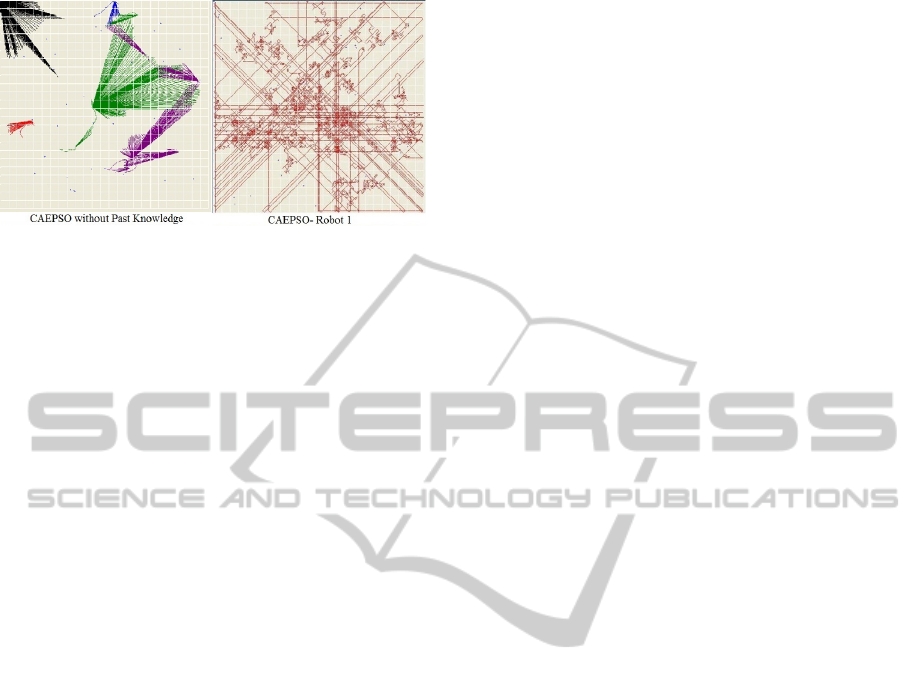
Figure 6: The testing phase trajectory traces of robots con-
trolled by CAEPSO with (a) and without application of past
knowledge. Different colors represent different robots. The
survivor rescuing tasks are time dependent and the environ-
ment is corrupted by illusion.
The figure indicate occasional stagnation and robots
inability to map a high percentage of the environment.
In contrast, as evidenced in sub figure b, when past
knowledge is presented to robots considerable per-
centage of the environment is mapped by each robot.
This is also evident from the difference in the quality
of the made decisions presented in tables 2 and 3. The
combination of the presented results in tables 2 and 3
and fig 6 suggest the importance of knowledge trans-
fer in environments polluted by combination of noises
originating from different sources as in illusion noise.
6 CONCLUSIONS
This study discussed the impact of past knowledge
on decisions made by a group of robots controlled
by two variations of PSO called Area Extended PSO
(AEPSO) and Cooperative AEPSO (CAEPSO). In or-
der to evaluate such an impact a type of noise called
Illusion effect is simulated. The illusion effect repre-
sent an iteratively changing noise that is the outcome
of some combinations of noises originating from dif-
ferent sources located somewhere near or far away.
The results of simulated experiments indicates the im-
portant role of past knowledge in compensating the il-
lusion noise and making correct decisions by the sim-
ulated robots.
REFERENCES
Atyabi, A. (2009). Navigating agents in uncertain environ-
ments using particle swarm optimization. In MSc The-
sis. Multimedia university of Malaysia.
Atyabi, A., Amnuaisuk, S. P., and Ho, C. K. (2010). Navi-
gating a robotic swarm in an uncharted 2d landscape.
In Appl. Soft Comput, pages 149–169. Elsevier.
Atyabi, A. and Samadzadegan, S. (2011). Particle swarm
optimization: A survey. In In Louis P. Walters, ed. Ap-
plications of Swarm Intelligence. Hauppauge, USA:
Nova Publishers, pages 167–178.
Grosan, C., Abraham, A., and Chis, M. (2006). Swarm
intelligence in data mining. In Springer, studies in
computational intelligence (SCI), pages 34:1–20.
Jakob, S. V. and Jacques, R. (2002). Particle swarms ex-
tensions for improved local, multi-modal, and dy-
namic search in numerical optimization. In MS.c The-
sis, Dept. Computer Science, Univ Aarhus, Aarhus C,
DenmarK.
Jim, P. and Martinoli, A. (2007). Inspiring and modeling
multi-robot search with particle swarm optimization.
In Proceeding of the 2007 IEEE Swarm Intelligence
Symposium (SIS2007).
Kennedy, J. and Eberhart, R. (1995). Particle swarm op-
timization. In IEEE Press Proceedings of the 1995
IEEE International Conference on Neural Networks,
pages 1942–1948.
Majid, N. A., Masoud, A., and Eiji, N. (2001). Cooperative
q-learning: the knowledge sharing issue. In Advanced
Robotics, pages 15(8): 815 – 832.
Mauris, C. (2002). The particle swarm - explosion, stability,
and convergence in multidimensional complex space.
In IEEE Transaction on Evolutionary.
Park, K. H., Kim, Y. J., and Kim, J. H. (2001). Modu-
lar q-learning based multi-agent cooperation for robot
soccer. In Robotics and Autonomous Systems, pages
34:109–122.
Peter, J. A. (1998). Evolutionary optimization versus parti-
cle swarm optimization: Philosophy and performance
differences. In Evolutionary Programming VII, Lec-
ture Notes in Computer Science, Springer.
Sousa, T., Neves, A., and Silva, A. (2004). Particle swarm
based data mining algorithms for classification tasks.
In IEEE Parallel and nature-inspired computational
paradigms and applications, pages 30:767–783.
Suranga, H. (2006). Distributed online evolution for swarm
robotics. In Autonomous Agents and Multi Agent Sys-
tems.
Tangamchit, P., Dolan, J. M., and Khosla, P. K. (2003). Cru-
cial factors affecting cooperative multi-robot learning.
In Proceeding of the 2003 IEEE/RSJ Intl conference
on intelligent Robots and Systems, Las Vegas, Nevada,
pages 2:2023–2028.
Yang, E. and Gu, D. (2004). Multi-agent reinforcement
learning for multi-robot systems: A survey. In Techni-
cal report, University of Essex Technical Report CSM-
404, Department of Computer Science.
ICINCO2013-10thInternationalConferenceonInformaticsinControl,AutomationandRobotics
184