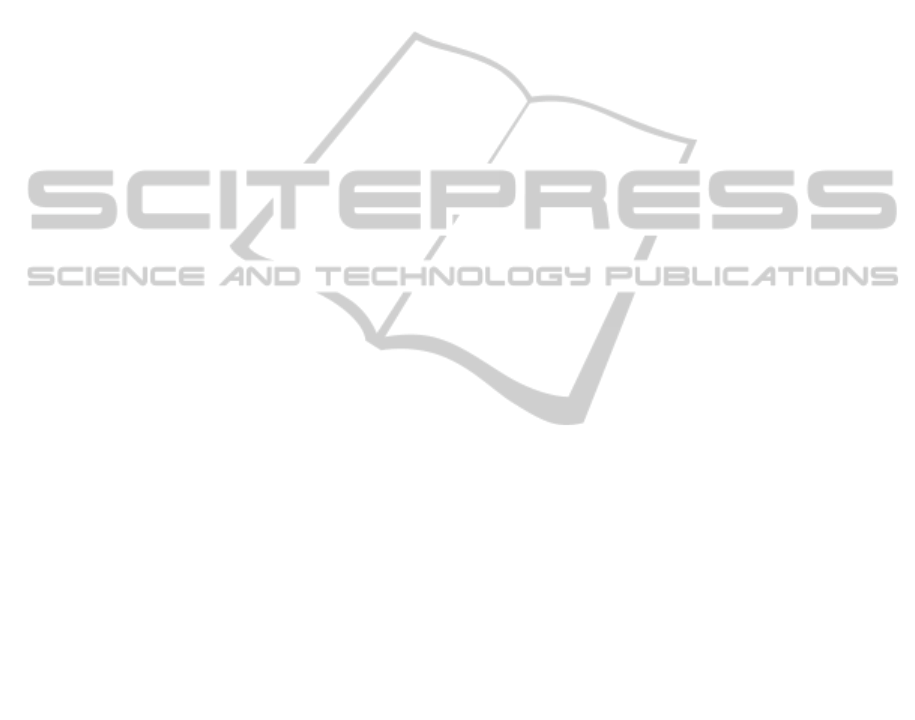
Technology in Teacher Education, vol. 2004, No. 1,
pp. 46-50.
Brookfield, S., 1995. Adult Learning: an overview.
Oxford: Pergamon.
Bubaš, G., Coric, A. & Orehovački, T., 2011. The
integration of students’ artifacts created with Web 2.0
tools into Moodle, blog, wiki,e-portfolio and Ning.
34th MIPRO International Convertion on Computers
in Education. Rijeka: Croation Society for Information
and Communication Technology, Electronics and
Microelectronics.
Buzzetto-More, N., 2010. Assessing the Efficacy and
Effectiveness of an E-Portfolio Used for Summative
Assessment. Interdisciplinary Journal of E-Learning
and Learning Objects, 6, pp. 61-85.
Candy, P.C., 2002. Lifelong learning and information
literacy. In White paper prepared for UNESCO, the
U.S. National Commission on Libraries and
Information Science, and the National Forum on
Information Literacy for use at the Information
Literacy Meeting of Experts. Prague, Czech Republic.
Cercone, K., 2008. Characteristics of adult learners with
implications for online learning design. AACE
Journal, 16(2), pp. 137-59.
De Goñi, J.I.M.M., 2006. What is adult education?
UNESCO answers. San Sebastián UNESCO Centre.
Field, J., 2011. Adult Learning, Health and Well-Being-
Changing Lives, Adult Learner. The Irish Journal of
Adult and Community Education, pp. 13-25.
Graf, S., Kinshuk, K. B., Khan, F. A., Maguire, P.,
Mahmoud, A., Rambharose, T., Shtern, V., Tortorella,
R. & Zhang, Q. 2012. Adaptivity and Personalization
in Learning Systems based on Students’
Characteristics and Context. In The 1st International
Symposium on Smart Learning Environment.
Grant, S., 2005. Clear e-portfolio definitions: A
prerequisite of interoperability. In 2005 ePortfolio
Conference. Cambridge.
