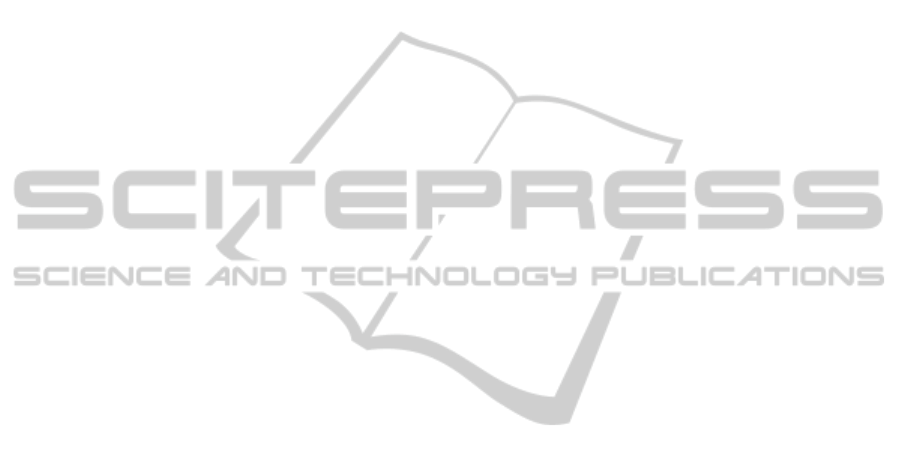
the Reunion Island. The same process can be repli-
cated to compute changes for the others land cover
types. The proposed approach presents a useful tool
for disaster prevention and monitoring, planting sta-
tus of agricultural products, and tree distribution of
forests. The evaluation depicts good results of the
proposed approach in predicting urban changes.
Future prospects are primarily research and ex-
traction of other relevant descriptors and indicators
that affect objects features of satellite images and im-
prove the complexity of our algorithm. Another chal-
lenge is to apply the proposed approach on others
sites.
ACKNOWLEDGEMENTS
There are so many people who have provided me with
their support, and it is not easy to find the right words
to express my gratitude.
REFERENCES
Amor, N. B., Benferhat, S., and Elouedi, Z. (2008). Deci-
sion trees as possibilistic classifiers. In International
Journal of Approximate Reasoning.
Anuradha, K., Radha, N. T., and Kumar, T. P. (2012). A
decision tree algorithm for uncertain data. In Interna-
tional Journal of Engineering and Advanced Technol-
ogy.
Benz, U., Hofmann, P., Willhauck, G., Lingenfelder, I., and
Heynen, I. (2004). Multi-resolution, object-oriented
fuzzy analysis of remote sensing data for gis-ready in-
formation. In ISPRS Journal of Photogrammetry and
Remote Sensing.
Boulila, W., Farah, I., Ettabaa, K., Solaiman, B., and
Ghzala, H. B. (2010). Spatio-temporal modeling for
knowledge discovery in satellite image databases. In
Conference en Recherche d’Information et Applica-
tions.
Boulila, W., Farah, I., Ettabaa, K., Solaiman, B., and
Ghzala, H. B. (2011). A data mining based approach
to predict spatiotemporal changes in satellite images.
In International Journal of Applied Earth Observation
and Geoinformation.
Boulila, W., Farah, I., Ettabaa, K., Solaiman, B., and
Ghzala, H. B. (2012). Combining decision fusion and
uncertainty propagation to improve land cover change
prediction in satellite image databases. In Journal of
Multimedia Processing and Technologies.
Chang, P., Fan, C., and Dzan, W. (2010). A cbr-based fuzzy
decision tree approach for database classification. In
International Journal: Expert Systems with Applica-
tions.
Congalton, R. (1991). A review of assessing the accuracy
of classifications of remotely sensed data. In IEEE
Transactions on Systems, Man, and Cybernetics, Part
C: Applications and Reviews.
Elouedi, Z., Mellouli, K., and Smets, P. (2001). Belief de-
cision trees: Theoretical foundations. In International
Journal of Approximate Reasoning.
Fried, M., Brodley, C., and Strahler, A. (1999). Maximiz-
ing land cover classication accuracies produced by de-
cision trees at continental to global scales. In IEEE
Transactions on Geoscience and Remote Sensing.
Garey, M. and Johnson, D. (1979). Computers and in-
tractability: A guide to the theory of np-completeness.
In Printed in the United States of America.
Hullermeier, E. (2002). Possibilistic induction in decision-
tree learning. In ECML ’02 Proceedings of the 13th
European Conference on Machine Learning.
Jiang, L., Wang, W., Yang, X., and N. Xie, Y. C. (2011).
Classification methods of remote sensing image based
on decision tree technologies. In Computer and Com-
puting Technologies in Agriculture IV.
Kumar, U., Kerle, N., Punia, M., and Ramachandra, T.
(2011). Mining land cover infor-mation using mul-
tilayer perceptron and decision tree from modis data.
In Journal of the Indian Society of Remote Sensing.
Levashenko, V. and Zaitseva, E. (2012). Fuzzy decision
trees in medical decision making support system. In
Proceedings of the Federated Conference on Com-
puter Science and Information Systems.
McIver, D., Friedl, M., and Dzan, W. (2002). Using
prior probabilities in decision-tree classification of re-
motely sensed data. In Remote Sensing of Environ-
ment.
Mishra, P., Singh, D., and Yamaguchi, Y. (2011). Land
cover classification of palsar images by knowledge
based decision tree classifier and supervised classifiers
based on sar observables. In Progress In Electromag-
netics Research B.
Mitra, S., Konwar, K., and Pal, S. (2002). Fuzzy decision
tree, linguistic rules and fuzzy knowledge-based net-
work: generation and evaluation. In Remote Sensing
of Environment.
Neagoe, V., Neghina, M., and Datcu, M. (2011). Neural
network techniques for automated land-cover change
detection in multispectral satellite time series imagery.
In International Journal of Mathematical Models and
Methods in Applied Sciences.
Ozols, M., Mancinska, L., Berzina, I., Agadzanjans, R.,
and Rosmanis, A. (2006). Principles of optimal prob-
abilistic decision tree construction. In International
Conference on Foundations of Computer Science. Las
Vegas, Nevada, USA.
Panda, S., Garg, V., and Chaubey, I. (2004). artificial neural
networks application in lake water quality estimation
using satellite imagery. In Journal of Environmental
Informatics.
Pulvirenti, L., Pierdicca, N., and Marzano, F. (2011). Pre-
diction of the error induced by topography in satellite
microwave radiometric observations. In IEEE Trans-
actions on Geoscience and Remote Sensing.
Quinlan, J. (1986). Induction on decision trees. Machine
Learning.
AnApproachbasedonAdaptiveDecisionTreeforLandCoverChangePredictioninSatelliteImages
89