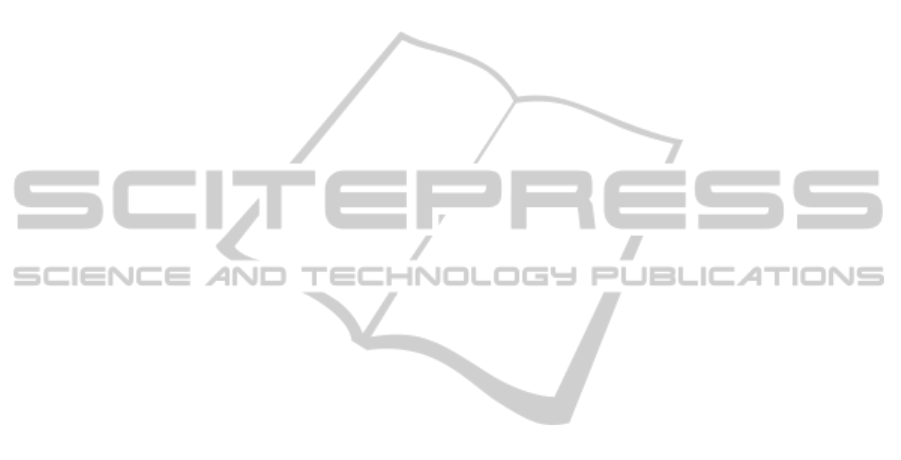
pen are normally related to a limited view onto the
wall, which either occurs when the wall is occluded
by some close object (e.g. truck), or the cameras tem-
porarily point away from the wall. If the optimiza-
tion was rejected we carry over the prediction as cur-
rent estimate and continue like that until the wall is in
proper view again.
The estimated plane parameters for both walls in a
sequence over 450 frames are plotted in Figure 8. The
upper diagram shows the angle between groundplane
and walls, the bottom diagram shows the plane dis-
tance parameters. The distances add up to the street
width, for this sequence with a mean of 9.3m.
The sequence begins on the left sidewalk and ends
on the right sidewalk after crossing the street. It con-
tains several parts in which the walls are out of view
due to the camera heading, some are shown in the
screen shots. As explained earlier, these parts are
bridged by predicting the parameters using the ego-
motion and are shaded in the diagram.
4 CONCLUSIONS
We have demonstrated two approaches towards esti-
mating the local, geometric structure in the scenario
of urban street canyons. We model the right and left
building walls as planar surfaces and estimate the un-
derlying plane parameters from 3D data points ob-
tained from a passive stereo-camera system, which is
replaceable by any kind of range sensor as long the
uncertainties of reconstructed 3D points are known
and can be considered.
The presented approaches are not intended as a
standalone version. Their purpose is rather to separate
a set of inlier points fitting the plane model to initial-
ize optimization procedures as we applied in form of
the iterative least-squares. By taking visual odometry
in combination with a prediction and update step into
the loop we are able to present a stable approach to
keep track of groundplane and both walls.
Future work includes integrating the rich informa-
tion offered by the depth-registered image intensity
values and relaxing the assumptions implied by the
street canyon scenario.
ACKNOWLEDGEMENTS
The work was supported by the German Federal Min-
istry of Education and Research within the project
OIWOB. The authors would like to thank the
”Karlsruhe School of Optics and Photonics” for sup-
porting this work.
REFERENCES
Barinova, O., Lempitsky, V., Tretiak, E., and Kohli, P.
(2010). Geometric image parsing in man-made en-
vironments. ECCV’10, pages 57–70, Berlin, Heidel-
berg. Springer-Verlag.
Borrmann, D., Elseberg, J., Lingemann, K., and N
¨
uchter, A.
(2011). The 3d hough transform for plane detection in
point clouds: A review and a new accumulator design.
3D Res., 2(2):32:1–32:13.
Chumerin, N. and Van Hulle, M. M. (2008). Ground Plane
Estimation Based on Dense Stereo Disparity. ICN-
NAI’08, pages 209–213, Minsk, Belarus.
Cornelis, N., Leibe, B., Cornelis, K., and Gool, L. V.
(2008). 3d urban scene modeling integrating recog-
nition and reconstruction. International Journal of
Computer Vision, 78(2-3):121–141.
Fischler, M. A. and Bolles, R. C. (1981). Random sample
consensus: a paradigm for model fitting with appli-
cations to image analysis and automated cartography.
Commun. ACM, 24(6):381–395.
Geiger, A., Lauer, M., and Urtasun, R. (2011a). A gen-
erative model for 3d urban scene understanding from
movable platforms. In CVPR’11, Colorado Springs,
USA.
Geiger, A., Ziegler, J., and Stiller, C. (2011b). Stereoscan:
Dense 3d reconstruction in real-time. In IEEE Intelli-
gent Vehicles Symposium, Baden-Baden, Germany.
Gutmann, J.-S., Fukuchi, M., and Fujita, M. (2008).
3d perception and environment map generation for
humanoid robot navigation. I. J. Robotic Res.,
27(10):1117–1134.
Hoiem, D., Efros, A. A., and Hebert, M. (2007). Recovering
surface layout from an image. International Journal
of Computer Vision, 75:151–172.
Hoover, A., Jean-baptiste, G., Jiang, X., Flynn, P. J., Bunke,
H., Goldgof, D., Bowyer, K., Eggert, D., Fitzgibbon,
A., and Fisher, R. (1996). An experimental compari-
son of range image segmentation algorithms.
Hough, P. (1962). Method and Means for Recognizing
Complex Patterns. U.S. Patent 3.069.654.
Iocchi, L., Konolige, K., and Bajracharya, M. (2000). Vi-
sually realistic mapping of a planar environment with
stereo. In ISER, volume 271, pages 521–532.
Labayrade, R., Aubert, D., and Tarel, J.-P. (2002). Real
time obstacle detection in stereovision on non flat road
geometry through ”v-disparity” representation. In In-
telligent Vehicle Symposium, 2002. IEEE, volume 2,
pages 646 – 651 vol.2.
Lee, D. C., Hebert, M., and Kanade, T. (2009). Geomet-
ric reasoning for single image structure recovery. In
CVPR’09.
Murray, D. R. and Little, J. J. (2004). Environment model-
ing with stereo vision. IROS’04.
Poppinga, J., Vaskevicius, N., Birk, A., and Pathak, K.
(2008). Fast plane detection and polygonalization in
noisy 3d range images. IROS’08.
Schindler, K. and Bischof, H. (2003). On robust regression
in photogrammetric point clouds. In Michaelis, B. and
Krell, G., editors, DAGM-Symposium, volume 2781 of
Lecture Notes in Computer Science, pages 172–178.
Springer.
Se, S. and Brady, M. (2002). Ground plane estimation, error
analysis and applications. Robotics and Autonomous
Systems, 39(2):59–71.
ICINCO2013-10thInternationalConferenceonInformaticsinControl,AutomationandRobotics
90