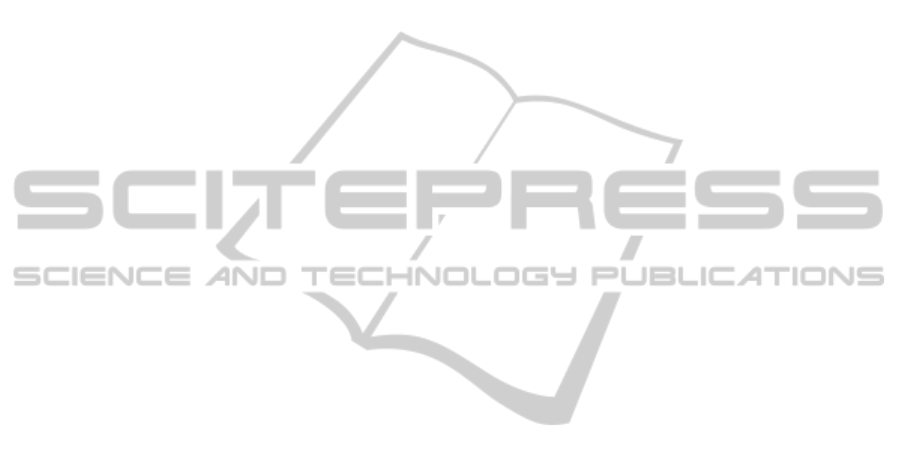
that only few Trisarea features are relevant to
recognition processes. Statistics on relevant features
was performed and this analysis shows that relevant
triangles are, in most cases, formed by a swing of
non-adjacent joints with unconstrained movements in
3D space (i.e. elbows, knees or wrist) with respect
to a joint with a constrained position from torso
reference (i.e. shoulder, hip or neck).
The comparison with other recognition
approaches denotes that our approach performs
reasonably well. For this testbed, our results
outperform (Li et al., 2010) approach. On the other
hand, (Vieira et al., 2012; Miranda et al., 2012) have
better results because they have a more accurate
temporal information about motion. However, our
approach have the most compact representation and
surely is faster than previous pose-based methods as
(Miranda et al., 2012).
As conclusion, the results show the usefulness
of Trisarea features extraction to perform a motion
feature space that permits us the use of classical
and powerful Machine Learning methods to perform
human motion recognition. Although we have
obtained promising results with the Pearsons
coefficient of variation, this measure is ambiguous
for similar movements and we have to explore other
options to encode the dynamic behavior of Trisarea
features in motion sequences.
7 CONCLUSIONS
A new relative pose feature called Trisarea has been
presented and its use for human motion recognition
has been proposed. Specifically, a motion descriptor
based on the evolution of Trisarea features has
been performed. A Principal Feature Analysis has
provided good results selecting the most relevant
Trisarea features with the objective of reducing the
dimension of the obtained feature vector for posterior
recognition steps.
With the filtered motion descriptors, a single
instance of a motion has been proposed applying
Pearsons relative coefficient of variation for each
Trisarea feature over time. These motion instances
have been generated over three different datasets in
order to verify recognition results.
As a result, the experiments have demonstrated
the usefulness of Trisarea features for human
motion recognition tasks. A comparison with other
approaches in the same scenario has revealed that
our recognition results have not been far from other
methodologies results. In addition, the presented
approach has got a good accuracy/speed ratio because
it has less preprocessing calculations than the
compared pose-based approaches.
Motivated with the presented results, in a future
scope we will explore some kind of temporal
modelling in order to deal with changes of order of
motion execution and will test them on-line in real
world scenarios.
ACKNOWLEDGEMENTS
This work has been done under project IPRES,
DPI2011-29660-C04-01 of the Spanish National
Research Program, with partial FEDER funds.
REFERENCES
Aggarwal, J. and Ryoo, M. (2011). Human activity
analysis: A review. ACM Computing Survey,
43(3):16:1–16:43.
Chen, C., Zhuang, Y., Xiao, J., and Liang, Z. (2009).
Perceptual 3D pose distance estimation by boosting
relational geometric features. Computer Animation
and Virtual Worlds, 20(2-3):267277.
Ellis, C., Masood, S. Z., Tappen, M. F., Laviola, Jr., J. J.,
and Sukthankar, R. (2013). Exploring the trade-off
between accuracy and observational latency in action
recognition. International Journal of Computer
Vision, 101(3):420–436.
Gorelick, L., Blank, M., Shechtman, E., Irani, M.,
and Basri, R. (2007). Actions as space-time
shapes. Transactions on Pattern Analysis and
Machine Intelligence, 29(12):2247–2253.
Gu, J., Ding, X., Wang, S., and Wu, Y. (2010). Action
and gait recognition from recovered 3-d human joints.
Trans. Sys. Man Cyber. Part B, 40(4):1021–1033.
Li, W., Zhang, Z., and Liu, Z. (2010). Action recognition
based on a bag of 3d points. In Computer Vision
and Pattern Recognition Workshops (CVPRW), 2010
IEEE Computer Society Conference on, pages 9–14,
Washington, DC, USA. IEEE Computer Society.
Lu, Y., Cohen, I., Zhou, X. S., and Tian, Q. (2007).
Feature selection using principal feature analysis. In
Proceedings of the 15th international conference on
Multimedia, pages 301–304, New York, NY, USA.
ACM.
Luo, X., Berendsen, B., Tan, R. T., and Veltkamp, R. C.
(2010). Human pose estimation for multiple persons
based on volume reconstruction. In Proceedings of
the 2010 20th International Conference on Pattern
Recognition, pages 3591–3594, Washington, DC,
USA. IEEE Computer Society.
Matikainen, P., Hebert, M., and Sukthankar, R. (2010).
Representing pairwise spatial and temporal relations
for action recognition. In Proceedings of the 11th
European conference on Computer vision: Part I,
pages 508–521, Berlin, Heidelberg. Springer-Verlag.
HumanMotionRecognitionfrom3DPoseInformation-Trisarea:ANewPose-basedFeature
81