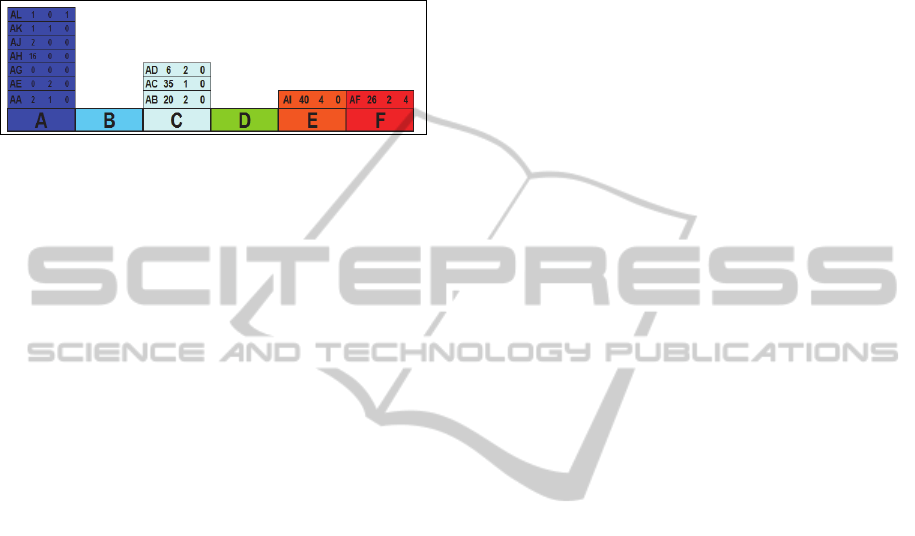
analyzing values such as, for example, classification
of AI and AF feeders, as C5 and C7 values in AI
area higher than in AF, which could indicate a lower
quality in AI compared to AF but, as C8 has a lower
value for AI, the techniques applied indicated that
AF has lower quality than the AI feeder.
Figure 10: Quality label with feeder classification.
Thus, the methodology developed and applied in
this study revealed non-explicit knowledge in the
concessionaire’s data bases to an unprecedented real
problem: the PQ considering voltage sags.
ACKNOWLEDGEMENTS
This study is an integral part of the P & D project
number 2866-019/2007 – Event Classification for
Power Quality, approved by ANEEL and developed
in partnership with COPEL and the UFPR.
REFERENCES
Adepoju, G. A.; Ogunjuyigbe, S. O. A.; Alawode, K. O.
Application of Neural Network to Load Forecasting in
Nigerian Electrical Power System. The Pacific
JouANNl of Science and Technology. Spring. v. 8, p.
68-72, 2007.
ANEEL Agência Nacional de Energia Elétrica.
Procedimentos de Distribuição de Energia Elétrica no
Sistema Elétrico Nacional – PRODIST: Módulo 8 –
Qualidade da Energia Elétrica. 2008.
Burges, C. J. C. A Tutorial on Support Vector Machines
for Pattern Recognition. Data Mining and Knowledge
Discovery, v. 2, p. 121-168, 1998.
Caciotta, M.; Giarnetti, S.; Leccese, F. Hybrid Neural
Network System for Electric Load Forecasting of
Telecomunication Station. XIX IMEKO World
Congress - Fundamental and Applied Metrology.
Lisboa, Portugal, p. 657-661, 2009.
Casteren, J. F. L. Van.; Enslin, L. H. R.; Hulshorst, W. T.
J.; Kilng, W.L.; Hamoen, M. D.; Cobben, J. F. G.
Acustomer oriented approach to the classification of
voltage dips. In: The18th International Conference
and exhibition on Electricity Distribuion – CIRED,
2005.
Cobben, J. F. G.; Casteren, J. F. L. Classification
Methodologies for Power Quality. Electrical Power
Quality & Utilization Magazine. v. 2, no 1, p. 11-17,
2006.
Dash, P. K.; Padhee, M.; Barik, S. K. Estimation of power
quality indices in distributed generation systems
during power islanding conditions. Electrical Power
and Energy Systems, v. 36, p. 18-30, 2012.
Fayyad, U.; Piatetsky-Shapiro, G.; Smyth, P.;
Uthurusamy, R. Advances in Knowledge Discovery &
Data Mining. 1 ed. American Association for Artificial
Intelligence, Menlo Park, Califórnia, 1996.
Gencer, O.; Ozturk, S.; Erfidan, T. A new approach to
voltage sag detection based on wavelet transform.
Electrical Power and Energy Systems, v. 32, p. 133-
140, 2010.
Góes, A. R. T. Uma metodologia para a criação de
etiqueta de qualidade no contexto de Descoberta de
Conhecimento em Bases de Dados: aplicação nas
áreas elétrica e educacional. 145 f. Tese (Doutorado
em Métodos Numéricos em Engenharia) - Setor
Tecnologia e Setor de Ciências Exatas, Universidade
Federal do Paraná, Curitiba, 2012.
Goldberg, D. E. Genetic algorithms in search, optmization,
and machines learning. Addison-Wesley Publishing
Company, Inc. Massachusetts, 1989.
Haykin, S. Neural Networks – A Comprehensive
Foundation. 2.nd., Prentice Hall, New Jersey, 1999.
Kaewarsa, S.; Attakitmongcol, K.;
Kulworawanichpong, T. Recognition of power quality
events by using multiwavelet-based neural networks.
Electrical Power and Energy Systems, v. 30, p. 245-
260, 2008.
Kappor, R.; Saini, M. K. Hybrid demodulation concept
and harmonic analysis for single/multiple power
quality events detection and classification. Electrical
Power and Energy Systems, v. 33, p. 1608-1622, 2011.
Oleskovicz, M.; Coury, D. V.; Carneiro, A. A. F. M.;
Arruda, E. F.; Delmont, O.; Souza, S. A. Estudo
comparativo de ferramentas modeANNs de análise
aplicadas à qualidade da energia elétrica. Revista
Controle & Automação, v. 17, n. 3. Julho, agosto e
setembro 2006.
Trindade, R. M. Sistema Digital de Detecção e
Classification de Eventos de Qualidade de Energia.
114 f. Dissertação (Mestrado em Engenharia Elétrica)
– Faculdade de Engenharia da Universidade Federal
de Juiz de Fora, Juiz de Fora, 2005.
Vapnik, V. The nature of statistical learning theory.
Springer-Verlag, New Yourk, 1995.
IJCCI2013-InternationalJointConferenceonComputationalIntelligence
442