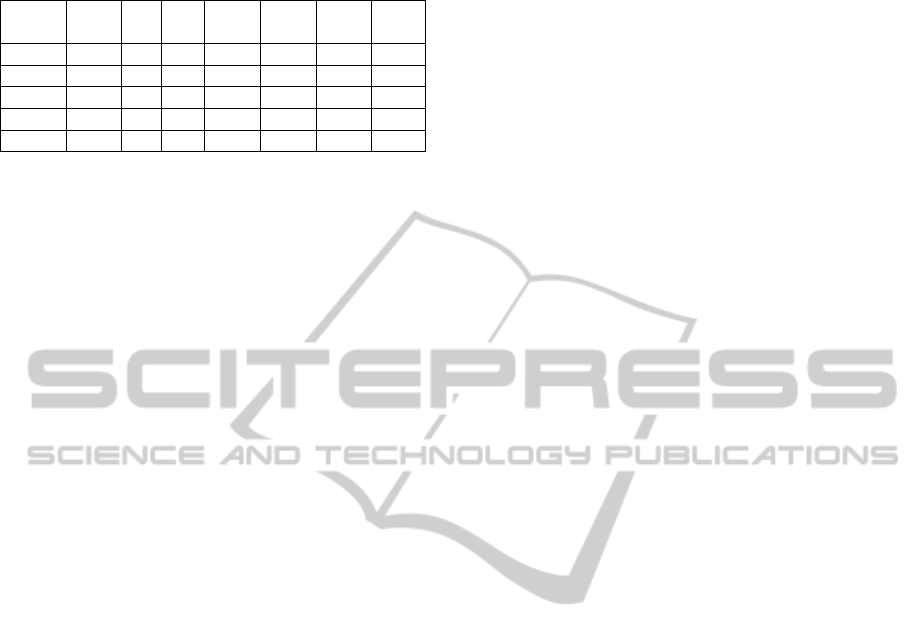
Table 4: Analysis of students satisfaction estimates
according to PCM.
Quality
criterion
Mean Min Max Mode Media
MSE SD
1 0,16 -2,35 4,26 0,54 -0,04 0,52 1,18
-0,04 -5,57 3,5 -0,23 -0,23 0,68 0,68
3 0,28 -2,54 5,39 0,42 0,21 0,53 1,17
0,32 -1,48 2,74 0,09 0,09 0,52 0,99
5 0,03 -3,6 2,84 -0,26 -0,05 0,53 1,23
errors for RM are greater than for PCM which
indicates a smaller dispersion of estimates for PCM.
So estimates obtained with the help of PCM are
more adequate and preferable.
Since PCM is more adequate we calculate
weighted and unweighted arithmetical means (WAM
and UWAM) and geometrical means (WGM and
UWGM) only for this model. To find WAM and
WGM the following weight coefficients were used:
α1 = 0,27, α2 = 0,2, α3 = 0,15, α4 = 0,25, α5 = 0,13.
The obtained results are the following: UWAM =
0,15, WAM = 0,16, UWGM = -0,11, WGM = -0,13.
So these results are close to overall satisfaction
level equal to 0,1 which was found as the mean of
i
θ
(Table 2). Using such decomposition except
aggregated estimate we can find intermediate
estimates. Furthermore we can assign different
weight coefficient to make the comprehensive result
more suitable for the purposes of decision-making.
In the case when we evaluate whether the quality
perceived by students corresponds to the given level,
we deal with the CA problem of the first class. Such
estimate can be found based on input data which are
the estimates of students’ satisfaction by five quality
criteria. The switch chain consists of three layers of
Boolean functions. The first layer is represented by
the function that defines whether each student’s
satisfaction value is greater than defined level (it
returns 1, if this requirement is fulfilled, 0 –
otherwise). We take the median
06,0
. The
function of the second layer checks whether a single
criterion is assessed positively, i.e. the most of
satisfaction values of single criterion are greater than
defined level. In our case the estimates given by
more than 37 students have to be bigger than the
defined level. The third layer function defines
whether the requirement to satisfaction level over all
criteria is fulfilled. In this case study at least 3
criteria must meet the requirement. Under the value
6,0
we found out that the perceived quality is
satisfactory.
To accomplish our case study we made the CA
of the third class. As CA we take the estimate of
proposed quality. The main hypothesis is that
proposed quality defines the estimates of perceived
quality. We suppose that these estimates correspond
to calculated
i
θ . To find CA value we suggest to use
Spearman Single Factor Model. We obtained value
of proposed quality equal to 1,97 logits. This
corresponds to enough level of educational services.
The obtained results showed the satisfactory
education quality from three different points of
view. Therefore we suggest to use described
approach for implementation of M&E IS.
5 CONCLUSIONS AND FUTURE
WORK
According to the functionality classification the
following IS can be distinguished: systems of
administrative, financial and economic management;
systems of educational process management and
support; systems of scientific and research work
management; systems of information resources
management.
All of them should contain the CA unit. Due to
goals and management tasks the different models
can be used. We have realized three main classes of
CA problems. The certain framework is associated
with every problem’s class.
We have discovered the most advanced
procedures of CA. They are expert judgments,
qualimetric practices or statistical analysis for initial
estimates (inputs of CA). We suggest Switch Chains,
Network Assessment and Probability-based
Reasoning in order to construct comprehensive
assessment model. Our researches are strictly
devoted to implementation of CA procedures. On
the other hand, we have tried to generalize our
experience to provide some formal approach.
The investigation of the case-study shows
potential possibilities of suggested frameworks
usage. We should note that the estimation of
students’ satisfaction is not the clearest way to
demonstrate the advantages of our approach. But we
hope that the aim of illustration how different tasks
influence comprehensive assessment is reached.
As a result of this work we can underline the
following: 1) the process of CA is represented in two
stages: estimation of separate elements and their
aggregation into CA value; 2) three classes of
problems and CA frameworks related to those
classes are defined; 3) the set of experiments based
on evaluation of students’ satisfaction were done; 4)
the principle role of probability-based reasoning
methods for performance evaluation is proved.
MonitoringandEvaluationProblemsinHigerEducation-ComprehensiveAssessmentFrameworkDevelopment
459