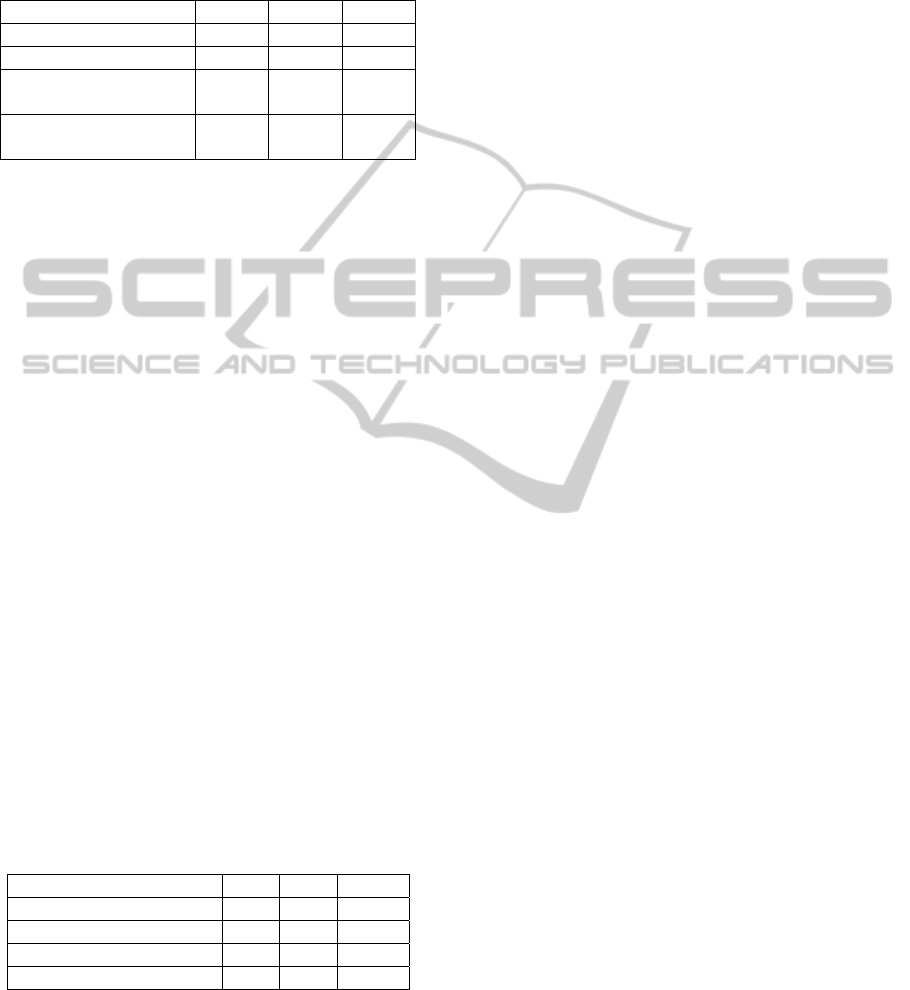
steps of normal walking speed and increasing
measurement and/or actuator noises (see
Table 1).
Table 1: Long-term cost during 20 steps of normal
walking speed and increasing measurement/actuator
noises.
Noise PD FLNN DNDP
2% measurement noise 0.715 0.239 0.075
5% measurement noise 3.96 2.003 0.118
5% measurement noise
and 2% actuator noise
3.961 2.079 0.120
5% measurement noise
and 5% actuator noise
3.966 2.336 0.130
PD – Proportional-Derivative control
FLNN –Feedback Linearization Neural Network control
For the comparison purpose, the model is
simulated with other types of control at the ankle
joint, including Proportional-Derivative control (PD)
and direct Feedback Linearization-based multilayer
Neural Network control (FLNN). Ideal computed
torque controls are still used at the hip and knee
joints given the assumption on the human ability in
generating normal gait. The average long-term cost
function as calculated by (14) is reported in Table 1.
It can be seen that as the measurement/actuator
noises increase, the DNDP-based control
outperforms other control methods by producing
robust tracking performance with lower long-term
cost.
5.4 Effect of Variations in Walking
Speed
Similar setups to Section 5.3 are repeated here to
evaluate the performance of the DNDP-based
control in the presence of variations in walking
speed. The model is simulated with 5%
measurement noise, 5% actuator noise, and 4
different walking setups (see
Table 2).
Table 2: Long-term cost with 5% measurement noise, 5%
actuator noise, and combinations of different walking
speeds.
Number of steps PD FLNN DNDP
10 normal + 10 fast
2.140 0.567 0.100
10 normal + 10 slow
3.910 1.915 0.106
10 normal + 5 fast + 5 slow
2.233 0.461 0.082
10 normal + 5 slow + 5 fast
2.206 0.490 0.084
Again, despite the variations in walking speed,
the DNDP-based control is still able to provide
lower long-term performance cost compared to other
control strategies.
6 CONCLUSIONS
The performance of a model-free Direct Neural
Dynamic Programming-based controller for a
prosthetic ankle joint was investigated in this paper.
Issues such as gait dynamics formulation, desired
ankle joint behaviours, control strategies, and long-
term gait-related efficiency were addressed in order
to implement the DNDP-based control approach. We
augmented the original training rules with additional
terms to provide robustness against the disturbance
generated by the ground reaction force. Results of
the simulation study indicate that the DNDP-based
control is stable, robust to measurement/actuator
noises and variations in walking speeds, and
improves the overall performance of the prosthetic
ankle. It is also observed that the generated ankle
torque is similar to the torque measured from
biological ankle during gait testing. The results of
this study serve as a starting point for the
development of intelligent ankle prosthesis. The
authors are currently pursuing research on adaptive
determination of gait using biofeedback signals
measured from below-knee amputees and
implementation of the DNDP-based control strategy
on actual prosthetic ankle joint.
REFERENCES
Amirouche, F. M. L. 1992. Computational Methods in
Multibody Dynamics, Prentice Hall.
Brugger, P. & Schemiedmayer, H.-B. 2003. Simulating
prosthetic gait - lessons to learn. Proceedings in
Applied Mathematics and Mechanics, 3, 64-67.
Bruneau, O. & Ouezdou, F. B. 1997. Compliant contact of
walking robot feet. 3rd ECPD International
Conference on Advanced Robotics. Bremen, Germany.
Enns, R. & Si, J. 2003. Helicopter trimming and tracking
control using direct neural dynamic programming.
IEEE Transactions on Neural Networks, 14, 929-939.
Hansen, A. H. 2005. Scientific methods to determine
functional performance of prosthetic ankle-foot
systems. Journal of Prosthetics and Orthotics, 17, 23-
29.
Hitt, J., Sugar, T., Holgate, M., Bellman, R. & Hollander,
K. 2009. Robotic transtibial prosthesis with
biomechanical energy regeneration. International
Journal of Industrial Robot, 36, 441-447.
iWalk. 2012. Bionic Technology with Powered Plantar
Flexion [Online]. Available: http://
www.iwalkpro.com/Prosthetists.html [Accessed Aug.
28th 2012].
Lewis, F. L., Jagannathan, S. & Yesilderek, A. 1999.
Neural network control of robot manipulators and
nonlinear systems, London, UK, Taylor & Francis.
ICINCO2013-10thInternationalConferenceonInformaticsinControl,AutomationandRobotics
24