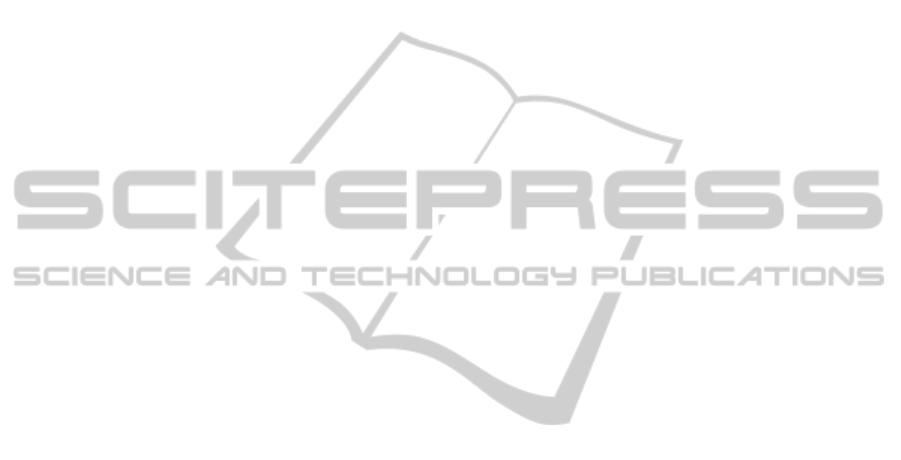
learning is just an instructional tool. We conclude
that instructor’s facilitating roles and feedback is the
most critical factor in e-learning that changes the e-
learning process positively and that changes learner-
instructor relationship positively to make e-learning
a superior mode of instruction.
REFERENCES
Akyol, Z. and Garrison, D. R. (2011) In Student
Satisfaction and Learning Outcomes in e-Learning:
An introduction to empirical research (Eds, Eom, S.
B. and Arbaugh, J. B.) Information Science Reference
(an imprint of IGI Global), Hersey, PA, pp. 23-35.
Alexander, M. W., Perrault, H., Zhao, J. J. and Waldman,
L. (2009) In Journal of Educators Online, Vol. 6.
Alfonseca, E., Carro, R. M., Martín, E., Ortigosa, A. and
Paredes, P. (2006) User Modeling and User - Adapted
Interaction, 16, 377-401.
Arbaugh, J. B. (2000) Business Communication Quarterly,
63, 9-18.
Arbaugh, J. B. (2001) Business Communication Quarterly,
64, 42-54.
Arbaugh, J. B., Godfrey, M. R., Johnson, M., Pollack, B.
L., Niendorf, B. and Wresch, W. (2009) Internet and
Higher Education, 12, 71-87.
Bonk, C. J. (2009) The World is Open: How Web
Technology is Revolutionizing Education Jossey-Bass,
San Francisco, CA
Butler, D. L. and Winne, P. H. (1995) Review of
Educational Research, 65, 245-281.
Chen, C. S. (2002) Information Technology, Learning, and
Performance Journal, 20, 11-25.
Chi, M. T. and VanLehn, K. A. (1991) The Journal of
Learning Sciences, 1, 69-105.
Chin, W. W. (1998a) MIS Quarterly, 22, VII-XVI.
Chin, W. W. (1998b) In Modern Methods for Business
Research(Ed, Marcoulides, G. A.) Lawrence Erlbaum
Associates, Mahwah, NJ, pp. 295-336.
Cohen, J. (1988) Statistical Power Analysis for the
Behavioral Sciences Lawrence Erlbaum Associates,
publishers, Hillsdale, NJ.
Dembo, M. and Eaton, M. (2000) The Elementary School
Journal, 100, 473-490.
Dick, W. and Carey, L. (1990) The Systematic Design of
Instruction, Harper Collins Publishers, New York,
NY.
Dunn, R., Beaudry, J. and Klavas, A. (1989) Education
Leadership, 46, 50-58.
Eom, S. B., Ashill, N. and Wen, H. J. (2006) Decision
Sciences Journal of Innovative Education, 4, 215-236.
Falk, R. F. and Miller, N. B. (1992) A primer for soft
modeling, The University of Akron Press, Akron, OH.
Fornell, C. R. and Bookstein, F. L. (1982) In A second
Generation of multivariate analysis(Ed, Fornell, C.)
Praeger, New York, pp. 289-394
Fornell, C. R. and Larcker, D. (1981) Journal of
Marketing Research, 18, 39-50.
Frankola, K. (2001) Workforce, 80, 53-60.
Galusha, J. M. (1997) Interpersonal Computing and
Technology: An Electronic Journal for the 21st
Century, 5, 6-14.
Gardner, H. (1983) Frames of Mind: The Theory of
Multiple Intelligences, Basic Books, New York.
Garrison, D. R. (2009) In Encyclopedia of distance and
online learning learning(Ed, Howard, C.) IGI Global,
Hersey, PA, pp. 352-355.
Garrison, D. R. and Anderson, T. (2003) E-learning in the
21st century: A framework for research and practice,
Routledge/Falmer, London, UK.
Geisser, S. (1975) Journal of the American Statistical
Association, 70, 320-328.
Hayes, S. K. (2007) MERLOT Journal of Online Teaching
and Learning, 3, 460-465.
Haytko, D. L. (2001) Marketing Education Review, 11,
27-39.
Huynh, M. Q. (2005) Journal of Electronic Commerce in
Organizations, 3, 33-45.
Kellogg, D. L. and Smith, M. A. (2009) Decision Sciences
Journal of Innovative Education, 7, 433-454.
Lam, W. (2005) Journal of Electronic Commerce in
Organizations, 3, 18-41.
LaPointe, D. K. and Gunawardena, C. N. (2004) Distance
Education, 25, 83-106.
LaRose, R. and Whitten, P. (2000) Communication
Education, 49, 320-338.
Leidner, D. E. and Jarvenpaa, S. L. (1995) MIS Quarterly,
19, 265-291.
Ley, K. (1999) Campus-Wide Information Systems, 16,
63-70.
Liu, X., Magjuka, R. J., Bonk, C. J. and Lee, S. (2007)
Quarterly Review of Distance Education, 8, 9-24.
Marriott, N., Marriott, P. and Selwyn, N. (2004)
Accounting Education, 13, 117-130.
Means, B., Toyama, Y., Murphy, R., Bakia, M. and Jones,
K. (2009) U.S. Department of Education, Washington,
DC.
Moore, M. G. (1989) The American Journal of Distance
Education, 3, 1-6.
Moore, M. G. (1991) The American Journal of Distance
Education, 5, 1-6.
Morgan, G. and Adams, J. (2009) Journal of Interactive
Learning Research, 20, 129-155.
Northrup, P. T. (2002) The Quarterly Review of Distance
Education, 3, 219-226.
Nunnally, J. C. (1978) Psychometric Theory, McGraw-
Hill, New York.
Peltier, J. W., Drago, W. and Schibrowsky, J. A. (2003)
Journal of Marketing Education, 25, 260-276.
Picciano, A. (1998) Journal of Asynchronous Learning
Networks, 12, 1-14.
Piccoli, G., Ahmad, R. and Ives, B. (2001) MIS Quarterly,
25, 401-426.
Pintrich, P. R., Smith, D. A., Garcia, T. and McKeachie,
W. J. (1991) A manual for the use of the Motivated
Strategies for Learning Questionnaire (MSLQ),
National Center for Research to Improve
Postsecondary Teaching and Learning, Ann Arbor:
DevelopingandTestingaModeltoUnderstandRelationshipsbetweene-LearningOutcomesandHumanFactors
369