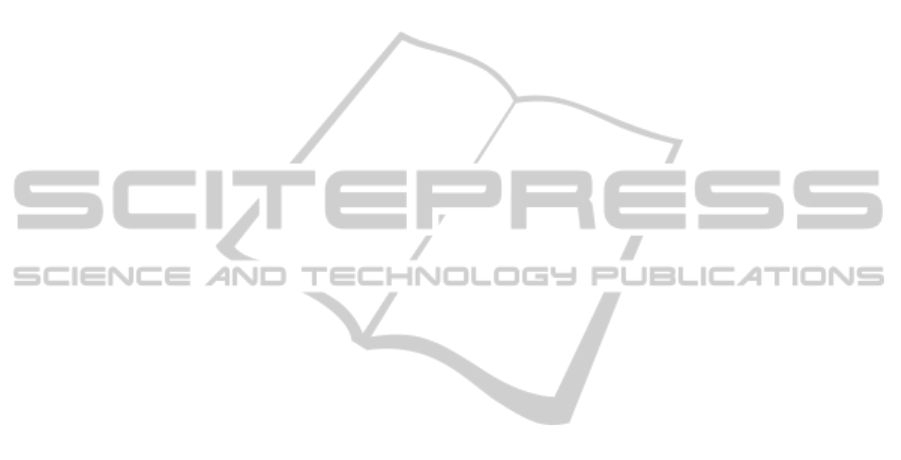
experimental conditions c
j
by which a compound is
tested, can be expressed as an ontology c
j
=> (m
e
, b
t
,
a
i
, l
c
). In this ontology, m
e
represents the measure of
biological effect (anti-enterococci activities or
toxicity). The element b
t
is referred to different
biological targets such as enterococci, Mus musculus
and Rattus norvegicus, and human immune system
cells (lymphocytes). For all biological targets,
information about different strains was taken into
consideration. The element a
i
defines specific
information regarding a test, i.e., if an assay is
focused on the study of functional (F) or
pharmacokinectic/pharmacodynamic profiles (A).
The term l
c
is the level of curation or verification of
the experimental information provided by a
particular test. The elements m
e
, b
t
, a
i
, and l
c
define
the four conditions which can change in our dataset.
So, we had N = 10918 cases from N
c
= 8560
compounds mentioned above, where the
experiments were performed using at least one out
of Nm
e
= 18 measures of biological effects, against
at least one out of Nb
t
= 131 biological targets, in
one out of Na
i
= 2 different types of assay
information, with at least one out of Nl
c
= 3 levels of
curation of the experimental information. In the case
of the element m
e
, we had diverse measures of
biological effects which were expressed in different
units. For this reason, all values of antibacterial
activity against enterococci were converted to nmol/l
(nM), while all toxicity values associated with
laboratory animals were expressed in umol/kg
(micromoles per kilograms). In both kinds of
conversions, it was necessary to divide the value of
each compound by its molar mass, and after multiply
by a factor (usually 10
3
or 10
6
). We realized these
transformations in order to make a better
interpretation of the biological data which permitted
us a more rigorous comparison between the
biological effects of any two compounds, measured
under exactly the same set of conditions c
j
. Data
associated with cytotoxicity against immune cells,
remained in nM. These transformations together
with the element l
c
, also contribute significantly to
reduce and control data uncertainty. All cases in our
dataset were assigned to 1 out of 2 possible groups
related with the biological effect of a defined
compound i in a specific condition c
j
[BE
i
(c
j
)]. Then,
any compound was considered as positive [BE
i
(c
j
) =
1] when it had high anti-enterococci activity, or any
desirable toxicological profile, otherwise, the
compound was considered as negative [BE
i
(c
j
) =
1]. All assignments were realized taking into
account certain cutoff values of biological effects
which are depicted in Table 1. For the whole dataset,
we used a file containing the SMILES of the
compounds/cases. Calculation of TIs using SMILES
was performed with the software MODESLAB
version 1.5 (Estrada and Gutiérrez, 2002-2004). Our
intention is to predict the biological effect of any
compound depending on the molecular structure and
the experimental conditions c
j
. For this reason if we
use the original TIs calculated above, they will not
discriminate the biological effect for a given
compound by varying the different conditions c
j
. To
achieve that goal, and inspired by the use of the
moving average approach (MAA) (Hill and Lewicki,
2006), we introduced new sets of molecular
descriptors like TIs which can be defined according
to the following equation:
ΔTI
i
(c
) = TI
i
– avgTI
i
(c
) (1)
In Eq. 1, the descriptor avgTI
i
(c
j
) characterizes each
set G of compounds tested under the same
experimental condition c
j
, being calculated as the
sum of all the TI
i
values for compounds in a subset
of G, which were considered as positive cases
[BE
i
(c
j
) = 1] in the same element of the ontology
(experimental condition) c
j
. For example, in the case
of the element b
t
, the descriptor avgTI
i
(c
j
) for a set G
of compounds tested against a defined target b
t
(bacterial strain, immune cell, etc), was calculated as
the average of the TI
i
by considering only the subset
of G, i.e., those compounds which were considered
as positive [BE
i
(c
j
) = 1]. A similar procedure was
carried out for the elements m
e
, a
i
, and l
c
. Anyway,
in Eq. 1, the most important element is the
descriptor ΔTI
i
(c
j
), which considers both, the
molecular structure and the experimental conditions
c
j
. For this reason, descriptors of the form ΔTI
i
(c
j
)
(120 in total) were used to develop the mtk-QSBER
model. These descriptors represent the deviation (in
structural terms) of a compound from the positive
compounds. The CHEMBL codes, SMILES and
other relevant experimental data for all the
compounds used in this work, appear in the
Supplementary Information 1 (Suppl. Inf. 1) file.
Our dataset of 10918 cases was randomly split into
two series: training and prediction sets. The training
set was used to construct the mtk-QSBER model.
This was formed by 8298 cases, with 4217 of
them considered as positive and 4081 negative. The
prediction set was used for validation of the model
and assessment of its predictive power, being
composed by 2620 cases, 1353 positive and 1267
negative cases. Taking into consideration that large
number of molecular descriptors, we used a
combination of the attribute evaluator
CFsSubsetEval and the search algorithms called
IJCCI2013-InternationalJointConferenceonComputationalIntelligence
460