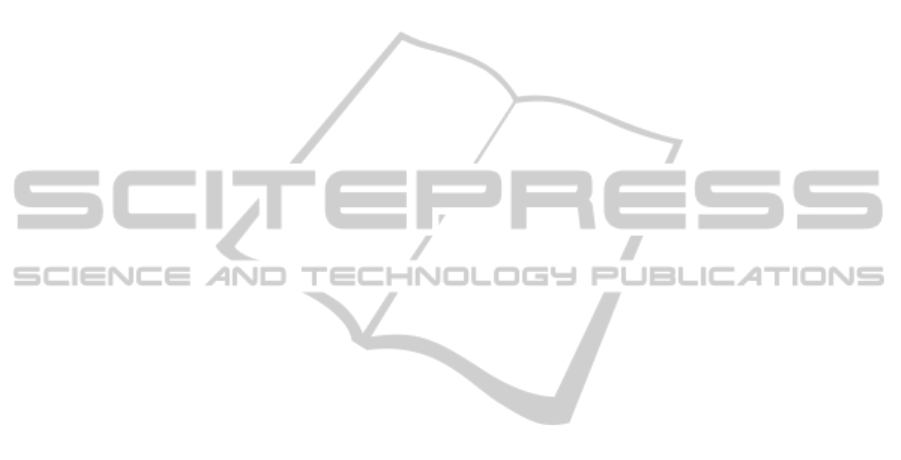
DeConde, R., Hawley, S., Falcon, S., Clegg, N., Knudsen,
B., and Etzioni, R. (2006). Combining results of mi-
croarray experiments: A rank aggregation approach.
Statistical Applications in Genetics Molecular Biol-
ogy, 5(1):1–17.
Dinu, L. P. and Manea, F. (2006). An efficient approach for
the rank aggregation problem. Theor. Comput. Sci.,
359(1):455–461.
Dwork, C., Kumar, R., Naor, M., and Sivakumar, D. (2001).
Rank aggregation methods for the web. pages 613–
622.
Ferri, C., Hern´andez-Orallo, J., and Modroiu, R. (2009).
An experimental comparison of performance mea-
sures for classification. Pattern Recognition Letters,
30(1):27–38.
Golub, T. R., Slonim, D.K., Tamayo, P., Huard, C., Gaasen-
beek, M., Mesirov, J. P., Coller, H., Loh, M. L., Down-
ing, J. R., Caligiuri, M. A., and Bloomfield, C. D.
(1999). Molecular classification of cancer: class dis-
covery and class prediction by gene expression moni-
toring. Science, 286:531–537.
Gotshall, S. and Rylander, B. Optimal population size and
the genetic algorithm.
Guyon, I. and Elisseff, A. (2003). An introduction to vari-
able and feature selection. Journal of Machine Learn-
ing Research, 3:1157–1182.
Hall, M. A. (2000). Correlation-based feature selection for
discrete and numeric class machine learning. In Pro-
ceedings of the Seventeenth International Conference
on Machine Learning, pages 359–366. Morgan Kauf-
mann.
Hastie, T., Tibshirani, R., and Friedman, J. (2001). The
Elements of Statistical Learning. Springer series in
statistics. Springer New York Inc.
Holland, J. H. (1992). Adaptation in natural and artificial
systems. MIT Press, Cambridge, MA, USA.
Kira, K. and Rendell, L. (1992). A practical approach to
feature selection. In Sleeman, D. and Edwards, P., ed-
itors, International Conference on Machine Learning,
pages 368–377.
Kohavi, R. and John, G. H. (1997). Wrappers for feature
subset selection. Artificial Intelligence, 97:273–324.
Kumar, R. and Vassilvitskii, S. (2010). Generalized dis-
tances between rankings. In Proceedings of the 19th
international conference on World wide web, WWW
’10, pages 571–580, New York, NY, USA. ACM.
Okun, O. (2011). Feature Selection and Ensemble Methods
for Bioinformatics: Algorithmic Classification and
Implementations.
Pomeroy, S. L., Tamayo, P., Gaasenbeek, M., Sturla, L. M.,
Angelo, M., McLaughlin, M. E., Kim, J. Y. H., Goum-
nerova, L. C., Black, P. M., Lau, C., Allen, J. C.,
Zagzag, D., Olson, J. M., Curran, T., Wetmore, C.,
Biegel, J. A., Poggio, T., Mukherjee, S., Rifkin, R.,
Califano, A., Stolovitzky, G., Louis, D. N., Mesirov,
J. P., Lander, E. S., and Golub, T. R. (2002). Prediction
of central nervous system embryonal tumour outcome
based on gene expression. Nature, 415(6870):436–
442.
Quinlan, J. R. (1993). C4.5: programs for machine learn-
ing. Morgan Kaufmann Publishers Inc.
Vafaie, H. and Imam, I. (1994). Feature Selection Meth-
ods: Genetic Algorithms vs. Greedy-like Search.
Manuscript.
Weston, J., Elisseeff, A., Schlkopf, B., and Kaelbling, P.
(2003). Use of the zero-norm with linear models and
kernel methods. Journal of Machine Learning Re-
search, 3:1439–1461.
Young, H. P. (1990). Condorcet’s theory of voting. Math-
matiques et Sciences Humaines, 111:45–59.
Young, H. P. and Levenglick, A. (1978”,). A consistent ex-
tension of Condorcet’s election principle. SIAM Jour-
nal on Applied Mathematics, 35(2):285–300.
FeatureSelectionbyRankAggregationandGeneticAlgorithms
81