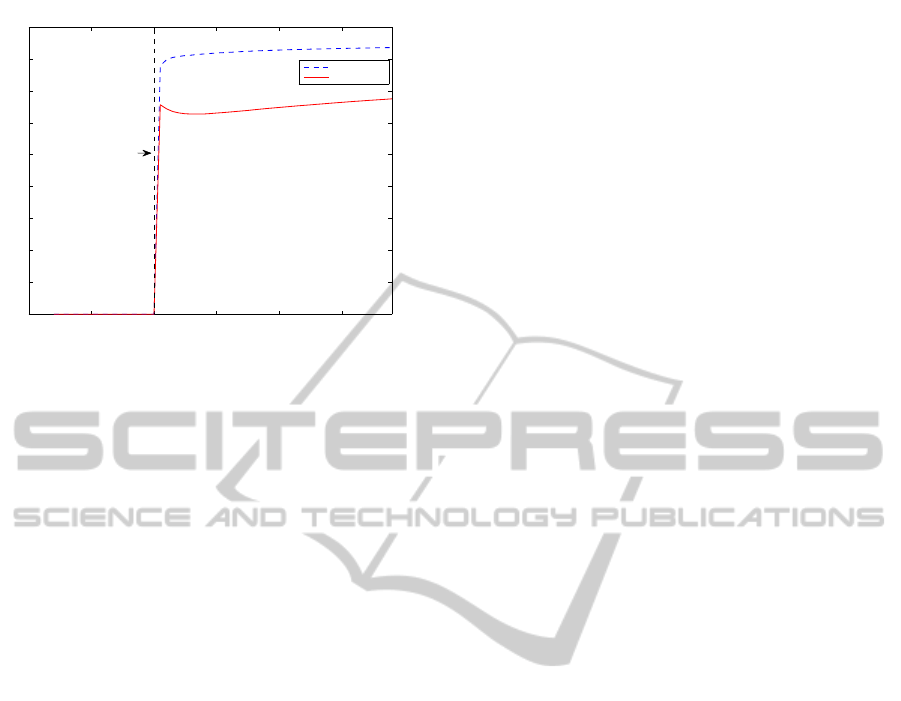
0 0.5 1 1.5 2 2.5
x 10
5
0
2
4
6
8
10
12
14
16
18
Sample time
Distance L
5% short circuit
2% short circuit
Fault
occurrence
Figure 9: The distance L in healthy and faulty case (2%, 5%
short circuit).
6 CONCLUSIONS
In this article a fault detection scheme for different
percentageof stator winding short circuit in one phase
of three phase induction motors is presented. The mo-
tor’s model was identified by the utilization of a Least
Squares Set Membership Identification (SMI) algo-
rithm, where additional to the identified parameters,
confidence intervals have been calculated, These in-
tervals in an µ–dimensional space can be represented
as hyper–ellipsoids having as a center the identified
parameters’ vector and thus a geometrical fault detec-
tion scheme has been proposed, which relied on the
calculation of the distance among centers of hyper–
ellipsoids and the corresponding intersections. Ex-
tended simulation results were presented that proven
the efficiency of the suggested scheme.
REFERENCES
A. Widodo, a. B. Y. (2008). Wavelet support vector ma-
chine for induction machine fault diagnosis based on
transient current signal. Expert Systems with Applica-
tions, 35:307316.
Aydin, I., Karakose, M., and Aki, E. (2011). A new
method for early fault detection and diagnosis of bro-
ken rotor bars. Energy Conversion and Management,
52(4):1790–1799.
B. Mirafzal, N. A. O. D. (2006). On innovative methods of
induction motor interturn and broken-bar fault diag-
nostics. IEEE TRANSACTIONS ON INDUSTRY AP-
PLICATIONS, 42(2).
Bachi, S., Tnani, S., Trigeassou, J., and Champenois,
G. (2006). Diagnosis by parameter estimation of
stator and rotor faults occurring in induction ma-
chines. IEEE Transactions on Industrial Electronics,
53(3):963–973.
Chen, S. and Zivanovic, R. (2009). Modelling and sim-
ulation of stator and rotor fault conditions in induc-
tion machines for testing fault diagnostic techniques.
European Transactions On Electrical Power, 20:611–
629.
Deller, J. (1989a). Set membership identification in digital
signal processing. IEEE ASSP Magazine, 6(4):4–20.
Deller, J. R. (1989b). Set membership identification in dig-
ital signal processing. IEEE ASSP Magazine, 6(4):4–
20.
F. Jawad, O. M. (2009). Different indexes for eccentricity
faults diagnosis in three-phase squirrel-cage induction
motors a review. Mechanical Sustem and Signal Pro-
cessing, 19:213.
Gaeid, K. S. and Mohamed, H. A. F. (2010). Bibliogra-
phy on induction motors faults detection and diagno-
sis. Australian Journal of Basic and Applied Sciences,
4(2):227–246.
Guastafsson, F. (Sep.2001). Adaptive Filtering and Change
Detection.
Kurzhanskiy, A. A. and Varaiya, P. (2008). Ellipsoidal tool-
box manual.
Le, K., Huang, Z., Moon, C., and Tzes, A. (2008). Fault
detection based on orthotopic set membership iden-
tification for robot manipulators. Proceedings of the
17th World Congress The International Federation of
Automatic Control , Seoul, Korea,2008, pages 7344–
7349.
Mustafa, M. O., Nikolakopoulos, G., and Guastafsson, T.
(2012a). Stator winding short circuit fault detec-
tion based on set membership identification for three
phase induction motors. Proceedings of IEEE, 20th
Mediterranean Conference on Control and Automa-
tion, MED12, pages 290–296.
Mustafa, M. O., Nikolakopoulos, G., and Gustafsson, T.
(2012b). A fault diagnosis scheme for three phase
induction motors based on uncertainty bounds. Pro-
ceedings Of The 38th Annual Conference of the IEEE
Industrial Electronics Society IECON, pages 1606–
1611.
Nandi, S. and Toliyat, H. (2005). Condition monitoring and
fault diagnosis of electrical machines-a review. IEEE
Transactions on Energy Conversion, 20(4):719–729.
Vas, P. (1992). Electrical Machines and Drives.
Widodo, A., Yang, B. S., and Han, T. (2007). Combination
of independent component analysis and support vector
machines for intelligent faults diagnosis of induction
motors. Expert Systems with Applications, 32:299–
312.
ICINCO2013-10thInternationalConferenceonInformaticsinControl,AutomationandRobotics
212