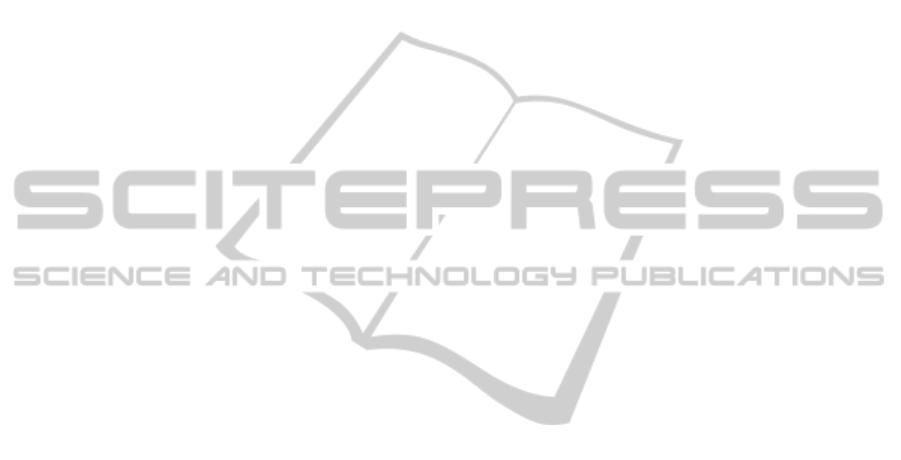
environment (see Fig. 8(b)). Plants grown in the
wide-open space tend to produce larger leaves length
with higher variability (in Fig. 9(b)). This isn’t the
case for plants grow in crowded condition. As
illustrated in Fig. 9(a), majority of the plants
continue to produce offspring with small leaf length,
a trait reflects receiving lesser sunlight.
5 FUTURE WORK
The above modeling framework shows the potential
of developing a simulated educational program to
educate students about shade-avoidance responses in
plants. Several improvements will be implemented
to bring the simulated response closer to nature. For
example, one improvement is to define the leaf area
equation and make light interception proportion to
leaf area. Another idea is to transfer this program
into graphic user interface (GUI), allowing students
play with the parameters to create different
experimental scenarios, learn, and observe the
simulated results (i.e. plants’ responses).
ACKNOWLEDGEMENTS
This research was supported through NSF Grant No.
0923752 to Weinig (PI), McClung, Welch, Das &
Maloof (co-PIs).
REFERENCES
Kincaid J. P. and Westerlund K. K., 2009. Simulation in
education and training, IEEE Proceedings of the 2009
Winter Simulation Conference (WSC), 273 – 280.
USC Annenburg Foundation, accessed December 19,
2012. The ReDistricting Game. Virginia Civics, Item
#273. available: http://vagovernmentmatters.org/web-
resources/273
Alinier G., 2007. A typology of educationally focused
medical simulation tools. Medical Teacher, 29(8),
243-250.
Kethireddy J. and Suthaharan S., 2004. Visualization
Teaching Tool for Simulation of OSI Seven Layer
Architecture. IEEE Proceesings in SoutheastCon, 335-
342.
Tinker N. A. and Mather D. E., 1993. GREGOR: software
for genetic simulation. Journal of Heredity, 84(3),
237-237.
Martin S. K. St. and Skavaril R.V., 1984. Computer
simulation as a tool in teaching introductory plant
breeding. Journal of Agronomic Education, 13, 43-47.
Fita A., Tarín N., Prohens J., and Rodríguez-Burruezo A.,
2010. A software tool for teaching backcross breeding
simulation. HortTechnology, 20(6), 1049-1053.
Deb K., 2001. Multi-Objective Optimization Using
Evolutionary Algorithms, 1st edn, John Wiley & Sons,
England, p. 123.
Engelbrecht A. P., 2007. Computational Intelligence: An
Introduction; John Wiley & Sons, England, p. 148-149
Casal J. J., 2012. Shade avoidance; Arabidopsis Book, 10,
ch. e0157, p. 1-19.
Franklin K. A. and Whitelam G. C., 2005. Phytochromes
and shade-avoidance responses in plants. Annals of
Botany, 96(2), 169-175.
Sasidharan R., Chinnappa C. C., Staal M., Elzenga J. T.
M., Yokoyama R., Nishitani K., Voesenek L. A.C.J.,
and Pierik R., 2010. Light quality-mediated petiole
elongation in Arabidopsis during shade avoidance
involves cell wall modification by xyloglucan
endotransglucosylase/hydrolases. Plant physiology,
154(2), 978-990.
Keuskamp D. H. and Pierik R., 2010. Photosensory cues
in plant–plant interactions: regulation and functional
significance of shade avoidance responses. Plant
Communication from an Ecological Perspective, 159-
178.
De Wit M., Kegge W., Evers J. B., Vergeer-van Eijk M.
H., Gankema P., Voesenek L. A. C. J., and Pierik R.,
2012. Plant neighbor detection through touching leaf
tips precedes phytochrome signals. Proceedings of the
National Academy of Sciences, 109(36), 14705-14710.
Hammer G. L, Dong Z., McLean G., Doherty A., Messina
C., Schussler J., Zinselmeier C., Paszkiewicz S., and
Cooper M., 2009. Can changes in canopy and/or root
system architecture explain historical maize yield
trends in the US corn belt? Crop Science, 49(1), 299-
312.
Smith H. and Whitelam G. C., 1997. The shade avoidance
syndrome: multiple responses mediated by multiple
phytochromes. Plant, Cell atid Environment, 20, 840-
844.
Eshelman L. J. and Schaffer J. D., 1993. Real-Coded
Genetic Algorithms and Interval-Schemata.
Foundations of Genetic Algorithms, 2, 187-202.
SIMULTECH2013-3rdInternationalConferenceonSimulationandModelingMethodologies,Technologiesand
Applications
170