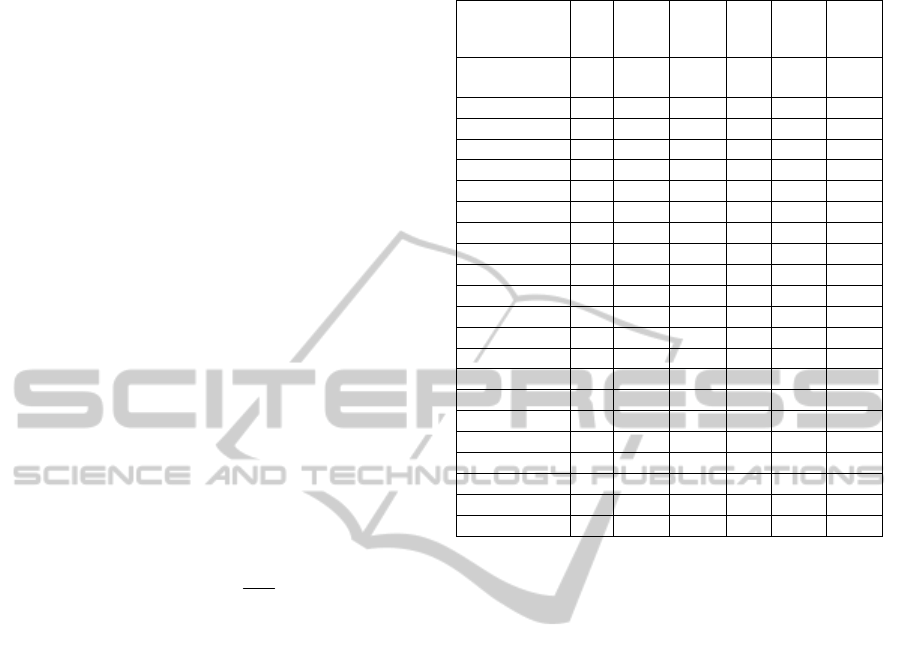
of
j
and
2
log
yj
at each design configuration
for each of the two primary performance criteria of
interest in this study. Note that only the throughput
rate (TR) seeks a maximization. The mean flow time
(MFT) and the variances of both TR and MFT need
be minimized. Therefore, the normalization
procedure of these values will consist of maximizing
the inverse. Further analysis of output results
indicates that design configurations labeled #3, 7,
16, 20, and 21 are the most cost effective as they
yield the least cost. This finding suggests that
operating the studied system under any of these
design settings would be far more economically
attractive than operating the same system under
other design settings even when they are also
identified as the most robust designs. For example,
the difference between the most cost-effective
design (configuration design #3) and the most
expensive one (design #13) represents
approximately 56 monetary units in normalized
values. This may represent a significant amount of
money if the value of the monetary loss coefficient
factor is important. Using for example $10.00 value
for the loss coefficient will lead to a difference of
$123.00 in expected losses between design # 3 and
design # 13 representing
38
(1 ) *100 76%
161
of
savings when operating under design # 3 setting
parameters. Design #13 has been used for the
comparison because it is among the strongest design
candidates in terms of robustness of the system (i.e.,
insensitivity to noise factors). This example shows
that significant savings (e.g. 76%) can be generated
when switching ample from design #13 to design #3.
6 CONCLUSIONS
This stud first uses an empirical optimization
procedure to avoid the controversial Taguchi
statistical tools. Then a metamodel is derived from
the simulation outputs. The study also derives a
multivariate quadratic loss function (QLF) from the
traditional Taguchi loss function in order to capture
the loss incurred to the overall system when
attempting to optimize a set of two objective
performances (throughput rate TR and mean flow
time MFT). Hence, the QLF is referred in this study
to as a bivariate quadratic loss function (BQLF).
Table 5: Normalized input data and predicted loss.
Design
Configuration
TR
TR log
(Var)
MFT
MFT
log
(Var)
Pred.
Loss
e.g. K=
$10.00
Norm Norm Norm Norm L(i)
Loss in
$
Design 1 0.056 -0.001 0.034 0.008 21.158 212
Design 2 0.020 0.845 0.009 0.032 59.481 594
Design 3 0.068 -0.007 0.079 0.075 3.792 38
Design 4 0.018 -0.212 0.012 0.007 57.632 576
Design 5 0.056 -0.002 0.036 0.010 18.947 189
Design 6 0.032 -0.097 0.030 0.013 54.482 545
Design 7 0.068 -0.007 0.079 0.075 3.792 38
Design 8 0.034 -0.795 0.021 0.046 53.631 536
Design 9 0.035 -0.299 0.020 0.051 52.277 522
Design 10 0.065 -0.004 0.095 0.114 6.142 61
Design 11 0.019 0.477 0.011 0.010 58.599 586
Design 12 0.033 1.429 0.023 0.026 54.035 540
Design 13 0.057 -0.002 0.087 0.088 16.118 161
Design 14 0.049 -0.001 0.026 0.006 31.707 317
Design 15 0.066 -0.005 0.081 0.076 4.958 50
Des 16 0.068 -0.007 0.079 0.075 3.792 38
Design 17 0.058 -0.002 0.087 0.088 15.599 156
Design 18 0.049 -0.001 0.026 0.006 31.137 311
Design 19 0.019 -0.295 0.009 0.043 59.900 599
Design 20 0.068 -0.007 0.079 0.075 3.792 38
Design 21 0.068 -0.007 0.079 0.075 3.792 38
Next (second level of the optimization scheme), the
BQLF is analytically applied to the metamodel
derived from the simulation outputs to fine-tune the
ptimization process em with respect to the two
objective performances. From the results obtained in
step 1 of the optimization scheme as developed in
this paper, optimum/target values of 100 parts/day
and 0.3666 units time/part (in coded data) have been
fixed for the TR, and MFT, respectively.This two-
level optimization procedure lead to a solution that
yield a minimum cost to be incurred to the overall
system as a penalty for missing the objective targets.
The values of 98 parts/day (-2% from target) and
0.3459 units time/part (+5.6% from target) are
obtained as optima, for TR and MFT, respectively.
These maximum outputs will be otained under a
overal system configuration that is considered to be
the most robust and economical, leading to the
following settings in natural values: Number of
AGVs (X
1
): 6; Speed of AGV (X
2
): 150 feet/min;
Queue discipline (machine rule) (X
3
): SPT; AGV
dispatching rule (X
4
): STD; Buffer size: (X
5
): 4.
Although, conceptually validated on a flexible
manufacturing system, the above-developed and
proposed optimization scheme can be easily
extended to other process-oriented industries
including banks, warehouse, ticketing lines at
airports, restaurants, healthcare facilities,
phamaceutical industries, and others.
ICINCO2013-10thInternationalConferenceonInformaticsinControl,AutomationandRobotics
564