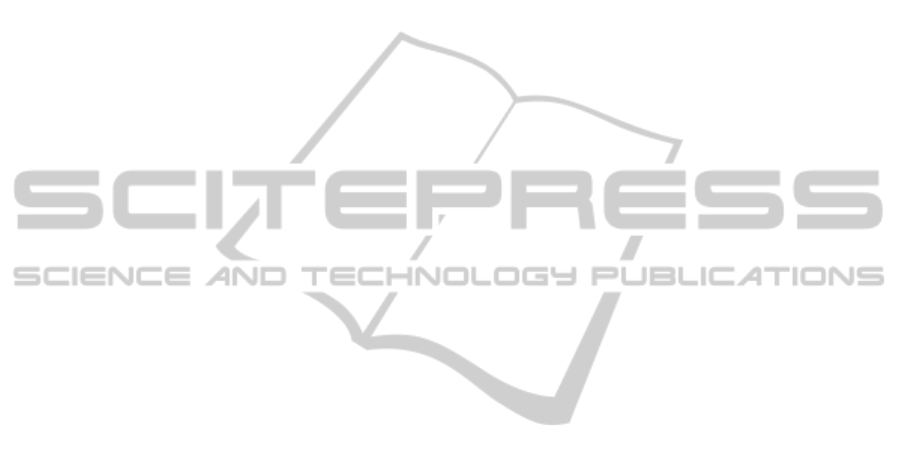
analytic controllers with an advanced self-learning
system was developed for a printing machine.
For this machine a transfer function model was
designed which describes the principal behaviour.
To identify the model parameter before the
production start, diverse devices and information
about the consumables and the environmental
conditions are used. All influences, whose
correlation can be described analytically, are also
calculated in that way. Influences with unknown
mode of action are regarded with a Neural Network.
Therefore the most important impacts are measured,
conditioned and fed to the network. So it is possible
to predict the machine’s behaviour under varying
operation conditions with unknown effects. The
simulation model and the controller are turned
according the adapted parameter to guarantee a
stable and dynamic production. This enables higher
product quality and efficiency.
ACKNOWLEDGEMENTS
The authors want to express their gratitude to the
state government of Bavaria for its financial support
of the project “CogSYS – Resource Efficiency by
Cognitive Control Systems”.
REFERENCES
Aström, K. J., Hägglund, T., 2004. Revisiting the Ziegler–
Nichols step response method for PID control.
Bayer, J., Osendorfer, C., Smagt, P., 2011: Learning
Sequence Neighbourhood Metrics. Technical
University Munich.
Beuschel, M., 2000. Neuronale Netze zur Diagnose und
Tilgung von Drehmomentschwingungen am
Verbrennungsmotor, Technical University Munich.
Eberhard, M., 2006. Optimisation of Filtration by
Application of Data Mining Methods, Dissertation,
Technical University Munich.
Faridi, A.; Golian, A., 2011: Use of neural network
models to estimate early egg production in broiler
breeder hens through dietary nutrient intake. In:
Poultry Science.
Govindhasamy, J. J.; McLoone, S. F.; Irwin, George W.;
French, J.; Doyle, R. P., 2005: Neural modelling,
control and optimisation of an industrial grinding
process. In: Control Engineering Practice 13.
Guanyuz, Zhou (2012): Online incremental feature
Learning with denoising autoencoders. International
Conference on Artificial Intelligence and Statistic,
2012.
Hafner, R. 2009: Dateneffiziente selbstlernende neuronale
Regler. University Osnabrück.
Hintz, Christian, 2003: Identifikation nichtlinearer
mechatronischer Systeme mit strukturierten
rekurrenten Netzen. Dissertation. Technical University
München.
Huang, S.H; Hong-Chao Z., 1994: Artificial neural
networks in manufacturing: concepts, applications,
and perspectives. IEEE Trans. Comp., Packag.,
Manufact. Technologies.
Kipphan, H., 2002. Handbuch der Printmedien, Springer
Verlag, Berlin.
Moon, P.; et al. , 2008. Ink-jet printing process modeling
using neural networks. International Electronics
Manufacturing Technology Conference.
Rangwala, S. Dornfeld, D. A., 1989. Learning and
optimization of machining operations using computing
abilities of neural networks, IEEE Transactions on
Systems, Man, and Cybernetics.
Ramesh. R., Mannan M.A., Poo A.N., 2002. Support
vector machines model for Classification of Thermal
Error in Machine Tools, in: The International Journal
of Advanced Manufacturing Technology.
Rajagopalan, Ramesh; Rajagopalan, Purnima, 1996.
Applications of Neural Network in Manufacturing.
International Conference on System Sciences.
Suzuki, K, 2011. Artificial Neural Networks - Industrial
and Control Engineering Applications.
Wang, D., 1984. An investigation of the applicacability of
Walker and Fetsko ink transfer equation, Rochester,
New York.
Rae, T.A; et al, 1996. The application of neural Networks
to induction machine control. Proceedings of IEEE.
Zell, A., 1994. Simulation neuronaler Netze, Oldenburg
Verlag, München.
ICINCO2013-10thInternationalConferenceonInformaticsinControl,AutomationandRobotics
138