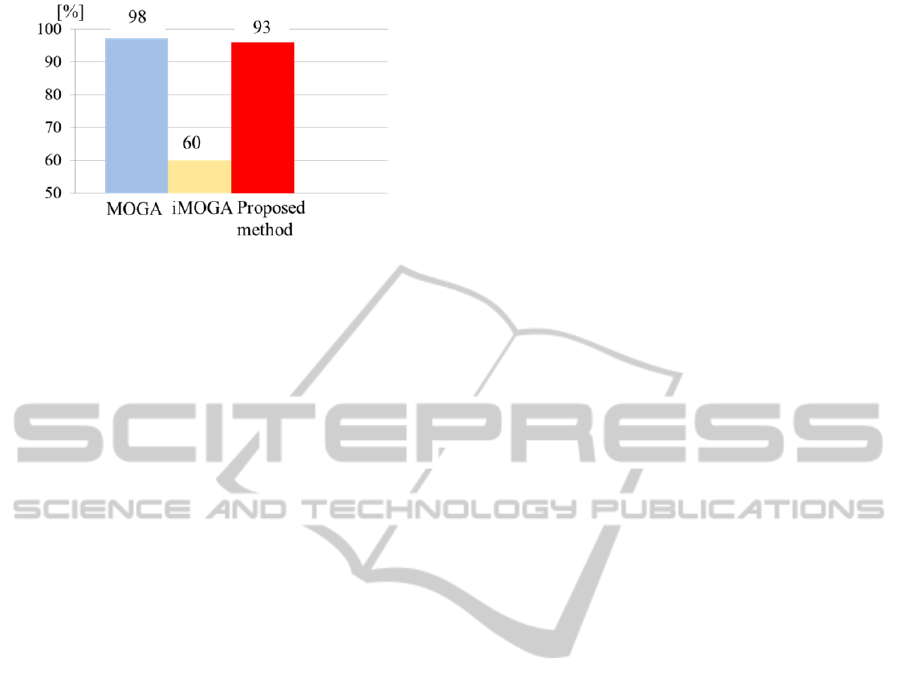
Figure 11: The rate of the successful search.
iMOGA can be executed in 9.3 seconds, and the
proposed method can be executed in 10 seconds.
Response time of proposed method is a little bit
longer than that of iMOGA because of selecting the
solution based on the amount of the additional
orders. But the increase of the computational time is
trivial for the decision-makers.
Fig. 10 shows the result of the interaction by 250
kinds of the agent. The proposed method can search
the preference solutions for the half of the agents
with 3 interactions. The more preference solutions
are searched up to 7 interactions. Finally, the
proposed method can not search 7% of the
preference solutions within 10 times of the
interactions. iMOGA can search the preference
solutions for 30% of the agents with 3 interactions
and also search more solutions up to 7 interactions.
iMOGA can not search 40% of the preference
solutions within 10 times of the interactions. As
shown in Fig. 10, the average of the interactions by
the proposed method is 3.9 and one by iMOGA is
6.3. So, the proposed method can succeed in
decreasing the number of the interactions by 38%
compared to iMOGA.
Focusing the rate of the successful searches
shown in Fig. 11, MOGA can search the preference
solutions for 98% of the agents within 10
interactions. The proposed method can search the
preference solutions for 93% of the agents within 10
interactions. This indicates that the proposed method
is as available as MOGA from the viewpoint of the
accuracy of displaying the preference solutions.
Because iMOGA selects solutions for next MOGA
only from a selected cluster, iMOGA often fails to
search the preference solutions within 10
interactions.
From the experimental result, it is confirmed that
it is possible to search more preference solutions by
the proposed method with fewer interactions
compared to iMOGA. And the representative
solutions are displayed within 10 seconds, which is
short enough to support the discussion.
5 CONCLUSIONS
In this paper, we proposed an interactive evaluation
method of decentralized procurement plan by Multi-
objective genetic algorithm (MOGA). Although
MOGA has been applied to the multi-objective
optimization problem, the decision-maker can not
understand many Pareto optimal solutions by
MOGA. And, MOGA takes several minutes to solve
the multi-objective optimization problem. Our
proposed method displays the representative
solutions to the decision-makers and has them to
select one. By repeating this interaction, the
decision-maker can search their preference solutions
in a short time. In order to evaluate the loss that is
caused probabilistic catastrophic disaster, we
developed the loss evaluation simulator. This
simulator can evaluate the loss by simulating the
stock with Monte Carlo method. Furthermore, in
order to decrease the interaction, the proposed
method selects not only the solutions that are in the
selected cluster but also the solutions that tend to
generate the preference solutions based on the
amount of the additional orders. Through the
application of the proposed method to the supply
chain model, we confirmed that the proposed
method decreased the 38% of the interactions of
selecting solutions compared to iMOGA.
Our future work is to expand the versatility of
the proposed method to apply other supply chain
model. It will be necessary to analyse the sensitivity
of the parameters, especially the number of suppliers
and additional procurement rates. For practical use
of this method, we will implement the graphical user
interface to display the representative solutions to
support selecting solutions by the decision-maker.
REFERENCES
Azaron, M. A., Brown, K.N., Tarim, S.A., Modarres M.,
2008. “A multi-objective stochastic programming
approach for supply chain design considering risk,”
International Journal of Production Economics, Vol.
116, pp. 129-138.
Chopra, S., Sodhi, M. S., 2004. “Managing Risk to Avoid
Supply-Chain Breakdown,” MIT Sloan Management
Review, Vol. 46, No. 1 pp.53-61.
Fonseca C. M., Fleming, P. J., 1993. “Genetic Algorithms
for Multiobjective Optimization: Formulation,
Discussion and Generalization,” In Proceedings of the
5
th
International Conference on Genetic Algorithms,
pp. 416-423.
Hearst, M. A., 2006. “Clustering versus faceted categories
for information exploration,” Communications of the
AnInteractiveEvaluationMethodofDecentralizedProcurementPlanbyMulti-ObjectiveGeneticAlgorithm
367