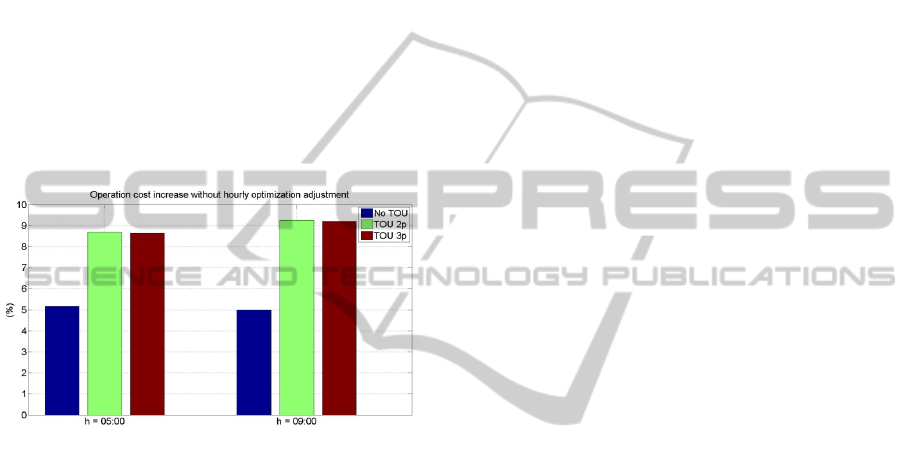
5 BENEFITS ANALYSIS
To demonstrate the advantages of proactive control
in terms of minimising the running costs, the savings
have been calculated and compared for the three
different tariffs applied. As the base case for this
comparison it is assumed the fixed daily schedule
without any changes applied by the proactive
management. In this base case the battery charge and
discharge cycles are fixed and any demand variation
is covered by importing/exporting the power from/to
the grid. In Figure 10 the increase in running costs as
a result of not applying the hourly adjustment is
shown. For the single tariff case the cost saving is
around 5% while for the two-tariff case and the
“super off-peak” tariff the potential for the savings
increase to about 9%.
Figure 10: Increase in operational costs when proactive
control updates are not applied.
The proactive management clearly demonstrates its
capacity to achieve cost savings with or without the
tariff discrimination system by matching the demand
peaks with the available local generation. In case of
the differential pricing system, the additional saving
benefits are gained by the demand shifting
techniques and the battery management.
6 CONCLUSIONS
This paper has discussed the operational and cost
related aspects of the proactive resource
management system for Smart buildings. It has been
demonstrated that the proposed solution is sensitive
to any unforeseen disturbance in demand,
generation, access tariffs, ambient conditions,
building occupancy, building services etc. and by
using optimised dispatch significantly reduces
operational costs.
The proposed methodology introduces a
hierarchical approach to the multi-energy system
design, long term resource scheduling and proactive
RT response. Energy surplus and energy shortage
scenarios have been both considered and with
optional capacity restrictions in the system grid
connection.
Simulation results and cost/benefit analysis have
been used to demonstrate some of the features of the
proposed proactive control.
ACKNOWLEDGEMENTS
The authors would like to thank Sacyr Vallehermoso
and Ministry of Science and Innovation,
Government of Spain, for their help and funding
research project THOFU (Cenit).
REFERENCES
Téllez M. B; Gafurov, T.; Prodanović M., 2011: Proactive
control for energy systems in smart buildings, IEEE
PES Innovative Smart Grid Technologies 2011 Europe
Prodanović, M., Gafurov, T., Tellez, M. B., 2012. A
demand based approach to optimisation of energy
supply mix for smart buildings, IEEE PES Innovative
Smart Grid Technologies, 2012
ABB, 2010., Technology of choice smart home and
intelligent building control ABB i-bus® KNX.
[Online]. Available: http://knx-gebaeudesysteme.de/
sto_g/English/GENERAL_DOCUMENTATION/Tech
nology_of_choice_2CDC500064M0201.pdf
Vikon by Tridium 2009. Creating better buildings.
[Online]. Available: http://www.vykon.com/galleries/
brochures/VYKONOverviewBroch.pdf
Doukas, H., Patlitzianas, K. D., Iatropoulos, K., and
Psarras, J., 2007, Intelligent building energy
management system using rule sets, Building and
Environment
Gupta, A., Saini R. P.,and Sharma, M. P., 2010, Modelling
of hybrid energy system -Part I: Problem formulation
and model development, Renewable Energy, 36: 459-
465, June 2010.
Brooks, A., Lu, E., Reicher, D., Spirakis, C., and Weihl.
B., 2010, Demand dispatch: Using real-time control of
demand to help balance generation and load, Power
and Energy Magazine, IEEE, 8(3):20–29, June 2010.
Todorović, M. S., Djurić, O. E., Matinović, I., Ličina, D.,
2011, Renewable Energy Sources and Energy
Efficiency for Building's Greening: From traditional
village houses via high-rise residential building's BPS
and RES powered co- and tri-generation towards net
ZEBuildings and Cities, in Proc. 2011 IEEE
International Symposium on Exploitation of
Renewable Energy Sources, pp. 29-37.
SMARTGREENS2013-2ndInternationalConferenceonSmartGridsandGreenITSystems
170