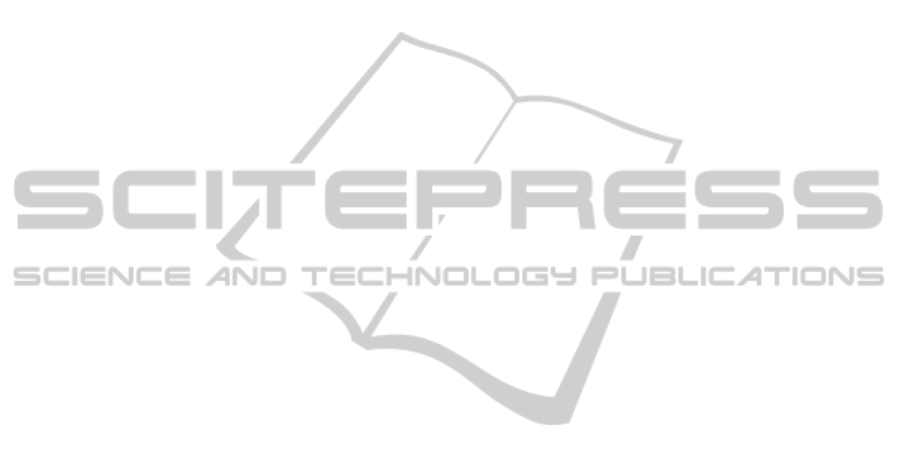
F. Ferre and P. Clote (2005). Disulfide connectivity pre-
diction using secondary structure information and
diresidue frequencies. Bioinformatics, 21(10):2336–
2346.
Guantao Chen, Hai Deng, Yufeng Gui, Yi Pan, and Xue
Wang (2006). Cysteine separations profiles on pro-
tein secondary structure infer disulfide connectivity.
In 2006 IEEE International Conference on Granular
Computing, pages 663–665.
Hsuan-Liang Liu and Shih-Chieh Chen (2007). Prediction
of disulfide connectivity in proteins with support vec-
tor machine. Journal of the Chinese Institute of Chem-
ical Engineers, 38(1):63–70.
Jayavardhana Rama G. L., Alistair P. Shilton, Michael M.
Parker, and Marimuthu Palaniswami (2005). Predic-
tion of cystine connectivity using svm. Bioinforma-
tion, 1(2):69–74.
Jianlin Cheng, Hiroto Saigo, and Pierre Baldi (2006).
Large-scale prediction of disulphide bridges using
kernel methods, two-dimensional recursive neural net-
works, and weighted graph matching. PROTEINS:
Structure, Function, and Genetics, 62:617–629.
Leonid A. Mirny and Eugene I. Shakhnovich (1996). How
to derive a protein folding potential? a new approach
to an old problem. Journal of Molecular Biology,
264(5):1164–1179.
Marc Vincent, Andrea Passerini, Matthieu Labbe, and Paolo
Frasconi (2008). A simplified approach to disulfide
connectivity prediction from protein sequences. BMC
Bioinformatics, 9(1):20.
P. Frasconi, A. Passerini, and A. Vullo (2002). A two-stage
svm architecture for predicting the disulfide bonding
state of cysteines. In Proceedings of the IEEE Work-
shop on Neural Networks for Signal Processing, pages
25–34.
Paul M. Harrison and Michael J. E. Sternberg (1994). Anal-
ysis and classification of disulphide connectivity in
proteins : The entropic effect of cross-linkage. Jour-
nal of Molecular Biology, 244(4):448–463.
Pier Luigi Martelli, Piero Fariselli, Luca Malaguti, and Rita
Casadio (2002). Prediction of the disulfide-bonding
state of cysteines in proteins at 88% accuracy. Protein
Science, 11:2735–2739.
Piero Fariselli, Paola Riccobelli, and Rita Casadio (1999).
Role of evolutionary information in predicting the
disulfide-bonding state of cysteine in proteins. PRO-
TEINS: Structure, Function, and Genetics, 36:340–
346.
Piero Fariselli and Rita Casadio (2001). Prediction of
disulfide connectivity in proteins. Bioinformatics,
17(10):957–964.
Pierre Baldi, Jianlin Cheng, and Alessandro Vullo (2005).
Large-scale prediction of disulphide bond connectiv-
ity. In Advances in Neural Information Processing
Systems 17, pages 97–104, Cambridge, MA, USA.
MIT Press.
Rotem Rubinstein and Andras Fiser (2008). Predicting
disulfide bond connectivity in proteins by correlated
mutations analysis. Bioinformatics, 24(4):498–504.
Sarunas Raudys and Fabio Roli (2003). The behavior
knowledge space fusion method: Analysis of general-
ization error and strategies for performance improve-
ment. In In Proc. Int. Workshop on Multiple Classifier
Systems (LNCS 2709, pages 55–64. Springer.
Stephen F. Altschul, Thomas L. Madden, Alejandro A.
Schaffer, Jinghui Zhang, Zheng Zhang, Webb Miller,
and David J. Lipman (1997). Gapped blast and psi-
blast: a new generation of protein database search pro-
grams. Nucleic Acids Research, 25(17):3389–3402.
Vladimir N. Vapnik (1999). The Nature of Statistical Learn-
ing Theory. Springer.
Wei-Chun Chung, Chang-Biau Yang, and Chiou-Yi Hor
(2009). An effective tuning method for cysteine state
classification. In Proc. of National Computer Sym-
posium, Workshop on Algorithms and Bioinformatics,
Taipei, Taiwan.
Yu-Ching Chen (2007). Prediction of Disulfide Connectiv-
ity from Protein Sequences. Ph. D. dissertation, Na-
tional Chiao Tung University, Hsinchu, Taiwan.
Yu-Ching Chen and Jenn-Kang Hwang (2005). Pre-
diction of disulfide connectivity from protein se-
quences. PROTEINS: Structure, Function, and Ge-
netics, 61:507–512.
Yu-Ching Chen, Yeong-Shin Lin, Chih-Jen Lin, and Jenn-
Kang Hwang (2004). Prediction of the bonding states
of cysteines using the support vector machines based
on multiple feature vectors and cysteine state se-
quences. PROTEINS: Structure, Function, and Ge-
netics, 55:1036–1042.
KDIR2013-InternationalConferenceonKnowledgeDiscoveryandInformationRetrieval
118