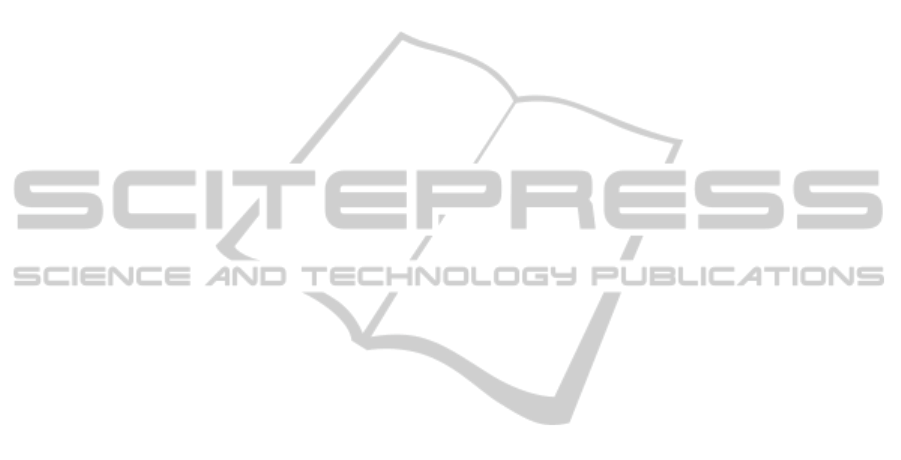
can be practically applied to further domains of
study (or lines of inquiry), providing a flexibly
method for mining new insight from readily
available data.
ACKNOWLEDGEMENTS
The authors acknowledge support of this work
through the MATCH Programme (UK Engineering
and Physical Sciences Research Council grants
numbers GR/S29874/01, EP/F063822/1 and
EP/G012393/1).
REFERENCES
Björkman, I. and Kock, S. (1995) "Social relationships
and business networks: The case of western companies
in China", International Business Review, vol. 4, no. 4,
pp. 519-535.
Buehrer, R. E., Senecal, S. and Bolman Pullins, E. (2005)
"Sales force technology usage—reasons, barriers, and
support: An exploratory investigation", Industrial
Marketing Management, vol. 34, no. 4, pp. 389-398.
Copp, C. B. and Ivy, R. L. (2002) "Networking Trends of
Small Tourism Businesses in PostSocialist
Slovakia", Journal of Small Business Management,
vol. 39, no. 4, pp. 345-353.
De Longueville, B., Smith, R. S. and Luraschi, G. (2009)
"Omg, from here, i can see the flames!: a use case of
mining location based social networks to acquire
spatio-temporal data on forest fires", Proceedings of
the 2009 International Workshop on Location Based
Social NetworksACM, , pp. 73.
Glaser, B. G. and Strauss, A. L. (1967) The discovery of
grounded theory: Strategies for qualitative research,
AldineTransaction.
Huberman, B., Romero, D. and Wu, F. (2008) "Social
networks that matter: Twitter under the microscope",
Available at SSRN 1313405, .
Hughes, A. L. and Palen, L. (2009) "Twitter adoption and
use in mass convergence and emergency events",
International Journal of Emergency Management, vol.
6, no. 3, pp. 248-260.
Java, A., Song, X., Finin, T. and Tseng, B. (2009) "Why
we twitter: An analysis of a microblogging
community", Advances in Web Mining and Web
Usage Analysis, , pp. 118-138.
Karpf, D. (2012) "Social Science Research Methods in
Internet Time", Information, Communication &
Society, vol. 15, no. 5, pp. 639-661.
Kucuktunc, O., Cambazoglu, B.B., Weber, I. and
Ferhatosmanoglu, H. (2012) "A large-scale sentiment
analysis for Yahoo! answers", Proceedings of the fifth
ACM international conference on Web search and
data miningACM, , pp. 633.
Lauw, H. W., Lim, E.P., Pang, H. and Tan, T. T. (2005)
"Social network discovery by mining spatio-temporal
events", Computational & Mathematical Organization
Theory, vol. 11, no. 2, pp. 97-118.
Lee, C. H., Yang, H.C., Chien, T.F. and Wen, W.S. (2011)
"A Novel Approach for Event Detection by Mining
Spatio-temporal Information on Microblogs",
Advances in Social Networks Analysis and Mining
(ASONAM), 2011 International Conference onIEEE, ,
pp. 254.
Lipparini, A. and Sobrero, M. (1994) "The glue and the
pieces: entrepreneurship and innovation in small-firm
networks", Journal of Business Venturing, vol. 9, no.
2, pp. 125-140.
Masurel, E. and Janszen, R. (1998) "The relationship
between SME cooperation and market concentration:
Evidence from small retailers in the Netherlands",
Journal of Small Business Management, vol. 36, pp.
68-73.
Michaelidou, N., Siamagka, N. T. and Christodoulides, G.
(2011) "Usage, barriers and measurement of social
media marketing: An exploratory investigation of
small and medium B2B brands", Industrial Marketing
Management.
Pak, A. and Paroubek, P. (2010) "Twitter as a corpus for
sentiment analysis and opinion mining", Proceedings
of LREC.
Papacharissi, Z. (2009) "The virtual geographies of social
networks: a comparative analysis of Facebook,
LinkedIn and ASmallWorld", New Media & Society,
vol. 11, no. 1-2, pp. 199-220.
Pitt, L., van der Merwe, R., Berthon, P., Salehi-Sangari, E.
and Caruana, A. (2006) "Global alliance networks: A
comparison of biotech SMEs in Sweden and
Australia", Industrial Marketing Management, vol. 35,
no. 5, pp. 600-610.
Shirzad, S. R., Bell D. (2013) "A Systematic Literature
Review of Flexible E-Procurement Marketplace", .
Skeels, M. M. and Grudin, J. (2009) "When social
networks cross boundaries: a case study of workplace
use of facebook and linkedin", Proceedings of the
ACM 2009 international conference on Supporting
group workACM, , pp. 95.
Thelwall, M., Buckley, K., Paltoglou, G., Cai, D. and
Kappas, A. (2010) "Sentiment strength detection in
short informal text", Journal of the American Society
for Information Science and Technology, vol. 61, no.
12, pp. 2544-2558.
Venkatesh, V. and Davis, F. D. (2000) "A theoretical
extension of the technology acceptance model: Four
longitudinal field studies", Management science, vol.
46, no. 2, pp. 186-204.
Walter, A., Ritter, T., Germunden, H. and Gemunden, H.
(1997) Relationships and networks in international
markets, Pergamon Press.
Yoo, J. and Hwang, J. (2008) "A framework for
discovering spatio-temporal cohesive networks",
Advances in Knowledge Discovery and Data Mining, ,
pp. 1056-1061.
WEBIST2013-9thInternationalConferenceonWebInformationSystemsandTechnologies
570