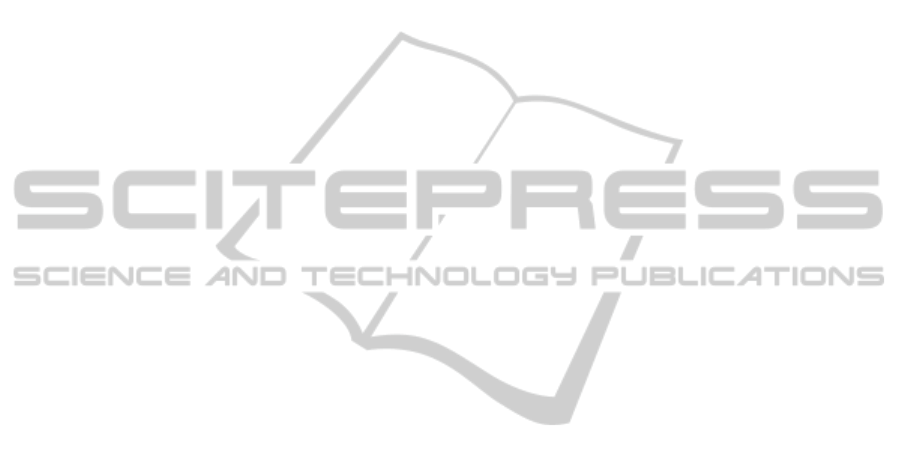
6 CONCLUSIONS
We develop a binary logit model with a Bayesian
mixture approach to model consumer clickstreams
across multiple types of online advertising and ana-
lyze the individual conversion probabilities of con-
sumers. The mixture approach we utilize outperforms
the standard normal approach and is useful for con-
sidering heterogeneity in the individual propensity of
consumers to purchase; for the majority of consumers
(more than 90%), repeated clicks on advertisements
decrease their probability of purchasing. Thus, for
this segment of consumers, the probability of pur-
chasing is highest after consumers’ first click on an
advertisement. In contrast with this segment, we find
a smaller segment of consumers (nearly 10%) whose
clicks on advertisements increase conversion proba-
bilities.
We successfully demonstrate how to simultane-
ously integrate and evaluate multiple types of online
advertising to gain knowledge that is indispensable to
allocating financial resources. The evaluation of con-
sumers on an individual level along their complete
user-journeyis essential to optimize the auction-based
process, particularly in the emerging new advertising
technology of real-time bidding.
Furthermore, we are able to show inherent differ-
ences in the effects of consumer clicks on purchas-
ing probabilities across multiple advertising channels.
Therefore, on the basis of our parameter estimates, we
develop an alternative approach of accounting for the
success of advertising channels—the average success
probability (ASP)—which may be interpreted as an
advertisement- and campaign-specific contribution to
companies’ success probabilities as we take into ac-
count both short- and long-term advertising effects of
the complete investigation period. Compared to stan-
dardized advertisement-specific conversion rates, we
find the “search” advertising channel to be overesti-
mated and the “retargeting” channel to be underesti-
mated.
In this paper, we analyze a large dataset contain-
ing detailed individual consumer-level information.
Tracking individual consumers across multiple online
advertising types is accomplished by the application
of cookies that are stored on the personal computer of
each consumer. Thus, we do not have combined in-
formation regarding consumer usage of web browsers
across multiple devices (i.e., personal computers at
work versus at home) and are thus unable to model
complete sessions for all consumers. Furthermore,
modern web browsers give consumers the opportu-
nity to deny websites access their personal computers
to store cookies.
Another limitation of this work is that we do not have
any information on consumers’ isolated exposures to
online advertisements that have not been clicked. The
long-term effects of unclicked online advertisements
are thought to be positive on conversions (Yoon and
Lee, 2007) but have not been analyzed yet on an indi-
vidual user-level while analyzing multiple online ad-
vertising channels. We leave this question open for
future research.
Further, there is room for research analyzing the
effects of specific online advertisements on consumer
online behavior. For example, the integration of
consumer-specific information, such as gender or
interests, might uncover further insights into con-
sumers’ individual online click and conversion prob-
abilities. We also see the combination of aggregated
data (that does not suffer from the cookie-deleting
problem) with consumer-level data (as it is analyzed
here) as an important and interesting topic for future
research.
REFERENCES
Abhishek, V., Hosanagar, K., and Fader, P. S. (2011). On
aggregation bias in sponsored search data: Existence
and implications. http://ssrn.com/abstract=1490169.
Allenby, G. M. and Ginter, J. L. (1995). Using extremes
to design products and segment markets. Journal of
Marketing Research, 32(4):392–403.
Ansari, A., Mela, C. F., and Neslin, S. A. (2008). Customer
channel migration. Journal of Marketing Research,
45(1):60–76.
Athey, S. and Ellison, G. (2009). Position auctions with
consumer search. http://ssrn.com/abstract=1454986.
Bass, F. M., Bruce, N., Majumdar, S., and Murthi, B.
P. S. (2007). Wearout effects of different adver-
tising themes: A dynamic bayesian model of the
advertising-sales relationship. Marketing Science,
26(2):179–195.
Broder, A. (2002). A taxonomy of web search. SIGIR Fo-
rum, 36(2):3–10.
Chatterjee, P., Hoffman, D. L., and Novak, T. P. (2003).
Modeling the clickstream: Implications for web-based
advertising efforts. Marketing Science, 22(4):520–
541.
Danaher, P. J. and Mullarkey, G. (2003). Factors affecting
online advertising recall: A study of students. Journal
of Advertising Research, 43(3):252–267.
Deleersnyder, B., Geyskens, I., Gielens, K., and Dekimpe,
M. G. (2002). How cannibalistic is the internet chan-
nel? a study of the newspaper industry in the united
kingdom and the netherlands. International Journal
of Research in Marketing, 19(4):337–348.
Dinner, I. M., van Heerde, H. J., and Neslin, S. A.
(2011). Driving online and offline sales: The cross-
channel effects of digital versus traditional advertis-
ing. http://ssrn.com/ abstract=1955653.
WhichClicksLeadtoConversions?-ModelingUser-journeysAcrossMultipleTypesofOnlineAdvertising
151