
tate programming for science learning. Interactive
Learning Environments, 4(1). 1-44.
Hambrusch, S., Hoffmann, C., Korb, J.T., Haugan, M.,
and Hosking, A.L. (2009). A multidisciplinary ap-
proach towards computational thinking for science
majors. In Proceedings of the 40th ACM technical
symposium on Computer science education (SIGCSE
'09). ACM, New York, NY, USA, 183-187.
Kelleher, C. & Pausch, R. (2005) Lowering the barriers
to programming: a taxonomy of programming envi-
ronments and languages for novice programmers,
ACM Computing Surveys, Vol. (37) 83–137.
Klopfer, E., Yoon, S. and Um, T. (2005). Teaching Com-
plex Dynamic Systems to Young Students with Star-
Logo. The Journal of Computers in Mathematics and
Science Teaching; 24(2): 157-178.
Lehrer, R., & Schauble, L. (2006). Cultivating model-
based reasoning in science education. In R. K. Sawyer
(Ed.), The Cambridge handbook of the learning sci-
ences (pp. 371–388). New York: Cambridge Universi-
ty Press.
Maloney, J., Burd, L., Kafai, Y., Rusk, N., Silverman, B.,
and Resnick, M. (2004) Scratch: A Sneak Preview. In
Proc. of Creating, Connecting, and Collaborating
through Computing, 104-109.
National Research Council. (2011). A framework for K-12
Science Education: Practices, Crosscutting Concepts,
and Core Ideas. Washington, DC: The National Acad-
emies Press.
Papert, S. (1980). Mindstorms: children, computers, and
powerful ideas. Basic Books, Inc. New York, NY.
Redish, E. F. and Wilson, J. M. (1993). Student program-
ming in the introductory physics course: M.U.P.P.E.T.
Am. J. Phys. 61: 222–232.
Repenning, A. (1993). Agentsheets: A tool for building
domain-oriented visual programming, Conference on
Human Factors in Computing Systems, 142-143.
Sengupta, P., Farris, A.V, & Wright, M. (2012a). From
Agents to Aggregation via Aesthetics: Learning Me-
chanics with Visual Agent-based Computational Mod-
elling. Technology, Knowledge & Learning. 17 (1-2),
pp 23 - 42.
Sengupta, P., Kinnebrew, J.S., Biswas, G., & Clark, D.
(2012b). Integrating computational thinking with K-12
science education: A theoretical framework. In 4th In-
ternational Conference on Computer Supported Edu-
cation (pp. 40-49). Porto, Portugal.
Sengupta, P., Kinnebrew, J.S., Basu, S., Biswas, G., &
Clark, D. (2013). Integrating Computational Thinking
with K-12 Science Education Using Agent-based
Computation: A Theoretical Framework. Education
and Information Technologies.
Sherin, B. (2001). A comparison of programming lan-
guages and algebraic notation as expressive languages
for physics. International Journal of Computers for
Mathematics Learning: 6, 1-61.
Wilensky, U. (1999). NetLogo. Center for Connected
Learning and Computer-based Modelling (http://
ccl.northwestern.edu/netlogo). Northwestern Universi-
ty, Evanston, IL.
Wilensky, U., & Reisman, K. (2006). Thinking like a
wolf, a sheep or a firefly: Learning biology through
constructing and testing computational theories - An
embodied modelling approach. Cognition & Instruc-
tion, 24(2), 171-209.
Wing, J. M. (2010). Computational Thinking: What and
Why? Link Magazine.
CSEDU2013-5thInternationalConferenceonComputerSupportedEducation
378