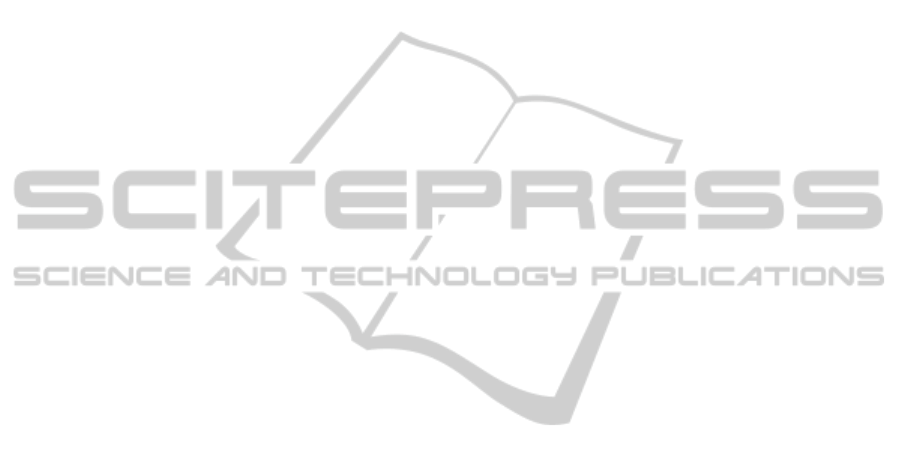
tics this metric is more inaccurate than the IRT metric
presented in this paper. By relying on three coeffi-
cients we can further refine relationships and besides
identifying equivalence and hierarchical relations also
identify associative relations between the types of two
taxonomies which can not be done with metrics pro-
posed so far.
Our instance enrichment approach is crucial since
it allows us to apply instance-based matching tech-
niques in the first place. Closest to that idea is the
QuickMig system (Drumm et al., 2007) where in-
stances have to be provided manually in a question-
naire. None of the existing systems is able to gener-
ate instances beforehand to apply instance matching
as we do in this paper. Moreover, we are the first to
apply ontology matching techniques for matching text
mining taxonomies.
8 CONCLUSIONS AND FUTURE
WORK
In this paper we presented a number of contributions
that help to automatically match and integrate tax-
onomies of text mining services and therewith en-
able the combination of several text mining services.
In particular we developed an instance enrichment
algorithm that allows us to apply instance match-
ing techniques in a complex matching strategy. We
proposed a general taxonomy alignment process that
applies a new instance-based matcher using a novel
metric called IRT. This metric allows us to derive
equality, hierarchical and associative mappings. Our
evaluation results are promising, showing that the
instance enrichment and matching approach returns
good quality mappings and outperforms traditional
metrics. Furthermore, the matching process again in-
dicated that the results of different text mining ser-
vices are very different, i.e., the instances of semanti-
cally identical taxonomy types are only partly over-
lapping (partly only 5% of the instances overlap).
This emphasizes the results from Seidler and Schill
(2011) that the quality and quantity of text mining can
be increased through the aggregation of text mining
results from different services. The presented taxon-
omy alignment process will allow us in future to au-
tomate the matching of text mining taxonomies and
subsequently the automatic merging of text mining re-
sults from different services.
REFERENCES
AlchemyAPI (2013). AlchemyAPI Homepage. http://
www.alchemyapi.com/. March 2013.
Chua, W. W. K. and Kim, J.-J. (2012). Discovering Cross-
Ontology Subsumption Relationships by Using On-
tological Annotations on Biomedical Literature. In
ICBO, volume 897 of CEUR Workshop Proc.
Do, H. H. and Rahm, E. (2002). COMA - A System for
Flexible Combination of Schema Matching Approach.
In VLDB Proc.
Drumm, C., Schmitt, M., Do, H.-H., and Rahm, E. (2007).
QuickMig: Automatic Schema Matching for Data Mi-
gration Projects. In CIKM’07 Proc.
Euzenat, J. and Shvaiko, P. (2007). Ontology Matching.
Springer-Verlag.
Evri (2012). Evri Developer Homepage. http://
www.evri.com/developer/. June 2012.
FISE (2013). Furtwangen IKS Semantic Engine project
page. http://wiki.iks-project.eu/index.php/FISE.
March 2013.
Grimes, S. (2008). Unstructured data and the 80 percent
rule. http://breakthroughanalysis.com/2008/08/01/
unstructured-data-and-the-80-percent-rule/.
Clarabridge Bridgepoints.
Hotho, A., N
¨
urnberger, A., and Paaß, G. (2005). A Brief
Survey of Text Mining. LDV Forum, 20(1):19–62.
Hu, W. and Qu, Y. (2008). Falcon-AO: A practical Ontology
Matching System. Web Semantics, 6(3):237–239.
Isaac, A., Van Der Meij, L., Schlobach, S., and Wang, S.
(2007). An Empirical Study of Instance-Based Ontol-
ogy Matching. In ISWC’07 Proc., pages 253–266.
Jean-Mary, Y. R., Shironoshita, E. P., and Kabuka, M. R.
(2009). Ontology Matching with Semantic Verifica-
tion. Web Semantics, 7(3):235–251.
Li, J., Tang, J., Li, Y., and Luo, Q. (2009). RiMOM: A Dy-
namic Multistrategy Ontology Alignment Framework.
TKDE, 21(8):1218–1232.
Massmann, S. and Rahm, E. (2008). Evaluating Instance-
based Matching of Web Directories. In WebDB’08
Proc.
OpenCalais (2013). Calais Homepage. http://
www.opencalais.com/. March 2013.
Rahm, E. and Bernstein, P. A. (2001). A Survey of Ap-
proaches to Automatic Schema Matching. The VLDB
Journal, 10:334–350.
Seidler, K. and Schill, A. (2011). Service-oriented Infor-
mation Extraction. In Joint EDBT/ICDT Ph.D. Work-
shop’11 Proc., pages 25–31.
Shvaiko, P. and Euzenat, J. (2005). A Survey of Schema-
Based Matching Approaches. Journal on Data Se-
mantics IV.
Suchanek, F. M., Abiteboul, S., and Senellart, P. (2011).
Paris: probabilistic alignment of relations, instances,
and schema. Proc. VLDB Endow., 5(3):157–168.
KDIR2013-InternationalConferenceonKnowledgeDiscoveryandInformationRetrieval
16