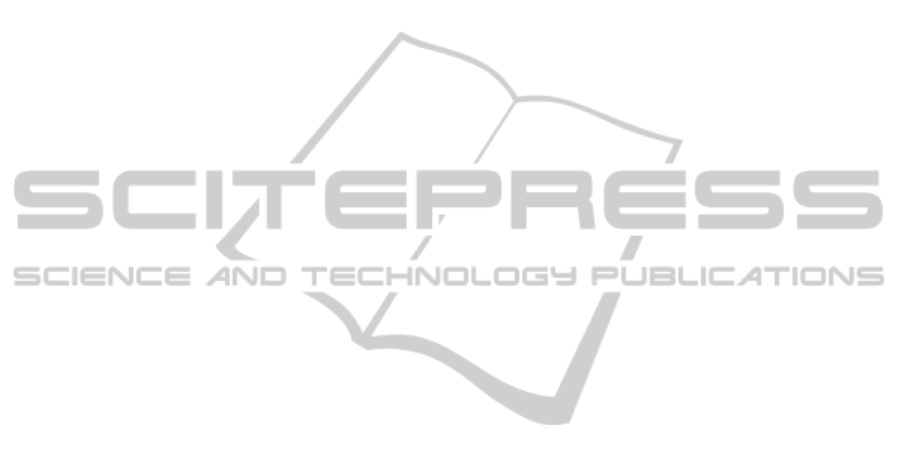
scope of this paper.
To demonstrate the similarity search we use two
chemical safety cards: Lead Arsenite, and Graphite
(Natural). We picked these two chemicals as we have
some background knowledge about them. The most
similar chemical card for Lead Arsenite was Lead
(II) Arsenite, and for Graphite (Natural) was Carbon.
Even without expertise in the area, we can see that
these two chemicals are relevant considering the orig-
inal chemical safety cards. To experiment the sim-
ilarity search, we conducted the search for 20 dif-
ferent chemicals. In the experiment, 90 % of the
highest scoring documents were considered very rel-
evant. Even though the number of queries performed
is small, we get a good indication of the feasibility of
the approach.
5 CONCLUSIONS
In this paper we have proposed a novel approach for
domain modeling that combines a fuzzy ontology and
an association network. We use the fuzzy ontology
to describe the hierarchical relationship of chemicals
and their attributes whereas the association network
describes the associations between the attributes of
the chemicals. The model is used for query expan-
sion in the chemical domain.
We also use the models to find similar chemical
safety cards. Here, the aim is to use the associations
to weight the cosine similarity assessment and find
which chemicals have similar attributes.
The proposed models produced promising results.
The query expansion performed as expected as it was
able to produce more information than a search where
the expansion was not used. In addition, the asso-
ciation weighted similarity assessment was able to
find chemical safety cards that we consider relevant.
Overall, the search engine performed better when the
query expansion and the similarity assessment are
used. This is crucial when considering the real world
applications.
The main challenge for the future is to lessen the
burden of the ontology creation without impacting its
capability to capture the concepts of the given do-
main. Learning the association network is an easier
task but identifying the keywords and terms to be used
in the network also requires some work. In addition,
the maintenanceof both models is time consuming. In
the future, it may be beneficial to utilize the associa-
tion network in ontology creation as the network may
hold valuable information about the domain and the
relationships of the terms. In order to achieve this we
would need to utilize an approach for keyword iden-
tification from documents. In conclusion, we believe
that by combining the association network with the
ontology we are able to create a richer model of the
domain that can be better utilized in different types of
applications.
ACKNOWLEDGEMENTS
Authors wish to thank the Finnish Funding Agency
for technology and Innovation (TEKES) for funding
this research and the anonymous reviewers for the
valuable feedback.
REFERENCES
Agrawal, R., Imielinski, T., and Swami, A. N. (1993). Min-
ing association rules between sets of items in large
databases. In Proceedings of the 1993 ACM SIG-
MOD International Conference on Management of
Data (SIGMOD’93), USA, pages 207–216.
Bhogal, J., Macfarlane, A., and Smith, P. (2007). A review
of ontology based query expansion. Information Pro-
cessing & Management, 43(4):866 – 886.
Bordogna, G. and Pasi, G. (2000). Application of fuzzy set
theory to extend boolean information retrieval. Studies
in Fuzziness and Soft Computing, 50:21–47.
Carlsson, C., Brunelli, M., and Mezei, J. (2010). Fuzzy
ontology and information granulation: an approach to
knowledge mobilisation. Information Processing and
Management of Uncertainty in Knowledge-Based Sys-
tems. Applications, pages 420–429.
Carpineto, C. and Romano, G. (2012). A survey of auto-
matic query expansion in information retrieval. ACM
Computing Surveys, 44(1):1:1–1:50.
Crestani, F. (1997). Application of spreading activation
techniques in information retrieval. Artificial Intelli-
gence Review, 11(6):453–482.
Cross, V. (2004). Fuzzy semantic distance measures be-
tween ontological concepts. In Fuzzy Information,
2004. Processing NAFIPS’04. IEEE Annual Meeting
of the, volume 2, pages 635–640. IEEE.
Formica, A., Missikoff, M., Pourabbas, E., and Taglino, F.
(2008). Weighted ontology for semantic search. On
the Move to Meaningful Internet Systems: OTM 2008,
pages 1289–1303.
Hirvonen, J., Tommila, T., Pakonen, A., Carlsson, C.,
Fedrizzi, M., and Full´er, R. (2010). Fuzzy keyword
ontology for annotating and searching event reports.
In KEOD 2010 - Proceedings of the International
Conference on Knowledge Engineering and Ontology
Development, Valencia, Spain, October 25-28, 2010,
pages 251–256.
Janowicz, K., Raubal, M., and Kuhn, W. (2012). The
semantics of similarity in geographic information
retrieval. Journal of Spatial Information Science,
(2):29–57.
KEOD2013-InternationalConferenceonKnowledgeEngineeringandOntologyDevelopment
36