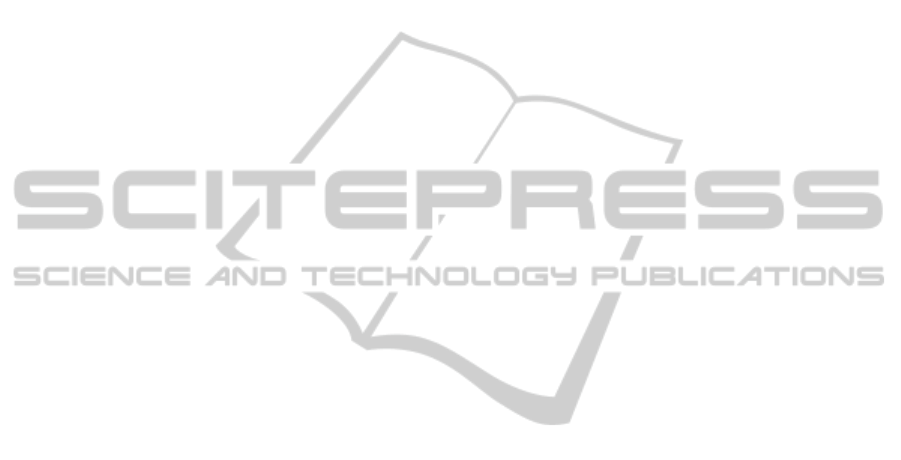
response obtained showed an error of 0.07108 to an
ANN with 23 neurons in the input layer, six neurons
in the hidden layer, with 50 iterations (Table 5.2).
The MLR was performed using
STATIGRAPHICS Plus 5.1 Software. In tests with
this tool, the data sets used (training and testing)
were the same of the ANNs, in order to obtain
comparative parameters between the two
mathematical tools. The error obtained was equal to
0.0743 for the training set and 0.1287 for the test set.
The Decision Tree technique was applied via
WEKA software (Waikato Environment for
Knowledge Analysis), which is free and has an open
code source, used for data mining. We used the same
sets of data in order to compare the applied
techniques. With this tool the error obtained was
0.0881. With this tool the 3rd test also showed the
best result, considering a full MSE equal to 0.0881.
Although the techniques have shown satisfactory
results, the ANNs presented a superior performance
when compared to other methods, as it can observed
through the errors 0.07108 (ANNs), 0.1287 (MLR)
and 0.0881 (Decision Tree).
Thus, the best way to predict the length of the
processing of a new labor lawsuit, is to use ANN
"E23N6I50", Table 5.2, where the weights were
generated by the 3 third test (ordinal attributes
without encoding, and with PCA) . This way, when
it is desired to know the length of proceeding of a
new labor lawsuit, one must determine the principal
components of this case and then, using the topology
and network weights E23N6I50, obtain the required
number of months for this case.
It is worth mentioning that from time to time, in
accordance with the suggestion of specialists of the
area (labor judges), latest data (files) with known
and reliable answers should be included in the
database and methodology should be repeated,
always glimpsing the lowest possible error for that
prediction. With this, it is expected to obtain a more
dynamic and accurate judiciary system, as well as
greater satisfaction of its users.
REFERENCES
Adamowicz, E. C., 2000. Reconhecimento de Padrões na
Análise Econômico–Financeira de Empresas. Curitiba.
Dissertação de Mestrado, PPGMNE, UFPR.
Ambrósio, P. E., 2002 Redes Neurais Artificiais no Apoio
ao Diagnóstico Diferencial de Lesões Intersticiais
Pulmonares. Ribeirão Preto. Dissertação de Mestrado,
USP.
Baesens, B., Setiono, R., Mues, C. & Vanthienen, J., 2003.
Using Neural Network Rule Extraction and Decision
Tables for Credit-Risk Evalution. Management
Science, 49, 3, 312-329.
Baptistella, M., Cunico, L. H. B., Steiner, M. T. A., 2009.
O Uso de Redes Neurais na Engenharia de Avaliações:
Determinação dos Valores Venais de Imóveis
Urbanos. Revista de Ciências Exatas e Naturais, 9, 2,
215-229.
Biondi Neto, L., Sieira, A. C. C. F., Danziger B. R., Silva,
J. G. S., 2006. Neuro-CPT: Classificação de Solos
usando-se Redes Neurais Artificiais. Engevista, v. 8, p.
37-48.
Bond, M. T., Seiler, V. L., Seiler, M. J., 2002. Residencial
Real Estate Prices: a Room with a View. The Journal
of Real Estate Research, v. 23, n. 1, p. 129-137.
Haykin, S., 2002. Redes Neurais: Princípios e Prática.
Bookman, Porto Alegre, RS.
Lima, J. D., 2002. Análise Econômico–Financeira de
Empresa Sob a Ótica da Estatística Multivariada.
Curitiba. Dissertação de Mestrado, PPGMNE, UFPR.
Lu, H.; Setiono, R. & Liu, H., 1996. Effective Data
Mining Using Neural Networks. IEE Transactions on
Knowledge an Data Engineering, 8, 6, 957-961.
Nguyen, N., Cripps, A. 2001. Predicting Housing Value:
A Comparison of Multiple Regression Analysis and
Artificial Neural Networks. The Journal of Real Estate
Research, v. 22, n. 3, p. 313-336.
SLC - Superior Labor Court. (http://www.tst.gov.
br/) 16 february 2007.
Sousa, E. A., Teixeira, L. C. V., Mello, M. R. P. A.,
Torres, E. A. F. S., Moita Neto, J. M., 2003. Aplicação
de Redes Neurais para Avaliação do Teor de Carne
Mecanicamente Separada em Salsicha de Frango.
Ciência e Tecnologia de Alimentos, 23, 3, Campinas.
Steiner, M. T. A. 1995. Uma Metodologia Para o
Reconhecimento de Padrões Multivariados com
Resposta Dicotômica. Florianópolis. Tese de
Doutorado, Programa de Pós Graduação em
Engenharia de Produção, UFSC.
Steiner, M. T. A., Nievola, J. C., Soma, N. Y., Shimizu,
T., Steiner Neto, P. J., 2007. Extração de regras de
classificação a partir de redes neurais para auxílio à
tomada de decisão na concessão de crédito bancário.
Revista Pesquisa Operacional, 27, 407-426.
Steiner, M. T. A.; Mota, J. F., 2007. Estudando um Caso
de determinação do Preço de Venda de Imóveis
Urbanos utilizando Redes Neurais Artificiais e
Métodos Estatísticos Multivariados. X Encontro de
Modelagem Matemática, Nova Friburgo, RJ.
Steiner, M. T. A., Bráulio, S. N., Alves, V., 2008.
Métodos Estatísticos Multivariados aplicados à
Engenharia de Avaliações, Revista Gestão &
Produção, 15, 23-32.
Steiner, M. T. A., Carnieri, C., Stange, P., 2009.
Construção de um Modelo Matemático para o
Controle do Processo de Produção do Papel Industrial.
Pesquisa Operacional para o Desenvolvimento, 1, 1,
33-49.
TRT - Tribunal Regional do Trabalho. (http://www.trt9.
gov.br/> 07 october 2007.
Witten, I. H., Frank, E., 2005, Data Mining: Practical
Machine Learning Tools and Techniques, Morgan
Kaufmann Publishers, 2nd edition.
IJCCI2013-InternationalJointConferenceonComputationalIntelligence
450