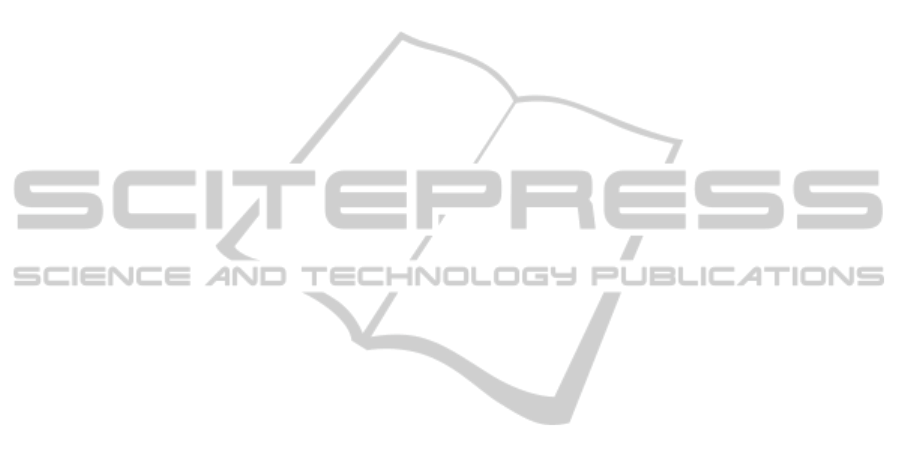
The proposed framework is based on recursive sub-
space system identification techniques to generate
fault dependent symptoms from eigenvalues residu-
als. The parametric fault detection architecture was
tested on a nonlinear plant whose results demonstrate
the feasibility of the proposed approach in detecting
parametric faults.
ACKNOWLEDGEMENTS
This work has been supported by iCIS-Intelligent
Computing in the Internet of Services, Project
CENTRO-07-ST24-FEDER-002003.
REFERENCES
Bart De Moor, Peter Van Overschee, W. F. (1999). Algo-
rithms for subspace state space system identification
- an overview. In Datta, B. N., editor, Applied and
Computational Control, Signals and Circuits: Volume
1: Vol 1, volume Vol. 1. Birkhauser.
Basseville, M., Abdelghani, M., and Benveniste, A. (2000).
Subspace-based fault detection algorithms for vibra-
tion monitoring. Automatica, 36(1):101 – 109.
Basseville, M., Benveniste, A., Goursat, M., and Mevel,
L. (2007). Subspace-based algorithms for struc-
tural identification, damage detection, and sensor
data fusion. EURASIP J. Appl. Signal Process.,
2007(1):200–200.
Brito Palma, L., Coito, F., and Neves da Silva, R. (2005).
Diagnosis of parametric faults based on identification
and statistical methods. In Decision and Control, 2005
and 2005 European Control Conference. CDC-ECC
’05. 44th IEEE Conference on, pages 3838 – 3843.
Chen, J. and Patton, R. (1999). Robust Model-Based Fault
Diagnosis for Dynamic Systems. Kluwer Academic
Publishers.
Chen, T., Chen, H., and wen Liu, R. (1995). Approxi-
mation capability in c(r macr;n) by multilayer feed-
forward networks and related problems. Neural Net-
works, IEEE Transactions on, 6(1):25 –30.
Dong, Q., Matsui, K., and Huang, X. (2002). Existence and
stability of periodic solutions for hopfield neural net-
work equations with periodic input. Nonlinear Analy-
sis: Theory, Methods & Applications, 49(4):471 479.
dos Santos, P. and de Carvalho, J. (2003). Improving the
numerical efficiency of the b and d estimates pro-
duced by the combined deterministic-stochastic sub-
space identification algorithms. In Decision and Con-
trol, 2003. Proceedings. 42nd IEEE Conference on,
volume 4, pages 3473 – 3478 vol.4.
Gertler, J. (1998). Fault Detection and Diagnosis in Engi-
neering Systems. Marcel Dekker.
Hwang, I., Kim, S., Kim, Y., and Seah, C. (2010). A survey
of fault detection, isolation, and reconfiguration meth-
ods. Control Systems Technology, IEEE Transactions
on, 18(3):636 –653.
Isermann, R. (1997). Supervision, fault-detection and fault-
diagnosis methods: An introduction. Control Engi-
neering Practice, 5(5):639 – 652.
Isermann, R. (2011). Fault-Diagnosis Applications.
Springer-Verlag.
Isermann, R. and Ball
´
e, P. (1997). Trends in the applica-
tion of model-based fault detection and diagnosis of
technical processes. Control Engineering Practice,
5(5):709 – 719.
Leshno, M., Lin, V. Y., Pinkus, A., and Schocken, S. (1993).
Multilayer feedforward networks with a nonpolyno-
mial activation function can approximate any func-
tion. Neural Networks, 6(6):861 – 867.
Merc
`
ere, G. (2005). Contribution
`
a l’identification
r
´
ecursive des syst
`
emes par l’approche des sous-
espaces (in French). PhD thesis, Universit
´
e des Sci-
ences et Technologie de Lille, France.
Merc
`
ere, G., Bako, L., and Lecœuche, S. (2008).
Propagator-based methods for recursive subspace
model identification. Signal Processing, 88(3):468–
491.
Merc
`
ere, G., Lecœuche, S., and Lovera, M. (2004). Re-
cursive subspace identification based on instrumental
variable unconstrained quadratic optimization. Inter-
national Journal of Adaptive Control and Signal Pro-
cessing, 18(9-10):771–797.
Merc
`
ere, G. and Lovera, M. (2007). Convergence analysis
of instrumental variable recursive subspace identifica-
tion algorithms. Automatica, 43(8):1377 – 1386.
Montgomery, D. C. (2001). Introduction to Statistical Qual-
ity. John Wiley & Sons.
Moor, B. D., Overschee, P., and Favoreel, W. (1999). Al-
gorithms for subspace state-space identification: An
overview. In Datta, B. N., editor, Applied and compu-
tational control, signals, and circuits, volume 1, chap-
ter 6, pages 247 – 311. Birkhuser.
Munier, J. and Delisle, G. (1991). Spatial analysis using
new properties of the cross-spectral matrix. Signal
Processing, IEEE Transactions on, 39(3):746 –749.
Oku, H. and Kimura, H. (2002). Recursive 4sid algorithms
using gradient type subspace tracking. Automatica,
38(6):1035 – 1043.
ICINCO2013-10thInternationalConferenceonInformaticsinControl,AutomationandRobotics
88