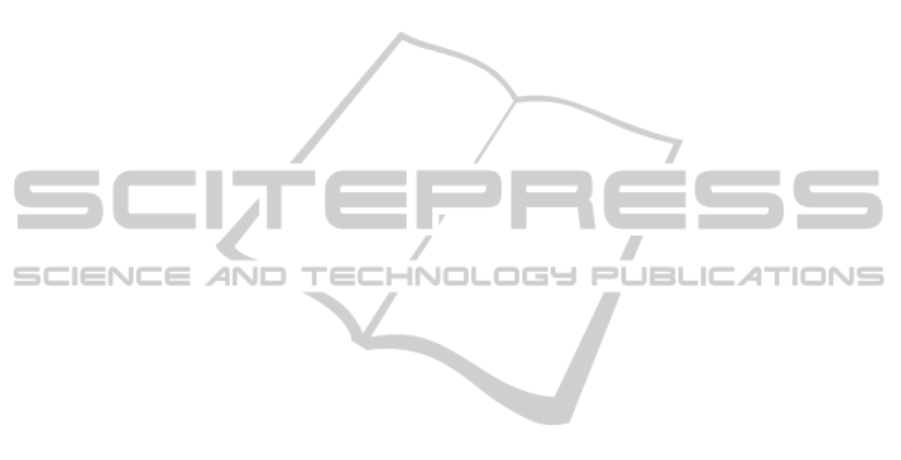
tipping effects. The analysis procedure results in best-
fit models that explicitly depict precise and dynamic
mechanisms. Equations such as 5 and 6 provide the
researcher with rich information beyond correlation
coefficients, since they express how variables change
with respect to each other’s state. In future studies, we
will show how the same mechanisms can be used to
look at three and more variables(Ranganathan et al.,
2013).
A key feature of our approach is that no prede-
fined model is imposed on the data. Instead the data
itself is used to find the best model. The same ap-
proach of calculating Bayes factor can of course be
used to test theoretically informed model specifica-
tions. Such testing can tell us how the best fit data-
driven model compares in terms of statistical fit, to
a model based on theoretical reasoning. There may
well be strong grounds to accept a theoretically jus-
tified model with a slightly worse fit, over a purely
data-driven model with the best fit. Indeed, we do not
suggest that social scientists should forget about the-
ories and always adopt the statistically best models.
No doubt, theories are useful to interpret results and
to evaluate models. But we think that social scientists
should be equally open to finding meaningful patterns
and mechanisms beyond established theories. If the
detected patterns and models are plausible and help to
understand social reality or give a new insight into a
phenomenon, then even new theoretical mechanisms
could be formulated or older theoretical mechanisms
revised, based on these findings.
REFERENCES
Allison, P. (2005). Fixed Effects Regression Methods for
Longitudinal Data. SAS Publishing.
Amemiya, T. (1985). Advanced econometrics. Blackwell,
Oxford.
Andersen, R. (2007). Modern Methods for Robust Regres-
sion. SAGE, London.
Ashimov, A. A., Sultanov, B. T., Adilov, Z. M., Borovskiy,
Y. V., Novikov, D. A., Nizhegorodtsev, R. M., and
Ashimov, A. A. (2011). Macroevonomic Analysis
and Economic Policy Based on Parametric Control.
Springer.
Barro, R. J. (1996). Democracy and growth. Journal of
Economic Growth, 1.
Barro, R. J. (1999). Determinants of democracy. Journal of
Political Economy, 107.
Bishop, C. M. (2006). Pattern recognition and machine
learning. Springer.
Boix, C. and Stokes, S. (2003). Endogenous democratisa-
tion. World Politics, 55.
Coleman, J. S. (1964). Introduction to Mathematical Soci-
ology. Free Press of Glencoe/Collier Macmillan.
de Marchi, S. (2005). Computation and Mathematical Mod-
eling in the Social Sciences. Cambridge University
Press.
Diamond, L. and Marks, G. (1992). Reexamining Democ-
racy. SAGE.
Garson, G. D. (2013). Two-Stage Least Square Regression.
Statistical Associates Publishers.
Gelman, A. (2004). Exploratory data analysis for com-
plex models. Journal of Computational and Graph-
ical Statistics, 13 (4).
Krieckhaus, J. (2003). The regime debate revisited: A sensi-
tive analysis of democracy’s economic effect. British
Journal of Political Science, 34.
Laird, N. M. and Ware, J. H. (1982). Random-effects mod-
els for longitudinal data. Biometrics, 38 (4).
Lewis-Beck, M. S. (1995). Data Analysis: An Introduction.
SAGE, London.
Ley, E. and Steel, M. F. (2009). On the effect of prior as-
sumptions in bayesian model averaging with applica-
tions to growth regression. Journal of Applied Econo-
metrics, 24:651–674.
Lipset, S. M. (1959). Some social requisites of democ-
racy: Economic development and political legitimacy.
American Political Science Review, 53.
Menard, S. (2001). Applied Logistic Regression Analysis.
SAGE, London.
Ostrom, C. W. (1990). Time Series Analysis: Regression
Techniques. SAGE, London.
Ranganathan, S., Mann, R. P., Nikolis, S. C., Swain, R. B.,
and Sumpter, D. J. (2013). A dynamical systems ap-
proach to modeling human development. Economet-
rica. submitted.
Robert, C. P. (1994). The Bayesian Choice: a decision-
theoretic motivation. Springer-Verlag, New York.
Saperstein, A. M. (2000). Dynamical Modeling of the Onset
of War. World Scientific Publishing Company.
Stebbins, R. A. (2001). Exploratory Research in Social Sci-
ences. SAGE, London.
Strogatz, S. H. (2000). Nonlinear Dynamics and Chaos:
With Applications to Physics, Biology, Chemistry and
Engineering. Westview Press.
Treiman, D. L. (2009). Quantitative Data Analysis: Doing
Social Research to Test Ideas. Jossey-Bass.
Tukey, J. (1977). Exploratory Data Analysis. Addison-
Wesley.
Weber, L. (2012). Demographic Change and Economic
Growth: Simulation on Growth Modeling. Physica.
Welzel, C. (2013). Freedom Rising. Human Empowerment
and the Quest for Emancipation. Cambridge Univer-
sity Press.
Wooldridge, J. M. (2010). Econometric Analysis of cross
section and panel data. MIT Press.
ABayesianApproachtoModelingDynamicalSystemsintheSocialSciences
131