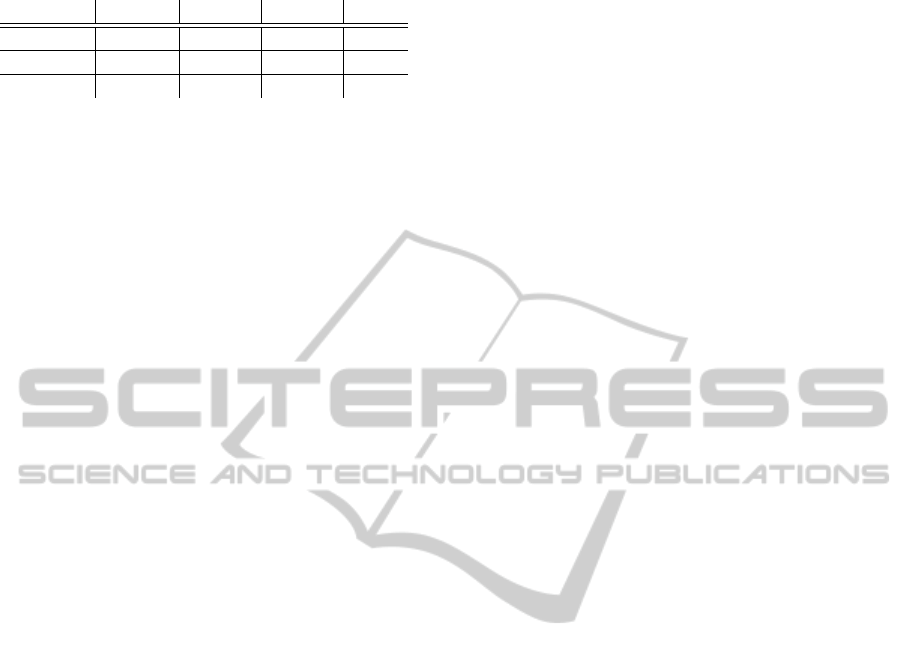
Table 4: Summary of results for QAR
2
.
Attribute Atomic ∈ Cons 6∈ Cons Total
Subsc > 20 28 1 29
Tric > 10 7 4 11
Chol ≥ 240 18 12 30
sidered as a consequence of this SI-formula. Thus
it seems reasonable to try to confirm the hypothe-
sis Subsc ↑
+
Hpt. In addition, we can conclude that
there are no strong indications of Tric ↑
+
Hpt and
Chol ↑
+
Hpt.
However, there are various possibilities of mod-
ifications of parameters of the set of relevant an-
tecedents, modifications of the quantifier ∼
+
0.5,30
and
modifications of the definitions of sets of conse-
quences of Tric ↑
+
Hpt and Chol ↑
+
Hpt. This can
lead to revision of the introduced conclusions.
8 CONCLUSIONS
We have presented a new way of dealing with domain
knowledge in association rules data mining. This is
based on mapping items of domain knowledge to sets
of association rules which can be considered as their
consequences. It was shown that there is both nec-
essary theory based on logic of association rules and
the 4ft-Miner procedure realizing relevant operations
with data, items of knowledge and rules. This makes
possible to formulate interesting analytical questions
and answer them in an efficient way. There is a very
fine way to define sets of relevant association rules.
These association rules, when true in data, can be con-
sidered as the smallest possible indications of more
complex dependences among related attributes.
However, there is still a challenge concerning sen-
sitivity of the presented approach to various param-
eters. There is also a challenge of combining of the
4ft-Miner procedure for mining the presented syntac-
tically rich association rules with additional data min-
ing procedures, namely with procedures of the LISp-
Miner system dealing with various contingency tables
(H
´
ajek et al., 2010). These topics are subjects of fur-
ther work.
ACKNOWLEDGEMENTS
The work described here has beeen supported by the
grant IGA 20/2013 of the University of Economics,
Prague.
REFERENCES
Agrawal, R., Mannila, H., Srikant, R., Toivonen, H., and
Verkamo, A. I. (1996). Fast discovery of association
rules. In Advances in Knowledge Discovery and Data
Mining, pages 307–328. AAAI/MIT Press.
Brossette, S. E., Sprague, A. P., Hardin, J. M., Waites, K. B.,
Jones, W. T., and Moser, S. A. (1998). Research pa-
per: Association rules and data mining in hospital in-
fection control and public health surveillance. JAMIA,
5(4):373–381.
Delgado, M., Ruiz, M., and Sanchez, D. (2011). New
approaches for discovering exception and anoma-
lous rules. International Journal of Uncertainty and
Knowledge-based Systems, 19(2):361–399.
Delgado, M., Sanchez, D., Martin-Bautista, M., and Vila,
M. (2001). Mining association rules with improved
semantics in medical databases. Artificial Intelligence
in Medicine, 21(1–3):241–245.
H
´
ajek, P. and Havr
´
anek, T. (1978). Mechanizing Hypothesis
Formation (Mathematical Foundations for a General
Theory. Springer–Verlag, Berlin Heidellberg New
York, 1st edition.
H
´
ajek, P., Hole
ˇ
na, M., and Rauch, J. (2010). The GUHA
method and its meaning for data mining. Journal of
Computer and System Science, 76(1):34–48.
Ordonez, C., Ezquerra, N., and Santana, C. A. (2006). Con-
straining and summarizing association rules in medi-
cal data. Knowledge and Information Systems (KAIS),
9(3):259–283.
Rauch, J. (2011). Consideration on a formal frame for data
mining. In Hong, T.-P., Kudo, Y., Kudo, M., Lin, T. Y.,
Chien, B.-C., Wang, S.-L., Inuiguchi, M., and Liu, G.,
editors, GrC, pages 562–569. IEEE.
Rauch, J. (2013). Observational Calculi and Association
Rules, volume 469 of Studies in Computational Intel-
ligence. Springer.
Rauch, J. and
ˇ
Sim
˚
unek, M. (2005). An alternative approach
to mining association rules. In Lin, T. Y., Ohsuga, S.,
Liau, C.-J., Hu, X., and Tsumoto, S., editors, Founda-
tions of Data Mining and knowledge Discovery, vol-
ume 6 of Studies in Computational Intelligence, pages
211–231. Springer.
Rauch, J. and
ˇ
Sim
˚
unek, M. (2011). Applying domain
knowledge in association rules mining process - first
experience. In Kryszkiewicz, M., Rybinski, H.,
Skowron, A., and Ras, Z. W., editors, ISMIS, volume
6804 of Lecture Notes in Computer Science, pages
113–122. Springer.
Roddick, J. F., Fule, P., and Graco, W. J. (2003). Ex-
ploratory medical knowledge discovery: experiences
and issues. SIGKDD Explorations, 5(1):94–99.
UsingDomainKnowledgeinAssociationRulesMining-CaseStudy
111