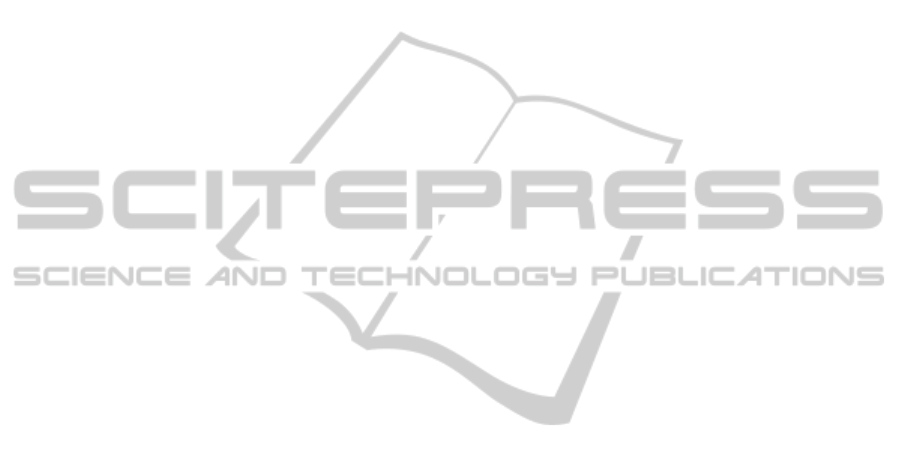
Shanghai Volkswagen and Honda China. About 35%
firms belong to high-tech manufacturing group,
example include Lenovo, Samsung China, Benq,
Foxconn, Chinabird Huawei and et al. We found no
significant differences of organizational size and
sales in the two groups by using one-way ANOVA.
4.2 Data Analysis
A covariance-based Structural Equation Model
(SEM) analysis was used for data analysis.
Exploratory factor analysis (EFA) was first
conducted using SPSS 16.0 to validate the proposed
factor structures. EFA showed the presence of ten
factors in the data and the factor structures matched
the ones we identified in the research model. The
results of measuring IT related resources and e-
supply chain capability represented two and three
factors solution with 64% and 77% cumulative
percent of variance extracted respectively. These
items employed in multi-level performance revealed
five factors with 85% cumulative percent of variance
extracted. The values of KMO are all above 0.85
with significant Bartlett’s test of sphericity at 0.05
levels. The factor structures suggested by the EFA
match the one proposed in the research model.
Next, confirmatory factor analysis (CFA) was
conducted to check the reliability and validity of the
measurement model using Lisrel.8.72. Construct
reliability was measured using Cronbach’s alpha and
composite reliability. The Cronbach’s alpha ranges
from 0.79 to 0.92 for the 10 constructs, indicating a
high internal consistency (Straub et al., 2004).
Further, composite reliability was evaluated and
found to be similar, based on which we may
conclude that the reliability for these constructs is
adequate (Straub et al., 2004). Convergent and
discriminant validities were examined by both factor
loadings and a correlation matrix. All estimated
standard loadings are significant (p<0.001),
suggesting good convergent validity. All square root
of AVEs were above 0.707 (AVEs were above 0.50),
and they are much larger than all cross-correlations.
In sum, the results indicate good convergent and
discriminant validity.
The research model was tested with Lisrel 8.72,
results are presented in Fig. 1. Several GFI indexes
of the structural model have been widely used in IS
research arena. The normed 2(2 to degree of
freedom) is 2.4, which is within the recommended
level of 3.0Barua et al. 2004). The incremental fit
indices include the normed fit index (NFI), Non-
Normed Fit Index(NNFI), comparative fit index
(CFI), and incremental fit index (IFI), which are all
higher than 0.9. This implies a good model fit (Hu
and Bentler, 1999). Results suggested that the
structural model fit the data adequately.
4.3 Hypothesis Tests
Out of 15, 14 hypotheses are supported in our study;
we also provide an overall validation of the model of
e-supply chain value shown in Fig. 1.
Since our model proposes that e-supply chain
capability(ESSC) are intermediate transferring
capabilities between inter-firm IT resource
integration and process performance, we test these
effects in three upstream and downstream processes
at the same time. In the e-procurement process, only
Internal IT resources integration of a focal firm have
a positive effect on e-procurement capabilities (EPC)
(β=0.64, p<0.001), while partner resources does not
have a significant impact (β=0.12, p>0.05). Thus, H2
is not supported. In the e-ordering process, the
results indicate the Internal IT resources integration
(β=0.61, p<0.001) is more likely to be associated
with the development of e-ordering capability (EPC)
than partner resources (β=0.15, p<0.05). Similarly,
the results also predict the impact of Internal IT
resources integration (β=0.67, p<0.001) and partner
resources (β=0.16, p<0.05) on the generation of
CRM capability (CRMC). Therefore, these results
support the hypothesis that firms have engaged in
integrating inter-firm IT resources together with
partners to create unique e-supply chain capability
through upstream and downstream e-business
processes.
We further tested the e-supply chain process
performance co-create by e-supply chain capability
in upstream and downstream e-business processes.
Hypotheses H7, H8, and H9 are all strongly
supported at 0.001 significance level, demonstrating
that ESSC involving in procurement (β=0.57,
p<0.001), ordering (β=0.66, p<0.001), and the CRM
process (β=0.65, p<0.001) leads to improved
individual process performance respectively.
We also identify the role of process performance
in transforming from e-supply chain capability to
financial performance (FPF) and collaborative
network performance (CNPF). The model shows a
significantly positive linkage between e-supply chain
process performance and financial performance in
the e-procurement (β=0.32, p<0.001) with higher
magnitude and greater significance than in the e-
ordering (β=0.30, p<0.05) and CRM (β=0.14,
p<0.001). Therefore, H10, H11, and H12 are
supported in this study. H13, H14, and
H15 predicted that process performance also would
Frome-SupplyChainCapabilityGenerationtoInformationTechnologyValueCo-creation-APerspectiveofe-Business
Process
157