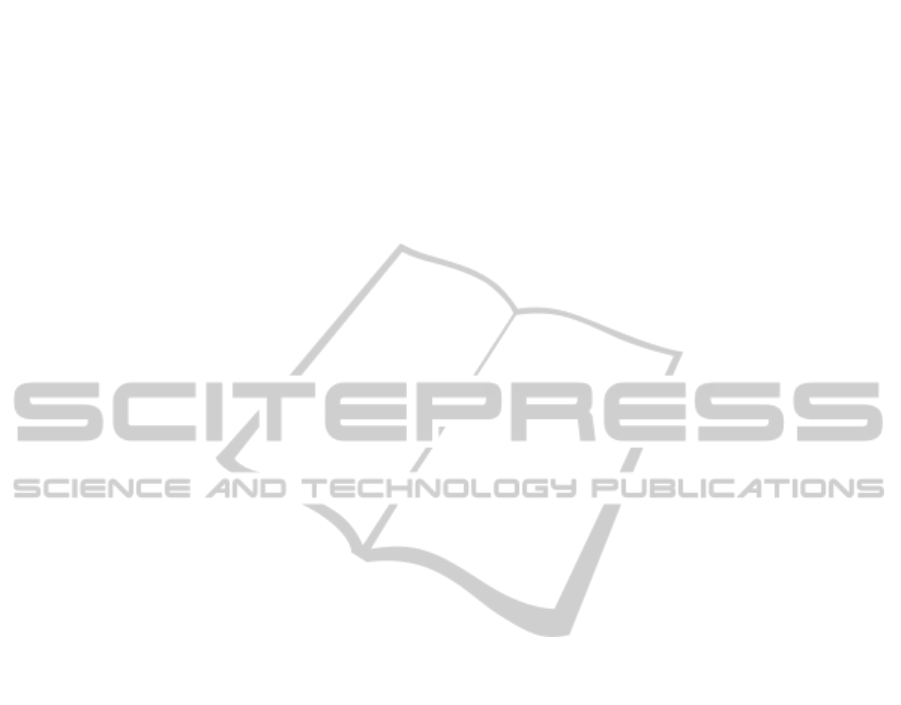
the optimal control problem solving for nonlinear
dynamic systems with one input and many outputs is
considered. The initial two-point optimal control
problem with integral functional was reduced to
extremum problem on real vectors field. With all the
transformations, listed above, the system with state
and co-state variables can be closed and the control
function structure can be defined in terms of the co-
state variables and system state. The seeking of
initial point for the co-state variables brings the
solution for the optimal control problem and
suggested to be based on stochastic or evolution
unconstrained global optimization algorithms.
As the optimization techniques evolutionary
strategies, differential evolution, particle swarm
optimization and their modifications were suggested
and investigated on the given optimal control
problems set. It is suggested that optimization
techniques with special features are required and
future works are related with designing hybrid
algorithms that allow both the extremum seeking
and surface scouting.
Since, there are plenty of different optimal
control problems, as the system equations and
functional differs from task to task, the mapping to
be optimized has different characteristics and there
is no set of the problems that allows making a
complete investigation of the optimization
algorithms efficiency. All in all, the investigation of
efficiency for heuristic techniques proceeds in the
same way, techniques are testing on the well-known
set of the objective functions. The proposed
approach leads to investigate the efficiency of
techniques with different extremum problem
definition, and it seems to be the only way to find
the most suitable algorithm by testing it on some set
of the control problems.
In the current investigation the hybrid
evolutionary strategies approach was the most
effective.
The aim of the further investigation is to design
the critique program agent, which will be the
controller for the optimization technique, via
analysing the data: fitness function set and its
history, the topology of the individuals and its
dynamics. The algorithms with implementation of
this agent probably will be more efficient for global
extremum problems.
REFERENCES
Popescu, M., Dumitrache, M., 2005: On the optimal
control of affine nonlinear systems. Mathematical
Problems in Engineering (4): pp. 465–475.
Chen, W. H., Balance, D. J., Gawthrop, P. J., 2003:
Optimal control of nonlinear systems: a predictive
control approach. Automatica 39(4): pp. 633-641.
Primbs, J. A., Nevistic, V., Doyle, J. C., 1999: Nonlinear
optimal control: a control Lyapunov function and
receding horizon perspective. Asian Journal of
Control, Vol. 1, No. 1: pp. 14-24.
Rao, A. V., 2009: Survey of Numerical methods for
Optimal Control, AAS/AIAA Astrodynamics
Specialist Conference, AAS Paper 09-334, Pittsburg,
PA.
Bertolazzi, E., Biral, F., Lio, M., 2005, Symbolic-Numeric
Indirect Method for Solving Optimal Control
Problems for Large Multibody Systems: The Time-
Optimal Racing Vehicle Example. Multibody System
Dynamics, Vol. 13, No. 2., pp. 233-252
Cruz, P., Torres, D., 2007, Evolution strategies in
optimization problems, Proc. Estonian Acad. Sci.
Phys. Math., 56, 4, pp. 299–309
Kirk, D. E., 1970: Optimal Control Theory: An
Introduction. Englewood Cliffs, NJ: Prentice-Hall.
Schwefel, H-P, 1995: Evolution and Optimum Seeking.
New York: Wiley & Sons.
Hansen, N., 2006: The CMA evolution strategy: a
comparing review. Towards a new evolutionary
computation. Advances on estimation of distribution
algorithms, Springer, pp. 1769–1776
Storn, R. Price, K.,1997: Differential Evolution - A Simple
and Efficient Heuristic for Global Optimization over
Continuous Spaces, Journal of Global Optimization,
11: pp. 341–359.
Kennedy, J., Eberhart, R., 2001: Swarm Intelligence.
Morgan Kaufmann Publishers, Inc., San Francisco,
CA.
AboutOptimizationTechniquesinApplicationtoSymbolic-NumericOptimalControlSeekingAproach
275