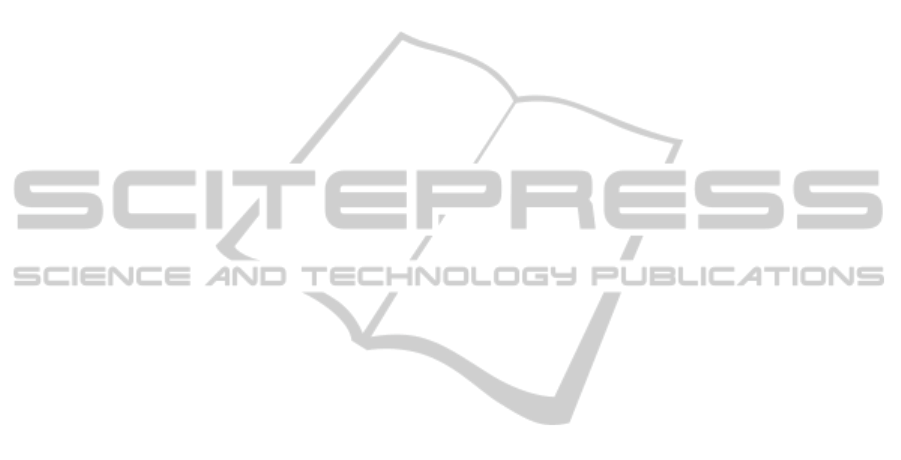
proper number of agents, it is possible to approximate
the course of a disease as observed in the real world.
Furthermore, our experiments indicate that the algo-
rithmic framework presented in this paper is able to
describe, to some extent, the impact of certain non-
pharmaceutical countermeasures on the behavior of
an epidemic.
REFERENCES
Adamic, L. A. and Huberman, B. A. (2000). Power-
law distribution of the world wide web. Science,
287(5461):2115.
Ajelli, M., Goncalves, B., Balcan, D., Colizza, V., Hu, H.,
Ramasco, J., Merler, S., and Vespignani, A. (2010).
Comparing large-scale computational approaches to
epidemic modeling: Agent-based versus structured
metapopulation models. BMC Infectious Diseases,
10(190).
Amaral, L. A., Scala, A., Barthelemy, M., and Stanley,
H. E. (2000). Classes of small-world networks. PNAS,
97(21):11149–11152.
Arbeitsgemeinschaft Influenza (2011). Bericht zur Epi-
demiologie der Influenza in Deutschland Saison
2010/11.
Balcan, D., Hu, H., Goncalves, B., Bajardi, P., Poletto, C.,
Ramasco, J. J., Paolotti, D., Perra, N., Tizzoni, M.,
den Broeck, W. V., Colizza, V., and Vespignani, A.
(2009). Seasonal transmission potential and activity
peaks of the new influenza A(H1N1): a Monte Carlo
likelihood analysis based on human mobility. BMC
Medicine, 7:45.
Bobashev, G. V., Goedecke, D. M., Yu, F., and Epstein,
J. M. (2007). A hybrid epidemic model: combining
the advantages of agent-based and equation-based ap-
proaches. In Proc. WSC ’07, pages 1532–1537.
Borgs, C., Chayes, J., Ganesh, A., and Saberi, A. (2010).
How to distribute antidote to control epidemics. Ran-
dom Struct. Algorithms, 37:204–222.
Chowell, G., Hyman, J. M., Eubank, S., and Castillo-
Chavez, C. (2003). Scaling laws for the movement
of people between locations in a large city. Physical
Review E, 68(6):661021–661027.
Els
¨
asser, R. and Ogierman, A. (2012). The impact of the
power law exponent on the behavior of a dynamic epi-
demic type process. In Proc. SPAA’12.
Eubank, S., Guclu, H., Kumar, V., Marathe, M., Srinivasan,
A., Toroczkai, Z., and Wang, N. (2004). Modelling
disease outbreaks in realistic urban social networks.
Nature, 429(6988):180–184.
Faloutsos, M., Faloutsos, P., and Faloutsos, C. (1999). On
power-law relationships of the internet topology. In
SIGCOMM ’99, pages 251–262.
Germann, T. C., Kadau, K., Longini, I. M., and Macken,
C. A. (2006). Mitigation strategies for pandemic in-
fluenza in the United States. PNAS, 103(15).
Grassberger, P. (1983). On the critical behavior of the
general epidemic process and dynamical percolation.
Mathematical Biosciences, 63(2):157 – 172.
Hethcote, H. W. (2000). The mathematics of infectious dis-
eases. SIAM Review, 42(4):599–653.
Jaffry, S. W. and Treur, J. (2008). Agent-Based and
Population-Based Simulation: A Comparative Case
Study for Epidemics. In Louca, L. S., Chrysan-
thou, Y., Oplatkova, Z., and Al-Begain, K., editors,
ECMS’08, pages 123–130.
Lee, B. Y., Bedford, V. L., Roberts, M. S., and Carley, K. M.
(2008). Virtual epidemic in a virtual city: simulat-
ing the spread of influenza in a us metropolitan area.
Translational Research, 151(6):275 – 287.
Lee, B. Y., Brown, S. T., Cooley, P. C., Zimmerman, R. K.,
Wheaton, W. D., Zimmer, S. M., Grefenstette, J. J.,
Assi, T.-M., Furphy, T. J., Wagener, D. K., and Burke,
D. S. (2010). A computer simulation of employee vac-
cination to mitigate an influenza epidemic. American
Journal of Preventive Medicine, 38(3):247 – 257.
Markel, H., Lipman, H. B., Navarro, J. A., Sloan, A.,
Michalsen, J. R., Stern, A. M., and Cetron, M. S.
(2007). Nonpharmaceutical Interventions Imple-
mented by US Cities During the 1918-1919 Influenza
Pandemic. JAMA, 298(6):644–654.
Newman, M. E. J. (2002). Spread of epidemic disease on
networks. Phys. Rev. E, 66(1):016128.
Newman, M. E. J. (2003). The structure and function of
complex networks. SIAM Review, 45(2):167–256.
Ripeanu, M., Foster, I., and Iamnitchi, A. (2002). Mapping
the gnutella network: Properties of large-scale peer-
to-peer systems and implications for system. IEEE
Internet Computing Journal, 6(1):50–57.
Robert Koch Institute (2012). SurvStat@RKI. A web-based
solution to query surveillance data in Germany.
SIMULTECH2013-3rdInternationalConferenceonSimulationandModelingMethodologies,Technologiesand
Applications
274