Griesbaum, J., & Kepp, S. J., 2010. Facilitating
collaborative knowledge management and self-
directed learning in higher education with the help of
social software. concept and implementation of
collabuni–a social information and communication
infrastructure. In Proceedings of I-KNOW 2010, 10
International Conference on Knowledge Management
and Knowledge Technologies, pp. 415–426.
Guo, Z. & Greer, J., 2006. Electronic Portfolios as a
Means for Initializing Learner Models for Adaptive
Tutorials. In Innovative Approaches for Learning and
Knowledge Sharing. Berlin/Heidelberg, Springer.
Hämäläinen, H., Ikonen, J. & Nokelainen, I., 2011. The
Status of Interoperability in E-Portfolios: Case
Mahara. In 7th E-learning Conference, e-Learning'11
(E-Learning and the Knowledge Society). Bucharest,
Romania, pp. 64-69.
Himpsl, K. & Baumgartner, P., 2009. Evaluation of e-
Portfolio Software. International Journal of Emerging
Technologies in Learning 2009, 4(1), pp. 16-22.
Knight, A. & Bush, F., 2009. The development of an
integrated learning environment. In ascilite Auckland.
Knowles, M., 1980. The Modern Practice of Adult
Education. In Pedagogy to Androgogy. Chicago, pp.
263-264.
Lorenzo, G. & Ittelson, J., 2005. An overview of e-
portfolios. EduCause Learning Initiative Paper, 1.
Merriam, S.B., 2001. Andragogy and Self-Directed
Learning:Pillars of Adult Learning Theory. New
Directions for Adult and Continuing Education, 89,
pp. 3-13.
Niedritis, A., Niedrite, L, Kozmina, N., 2011. Integration
Architecture of User Models. Perspectives in Business
Informatics Research, Local Proceedings, BIR 2011,
Latvia Riga, pp. 323-330.
Orehovački, T., Bubaš, G., Kovačić, A., 2012. Taxonomy
of Web 2.0 applications with educational potential. In:
Transformation in Teaching: Social Media Strategies
in Higher Education. Informing Science Institute,
Santa Rosa, pp. 43–72.
Raybourn, E., & Regan, D., 2011. Exploring e-portfolios
and Independent Open Learner Models: Toward Army
Learning Concept 2015. In The Interservice/Industry
Training, Simulation & Education Conference
(I/ITSEC) (Vol. 2011, No. -1). National Training
Systems Association.
Seldin, P., 1997. The teaching portfolio: A practical guide
to improved performance and promotion/tenure
decisions, 2nd ed. Boston: Anker.
Van Der Sluijs, K & Houben, GJ. 2006. A generic
component for exchanging user models between web-
based systems, International Journal of Continuing
Engineering Education and Life Long Learning, vol.
16, no. 1, pp. 64-76.
Sweat-Guy, R. & Buzzetto-More, N., 2007. A
comparative analysis of common e-portfolio platforms
and available features. Issues in Informing Science and
Information Technology Education, 5(1), pp. 327-42.
The Lifelong Learning Programme: education and training
opportunities for all, 2011. Available from:
<http://ec.europa.eu/education/lifelong-learning-
programme/doc78_en.htm > [30 March 2013].
Vagale, V. & Niedrite, L., 2012a. Intellectual Ability Data
Obtaining and Processing for E-learning System
Adaptation. In Workshops on Business Informatics
Research. Springer Berlin Heidelberg, pp. 117-1.
Vagale, V. & Niedrite, L., 2012b. Learner Model’s
Utilization in the e-Learning Environments. In
Databases and Information Systems, DB&IS 2012.
Lithuania Vilnius:Žara, pp. 162-174.
Walsh, E., O'Connor, A. & Wade, V., 2011. Supporting
Learner Model Exchange in Educational Web
Systems. In 7th International Conference on Web
Information Systems and Technologies.
Noordwijkerhout, The Netherlands.
Zubizarreta, J., 2004. Reflective practice for improving
student learning. In The learning portfolio. Bolton,
MA: Anker.
ICEIS2013-15thInternationalConferenceonEnterpriseInformationSystems
496