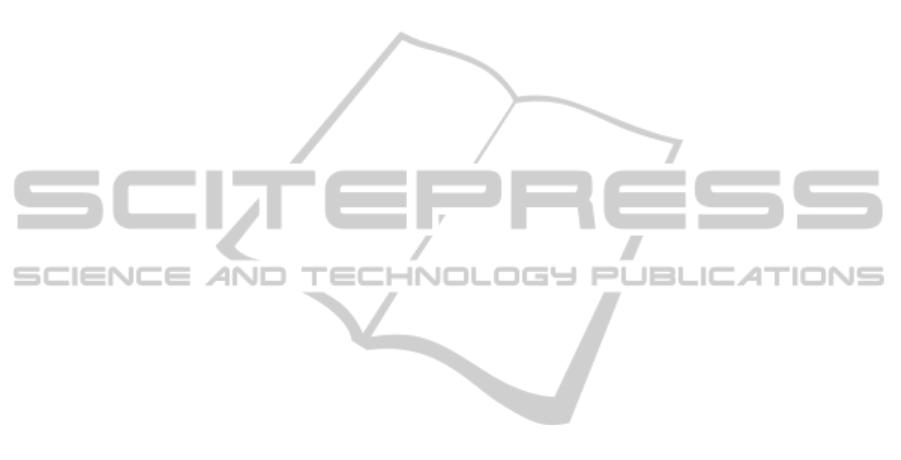
to be optimized in parallel. The objectives have differ-
ent levels of importance that are defined by the user.
A new relation model called Prio-ε-Preferred for
incorporating user preferences in many-objective op-
timization has been presented and experimentally
evaluated. It was shown, that high quality results
could be obtained, i.e. the standard method NSGA-
II was improved by more than 80%.
Furthermore, a new automatic technique for deter-
mining the parameters for relation Prio-ε-Preferred
automatically has been developed. In this context, the
epsilon values have been reduced dynamically during
the optimization run. Experiments showed that best
quality results could be obtained while adjusting the
parameters automatically.
REFERENCES
Auger, A., Bader, J., Brockhoff, D., and Zitzler, E. (2009).
Articulating user preferences in many-objective prob-
lems by sampling the weighted hypervolume. In
Genetic and Evolutionary Computation Conference,
pages 555–562.
Bader, J. and Zitzler, E. (2011). HypE: An algorithm for
fast hypervolume-based many-objective optimization.
Evolutionary Computation, 19(1):45–76.
Benchmarks (2012). Employee scheduling benchmark data
set: http://www.cs.nott.ac.uk/ tec/nrp/. Technical re-
port, ASAP, School of Computer Science, The Uni-
versity of Nottingham, UK.
Brockhoff, D. and Zitzler, E. (2009). Objective reduction in
evolutionary multiobjective optimization: Theorie and
applications. Evolutionary Computation, 17(2):135–
166.
Burke, E., Causmaecker, P. D., Berghe, G., and Landeghem,
H. (2004). The state of the art of nurse rostering. Jour-
nal of Scheduling, 7:441–499.
Burke, E., Curtois, T., Qu, R., and Vanden-Berghe, G.
(2012). Problem model for nurse rostering benchmark
instances. Technical report, ASAP, School of Com-
puter Science, University of Nottingham, UK.
Corne, D. and Knowles, J. (2007). Techniques for highly
multiobjective optimization: Theorie and applica-
tions. In Genetic and Evolutionary Computation Con-
ference, pages 773–780.
Deb, K. (2001). Multi-objective Optimization using Evolu-
tionary Algorithms. John Wiley and Sons, New York.
Deb, K., Thiele, L., Laumanns, M., and Zitzler, E.
(2005). Scalable test problems for evolutionary multi-
objective optimization. In In Evolutionary Multiob-
jective Optimization: Theoretical Advances and Ap-
plications, pages 105–145.
di Pierro, F., Khu, S., and Savic, D. (2007). An investi-
gation on preference order ranking scheme for multi-
objective optimization. IEEE Trans. on Evolutionary
Comp., 11(1):17–45.
Drechsler, N., Drechsler, R., and Becker, B. (2001). Multi-
objective optimisation based on relation favour. In
Int’l Conference on Evolutionary Multi-Criterion Op-
timization, pages 154–166.
Fleming, P., Purshouse, R., and Lygoe, R. (2005). Many-
objective optimization: An engineering design per-
spective. In International Conference on Evolutionary
Multi-Criterion Optimization, pages 14–32.
Fonseca, C. and Fleming, P. (1995). An overview of evo-
lutionary algorithms in multiobjective optimization.
Evolutionary Computation, 3(1):1–16.
Geiger, M. (2009). Multi-criteria curriculum-based course
timetabling - a comparison of a weighted sum and a
reference point based approach. In International Con-
ference on Evolutionary Multi-Criterion Optimiza-
tion, pages 290–304.
Goldberg, D. (1989). Genetic Algorithms in Search, Opti-
mization & Machine Learning. Addison-Wesley Pub-
lisher Company, Inc.
Hughes, E. (2007). Radar waveform optimization as a
many-objective application benchmark. In Interna-
tional Conference on Evolutionary Multi-Criterion
Optimization, pages 700–714.
Ishibuchi, H., Tsukamoto, N., and Nojima, Y. (2008). Evo-
lutionary many-objective optimization: A short re-
view. In IEEE Congress on Evolutionary Computa-
tion, pages 2424–2431.
Koza, J. (1992). Genetic Programming - On the Program-
ming of Computers by means of Natural Selection.
MIT Press.
Li, X. and Wong, H. (2009). Logic optimality for multi-
objective optimization. Applied Mathematics and
Computation, 215:3045–3056.
Pizzuti, C. (2012). A multiobjective genetic algorithm to
find communities in complex networks. IEEE Trans.
on Evolutionary Comp., 16(3):418–430.
Schmiedle, F., Drechsler, N., Große, D., and Drechsler, R.
(2001). Priorities in multi-objective optimization for
genetic programming. In Genetic and Evolutionary
Computation Conference, pages 129–136.
S
¨
ulflow, A., Drechsler, N., and Drechsler, R. (2007). Ro-
bust multi-objective optimization in high-dimensional
spaces. In International Conference on Evolutionary
Multi-Criterion Optimization, pages 715–726.
Wagner, T. and Trautmann, H. (2012). Integration of pref-
erences in hypervolume-based multiobjective evolu-
tionary algorithms by means of desirability functions.
IEEE Trans. on Evolutionary Comp., 14(5):688–701.
Wickramasinghe, U. and Li, X. (2009). A distance met-
ric for evolutionary many-objective optimization algo-
rithms using user-preferences. In 22nd Australasian
Joint Conference on Advances in Artificial Intelli-
gence (AI’09), pages 443–453.
Zitzler, E. and Thiele, L. (1999). Multiobjective evolu-
tionary algorithms: A comparative case study and the
strength pareto approach. IEEE Trans. on Evolution-
ary Comp., 3(4):257–271.
IJCCI2013-InternationalJointConferenceonComputationalIntelligence
74