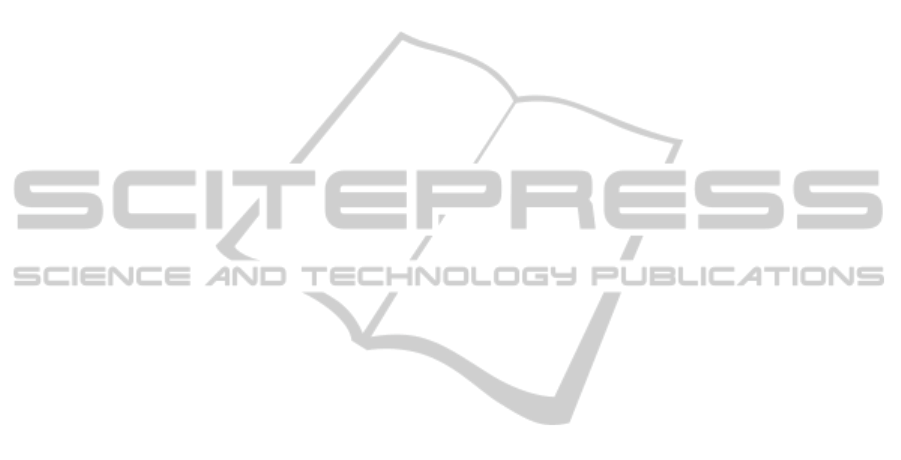
(positive/negative) visualization of trends and
events, with only few of them embedding neutral
opinions. The innovation of the proposed tools is
that it is not limited in a positive-negative scale, but
it is extended in order to capture a wider spectrum of
humans’ emotions.
The graphical representation of humans’
emotions on maps leads to easier understandable and
efficiently organized results. The tool can be useful
for the identification of social trends and events’
impact. It can also provide an unprecedented level of
analytics for companies interested in promoting their
presence and products, authorities interested in
promoting a better way of living in particular
geographical context, and individual users
depending on their specific needs.
6 CONCLUSIONS
Micro-blogging services (especially Twitter) has
brought much attention recently as a hot research
topic in the domain of sentiment analysis. Existing
approaches mainly focus on the evaluation of tweets
emotional orientation on a dual basis i.e. positive or
negative. Our work, offers a 3-tier framework for
emotion-aware microblogging analysis, and extends
this emotional spectrum in six emotions, offering
thus a more fine-grained analysis of users’ emotions.
The overall process is based on emotional
dictionaries and considers linguistic parameters,
(intensifiers and valence shifters), to result in a more
accurate evaluation of the expressed emotions. The
proposed framework is the basis for mobile
applications which summarize and depict crowds’
emotions towards a specific topic and within a
certain locality. Such mobile application tools are of
great importance in capturing branding success,
diffusion in market and emotional states in relevance
to different topics (such as events, campaigns etc),
as expressed by people.
In the future we aim to extend our work by
incorporating more multi-language dictionaries that
will make possible the analysis of tweets written in
languages other than English and also to enhance
offered services to more areas and thematic
categories. Particular clustering algorithms are under
development for summarizing microblogging posts
in a more efficient manner.
REFERENCES
Benamara F., Cesarano C., and Reforgiato D., 2007.
“Sentiment Analysis: Adjectives and Adverbs are
better than Adjectives Alone”, AAAI International
Conference on Weblogs and Social Media Boulder,
CO USA.
Bollen J., Pepe A., and Mao H. 2010. “Modeling public
mood and emotion: Twitter sentiment and socio-
economic phenomena”, International Conference on
WWW2010, April 26-30, 2010, Raleigh, North
Carolina.
Ekman, P., Friesen, W. V., & Ellsworth, P., 1982. “What
emotion categories or dimensions can observers judge
from facial behavior?” In Emotion in the human face,
pp. 39-55. Cambridge University Press, 1982.
Gill A. J., French R. M., Gerle D. and Oberlander J., 2008.
“Identifying Emotional Characteristics from Short
Blog Texts”, Proc. of the 30th Annual Conference of
the Cognitive Science Society, 2237-2242, 2008.
Godbole N., Srinivasaiah M., and Skiena S., 2007. “Large-
Scale Sentiment Analysis for News and Blogs”, AAAI
International Conference on Weblogs and Social
Media (ICWSM’ 2007) Boulder, Colorado, USA.
Maite T., Julian B., Milan T., Kimblerly V., Manfred S.,
2010. “Lexicon-Based Methods for Sentiment
Analysis”, Association for Computational Linguistics.
O’ Connor B., Balasubramanyan R., Routledge B. R. and
Smith N. A., 2010. “From Tweets to Polls: Linking
Text Sentiment to Public Opinion Time Series”, Proc.
of the International AAAI conference on Weblogs and
Social Media, Washington DC, 2010
Pak A., Paroubek P., 2010. “Twitter as a corpus for
Sentiment Analysis and Opinion Mining”, Proc. of the
7th conference on International Language Resources
and Evaluation, 1320-1326, 2010.
Pang B., Lee L., and Vaithyanathan S., 2002. “Thumbs
up? Sentiment Classification using Machine Learning
Techniques”, Proc. of the Conference on Empirical
Methods in Natural Language Processing (EMNLP).
Scherer, K.R., 2001. “Appraisal Considered as a Process
of Multi-Level Sequential Checking”, in Appraisal
Processes in Emotion: Theory, Methods, Research, pp.
92–120, Oxford University Press.
Tsagkalidou K., Koutsonikola V., Vakali A., Kafetsios K.,
2011. “Emotional aware clustering on micro blogging
sources”, In ACII'11 Proceedings of the 4th
International conference on Affective Computing and
Intelligent Interaction pp.387-396.
Turney, P., 2002. “Thumbs Up or Thumbs Down?
Semantic Orientation Applied to Unsupervised
Classification of Reviews”, Proc. 40th Annual Meeting
of the Association for Computational Linguistics.
Yessenalina A., Cardie C., 2011. “Compositional Matrix-
Space Models for Sentiment Analysis”, Proc. of the
2011 Conference on Empirical Methods in Natural
Language Processing, pages 172-182, 2011.
DATA2013-2ndInternationalConferenceonDataManagementTechnologiesandApplications
182