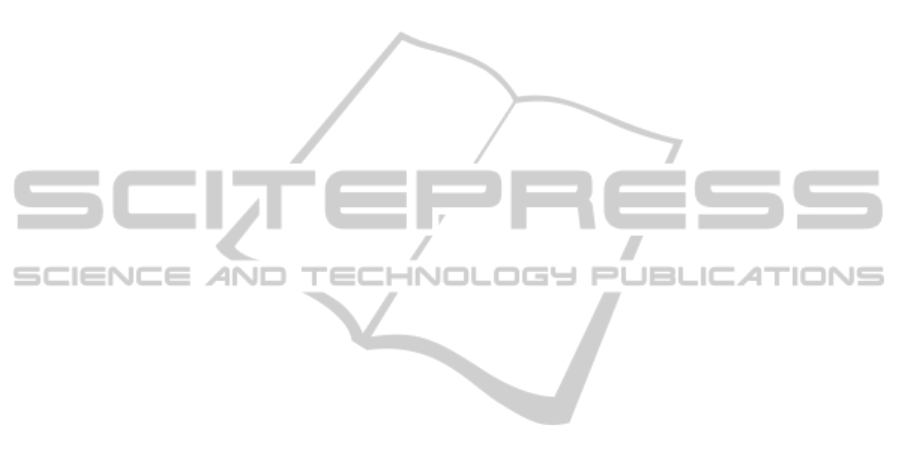
the effect size is computed. The histogram of 100,000
credible effect sizes has a mode of 0.103 and the zero
included in the 95% HDI. 66.5% of all computed out-
comes are positive while 27.8% are negative.
What can we derive from that? What is true is that
there is some probability that there is absolutely no
effect caused by the different signals in the advertise-
ments as we do not observe strongly significant un-
ambiguous results. If any effect is presumed, it will
have a higher probability of being positive for ”green
signals” in Sponsored Search ads, given the observed
data. How can this outcome be explained? One argu-
ment could be that ad texts do not influence users on
SERPs at all. Although we know about various other
effects, like the strong position bias described above,
that do affect the user there are too many indications
that ad texts do have influence on click decisions to
let this be true.
In fact, these results need to be interpreted with
caution. One possible explanation for this is that
users might not be as green in their decisions as mar-
keters would like them to be. In this case the promise
of ”Fast and Reliable delivery” seams to lead to a
slightly lower motivation to click on an ad than the
green signals the advertiser sends out to his potential
customers. This A/B test should be repeated over a
number of various branches before one can derive im-
plications for the whole e-Commerce industry. What
is an even more interesting outcome of this paper is
that more future research should be conducted on the
general impact of texts in Sponsored Search ads con-
sidering a variety of branches and containing more di-
versity in texts to make sophisticated assumptions on
the impact of text-details on click probabilities.
REFERENCES
Adar, E. (2007). User 4xxxxx9: Anonymizing query logs.
In Query Logs Workshop, WWW, volume 7. Citeseer.
Agarwal, A., Hosanagar, K., and Smith, M. D. Location,
Location, Location: An Analysis of Profitability of
Position in Online Advertising Markets. Journal of
Marketing Research, (Forthcoming).
Alby, T. and Funk, B. (2011). Search Engine Marketing in
Small and Medium Companies: Status Quo and Per-
spectives. In Cruz-Cunha, M. and Varaj˜ao, J., editors,
E-Business Managerial Aspects, Solutions and Case
Studies, pages 206–221. igi-global.
Blask, T. (2013). Investigating the Promotional Effect of
Green Signals in Sponsored Search Advertising us-
ing Bayesian Parameter Estimation. In Proceedings
of the International Conference on Information Tech-
nologies in Environmental Engeneering (ITEE 2013).
Blask, T., Funk, B., and Schulte, R. (2011). Should Com-
panies Bid on their Own Brand in Sponsored Search?
In Proceedings of the International Conference on e-
Business (ICE-B 2011), pages 14–21, Seville, Spain.
Citeseer.
Brenes, D. J. and Gayo-Avello, D. (2009). Stratified
analysis of AOL query log. Information Sciences,
179(12):1844–1858.
Chapelle, O. and Zhang, Y. (2009). A dynamic bayesian
network click model for web search ranking. In
Proceedings of the 18th international conference on
World wide web - WWW ’09, page 10, New York, New
York, USA. ACM Press.
Craswell, N., Zoeter, O., Taylor, M., and Ramsey,
B. (2008). An experimental comparison of click
position-bias models. In Proceedings of the interna-
tional conference on Web search and web data mining
- WSDM ’08, page 8, New York, New York, USA.
ACM Press.
Dave, K. S. and Varma, V. (2010). Learning the click-
through rate for rare/new ads from similar ads. In
Proceeding of the 33rd international ACM SIGIR con-
ference on Research and development in information
retrieval - SIGIR ’10, number July, page 897, New
York, New York, USA. ACM Press.
Dembczynski, K., Kotlowski, W., and Weiss, D. (2008).
Predicting ads click-through rate with decision rules.
In World Wide Web, volume 2008.
Evans, D. S. (2008). The Economics of the Online Ad-
vertising Industry. Review of Network Economics,
7(3):pp359–391.
Evans, D. S. (2009). The Online Advertising Industry: Eco-
nomics, Evolution, and Privacy. Journal of Economic
Perspectives, 23(3):37–60.
Gauzente, C. (2009). Information search and paid results
proposition and test of a hierarchy-of-effect model.
Electronic Markets, 19(2-3):163–177.
Gluhovsky, I. (2010). Forecasting Click-Through Rates
Based on Sponsored Search Advertiser Bids and In-
termediate Variable Regression. October, 10(3):1–28.
Haytko, D. and Matulich, E. (2008). Green advertising
and environmentally responsible consumer behaviors:
Linkages examined. Journal of Management and
Marketing Research, 1:2–11.
Jansen, B. and Mullen, T. (2008). Sponsored search: an
overview of the concept, history, and technology. In-
ternational Journal of Electronic Business, 6(2):114–
131.
Jansen, B. J., Flaherty, T. B., Baeza-Yates, R., Hunter, L.,
Kitts, B., and Murphy, J. (2009). The Components and
Impact of Sponsored Search. Computer, 42(5):98–
101.
Jansen, J. (2011). The Serious Game of Bidding on Key-
words. In Understanding Sponsored Search Core El-
ements of Keyword Advertising, chapter 8, pages 176–
201. Cambridge University Press.
Joachims, T., Granka, L., Pan, B., Hembrooke, H., and
Gay, G. (2005). Accurately interpreting clickthrough
data as implicit feedback. In Proceedings of the 28th
annual international ACM SIGIR conference on Re-
search and development in information retrieval - SI-
GIR ’05, page 154, New York, New York, USA. ACM
Press.
ICE-B2013-InternationalConferenceone-Business
318