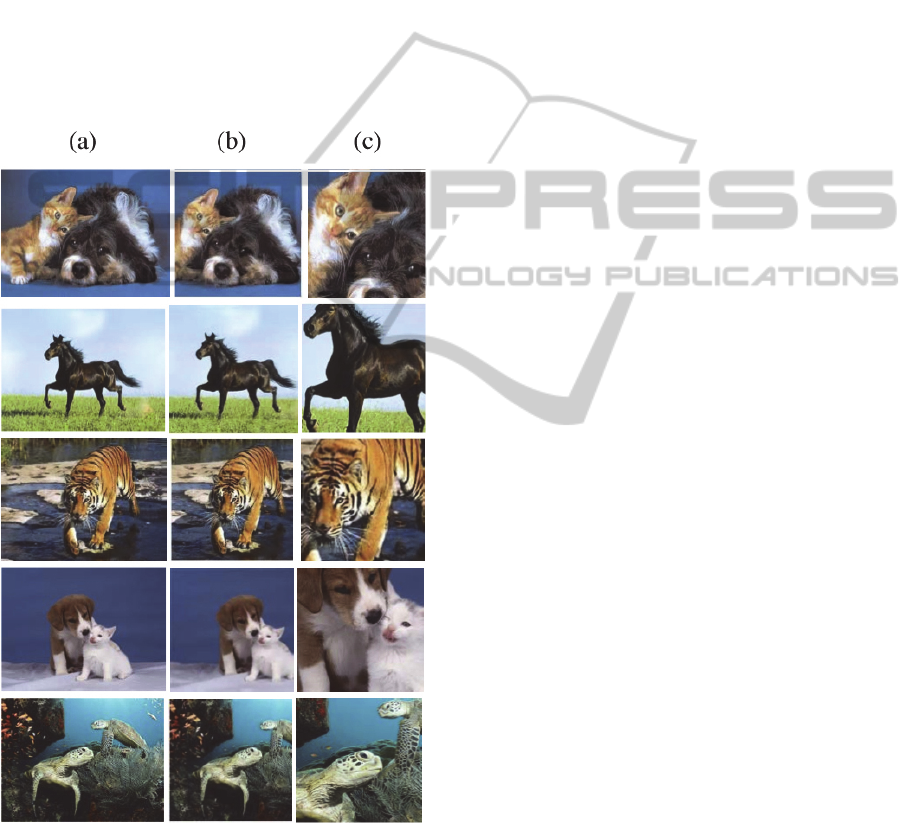
images obtained using the keyword "Animals". In
column (a) are shown the original images, in column
(b) the previews extracted from Yahoo!, in column
(c) our results.
The proposed algorithm generally focuses on
salient part producing a reduced part of the original
image while Yahoo! seems to simply crop the
image.
Generally, this representation is useful if the
previews are used for searching for details and
content of pictures or known image. High presence
of background in the preview reduce the information
shown. In personal photo collection, for example,
having a salient and zoomed part of an image can
lead to a faster visual retrieval of the image.
Figure 8: Comparison of yahoo thumbnails and the result
of the proposed method.
6 CONCLUSIONS
We presented a simple technique to automatically
extract relevant information from digital images
using a bottom-up visual saliency model.
The technique has been applied to detect the
most relevant subjects in the foreground also in the
case of cluttered background. Performance were
evaluated on small annotated image dataset we
prepared for our experiments and that will be
available for download and could be used for future
comparison.
A variation of the same technique has also been
demonstrated on the problem of smart thumbnailing
that is the creation of thumbnails keeping most of
the information of the original image using a much
smaller number of pixels. A qualitative comparison
with Yahoo! thumbnails has shown also in this case
the goodness of our approach.
In both cases we observed that filtering the
saliency map significantly increases the
performance.
Finally, even though in many cases a top-down
model-based approach might be preferable we have
shown that, in many cases, a simple data driven
approach could be satisfactory.
REFERENCES
Harel, J., Koch, C. and Perona, P., 2006. Graph-Based
Visual Saliency. Proceedings of Neural Information
Processing Systems (NIPS).
Marchesotti, L., Cifarelli, C. and Csurka, G., 2009. A
framework for visual saliency detection with
applications to image thumbnailing. Xerox Research
Centre Europe (XRCE), France
Suh, B., Ling, H., Bederson, B.B., Jacobs, D.W., 2003.
Automatic Thumbnail Cropping and its Effectiveness.
Proceedings of the 16th annual ACM symposium on
User interface software and technology
Liu, L., Chen, R., Wolf, L., Cohen-Or, D., 2010.
Optimizing Photo Composition. Computer Graphics
Forum, vol. 29, n. 2 pp. 469-478, Wiley Online
Library
Zhang, M., Zhang, L., Sun, Y., Feng, L., Ma, W., 2005.
Auto Cropping for Digital Photographs., IEEE
International Conference on Multimedia and Expo,
(ICME).
Liu, T., Yuan, Z., Sun, J., Wang, J., Zheng, N., Tang, X.
and Shum, H.-Y., 2007. Learning to detect a salient
object. IEEE Trans. Pattern Anal. Mach.
Cheng, M.-M., Zhang, G.-X., Mitra, N. J., Huang, X. and
Hu, S.-M., 2011. Global contrast based salient region
detection. IEEE Conference on Computer Vision and
Pattern Recognition (CVPR).
Wang, L., Xue, J., Zheng, N. and Hua, G., 2011.
Automatic salient object extraction with contextual
cue. IEEE Conference on Computer Vision (ICCV).
FullyAutomaticSaliency-basedSubjectsExtractioninDigitalImages
135