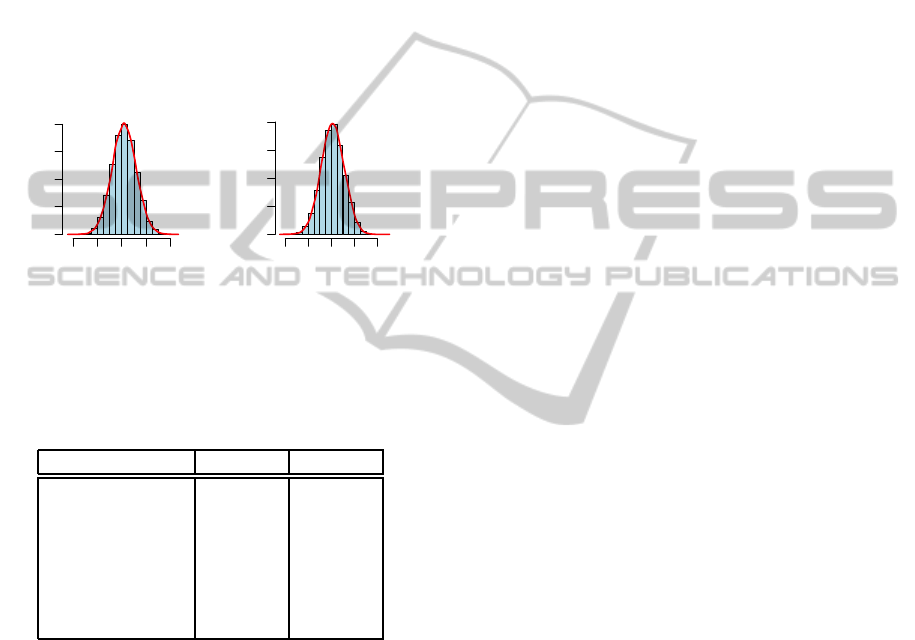
Results of estimation of regression coefficients are
given in Table 1. The mean values of posterior dis-
tributions are
ˆ
β
0
= −5.799 and
ˆ
β
1
= 0.11, with the
corresponding 95% credibility intervals (defined as
highest density intervals) being [−7.762,−3.827] and
[−1.853,2.075] for β
0
and β
1
, respectively. One of
the rules of thumb recommends that the simulation
should be run until the Monte Carlo error for each
parameter of interest falls below 5% of the sample
standard deviation. Table 1 shows that the simulation
reached less than 0.6% for both coefficients.
The posterior distributions of β
0
and β
1
are de-
picted in Fig. 8 as histograms of Monte Carlo samples
together with kernel density estimates (in red).
−10 −6 −2
0.0 0.2 0.4
−4 0 4
0.0 0.2 0.4
Figure 8: Bayesian beta regression – posterior distributions
of β
0
(left) and β
1
(right). Histograms depict relative fre-
quency of MCMC samples, red lines are respective kernel
density estimates.
Table 1: Results of MCMC estimation of beta regression
model.
˜
x
x
x
2.5
,
˜
x
x
x
50
and
˜
x
x
x
97.5
denote 2.5%, 50% and 97.5%
quantiles of posterior distributions.
β
0
β
1
mean -5.799 0.110
st. dev. 1.001 1.005
MC error 5.486e-3 5.177e-3
˜
x
x
x
50
-5.802 0.113
˜
x
x
x
2.5
-7.762 -1.853
˜
x
x
x
97.5
-3.827 2.075
MC error/stdev 0.548% 0.515%
For comparison, the betareg package was used
for beta regression in frequentist statistical framework
(Ferrari and Cribari-Neto, 2004). The model had the
same structure, the link function was identically the
logit. Coefficients estimates were
ˆ
β
0
= −5.866 and
ˆ
β
1
= 0.115, respectively, model precision was 2578.
ACKNOWLEDGEMENTS
The research project is supported by the grant M
ˇ
SMT
7D12004 (E!7262 ProDisMon).
REFERENCES
Bayes, C. L., Baz´an, J. L., and Garc´ıa, C. (2012). A new ro-
bust regression model for proportions. Bayesian Anal-
ysis, 7(4):841–866.
Boyd, S. and Vandenberghe, L. (2004). Convex Optimiza-
tion. Cambridge University Press.
Branscum, A. J., Johnson, W. O., and Thurmond, M. C.
(2007). Bayesian beta regression: Applications to
household expenditure data and genetic distance be-
tween foot-and-mouth disease viruses. Australian &
New Zealand Journal of Statistics, 49(3):287–301.
Dempster, A. P., Laird, N. M., and Rubin, D. B. (1977).
Maximum likelihood from incomplete data via the
EM algorithm. Journal of the Royal Statistical So-
ciety. Series B (Methodological), 39(1):1–38.
Ferrari, S. and Cribari-Neto, F. (2004). Beta regression for
modelling rates and proportions. Journal of Applied
Statistics, 31(7):799–815.
Gelman, A., Carlin, J. B., Stern, H. S., and Rubin, D. B.
(2003). Bayesian Data Analysis, Second Edition
(Chapman & Hall/CRC Texts in Statistical Science).
Chapman and Hall/CRC, 2 edition.
Geman, S. and Geman, D. (1984). Stochastic relaxation,
Gibbs distributions, and the Bayesian restoration of
images. IEEE Trans. Pattern Anal. Mach. Intell.,
6:721–741.
Hahn, E. (2008). Mixture densities for project management
activity times: A robust approach to PERT. European
Journal of Operational Research, 188(2):450–459.
Jaakkola, T. and Jordan, M. (2000). Bayesian parameter es-
timation via variational methods. Statistics and Com-
puting, 10(1):25–37.
Jordan, M. I. (1999). An introduction to variational meth-
ods for graphical models. In Machine Learning, pages
183–233.
Kullback, S. and Leibler, R. A. (1951). On information
and sufficiency. The Annals of Mathematical Statis-
tics, 22(1):79–86.
Ma, Z. and Leijon, A. (2011). Bayesian estimation of
beta mixture models with variational inference. Pat-
tern Analysis and Machine Intelligence, IEEE Trans-
actions on, 33(11):2160–2173.
Metropolis, N., Rosenbluth, A. W., Rosenbluth, M. N.,
Teller, A. H., and Teller, E. (1953). Equation of state
calculations by fast computing machines. The Journal
of Chemical Physics, 21(6):1087–1092.
Minka, T. P. (2001). Expectation propagation for approx-
imate Bayesian inference. In UAI ’01: Proceedings
of the 17th Conference in Uncertainty in Artificial In-
telligence, pages 362–369, San Francisco, CA, USA.
Morgan Kaufmann Publishers Inc.
Ospina, R. and Ferrari, S. (2010). Inflated beta distributions.
Statistical Papers, 51:111–126. 10.1007/s00362-008-
0125-4.
Rigby, R. A. and Stasinopoulos, D. M. (2005). Generalized
additive models for location, scale and shape. Jour-
nal of the Royal Statistical Society: Series C (Applied
Statistics), 54(3):507–554.
OverviewofBoundedSupportDistributionsandMethodsforBayesianTreatmentofIndustrialData
387