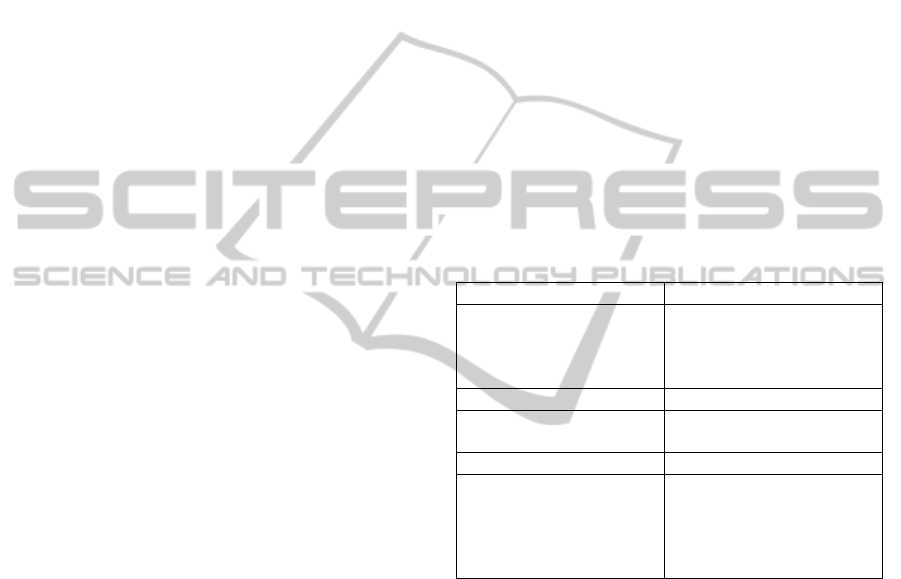
two large energy conversion systems - “electricity
grid” and “mobility” - with each other (KEMPTON;
LETENDRE 1997), several studies from various
perspectives with regard to the realisation of V2G
have been done so far. For instance, studies have
been performed on the potential availability of
electric vehicles (F
LUHR; AHLERT; WEINHARDT
2010), the target market (base load, peak load,
regulation) and the profitability including other
aspects like the influence of the car type (BEV,
FCEV, HEV) for (D
ALLINGER; KRAMPE;
WIETSCHEL 2011).
The effect of V2G on the German electricity
grid has also been investigated (B
IRNBAUM;
LINSSEN; MARKEWITZ [et. al.] 2009). In addition,
there are studies with regard to the integration of
RES by V2G (K
EMPTON; TOMIC 2005).
In summary, while intelligent charging of
batteries might be applicable in Germany´s
electricity market model without problems even at
present day, the application of V2G would however
need serveral modifications (G
UDERGAN; ANSORGE;
FROMBACH 2011). A complete and optimized
integration of electric vehicles into a Smart Grid
requires a bi-directional energy and information
flow. This accounts particularly for the regulation
market, because of high requirements with respect of
reliability and response time of the ICT system.
3 BUSINESS MODEL
There are different concepts of V2G business
models that can be applied for regulation market
services. Every business model can be assigned to
one of two types of models. The first model focuses
on vehicle specific aspects and the second focuses
more on the information respective infrastructural
perspective. The business model described in this
paper is the second one (infrastructure-focused). It
was further investigated for the application for
regulation market services. It is an extension of
existing business models by the very important
factor of battery degradation through additional
cycles because of balancing power services. In this
context, every cycle incurs costs by decreasing the
electric vehicles battery monetary value. Only by
knowing these costs allows to deduct adequate fees
for the supply of balancing power services as a basis
for a robust business model.
3.1 Aggregator of Electric Vehicles
The business model "aggregator of electric vehicles"
is developed exemplary and is based on the
optimization model and the additional information
about degradation available from the car’s BMS.
It is very important to get a deep and clear
understanding of the business model especially from
the very beginning of the conception and planning
stages to enable and facilitate an optimized
integration of the electric vehicle´s batteries into the
regulation service market of smart grids. To comply
with the requirements of smart grid integration, a
structured scientific substantiation and
documentation of the business model would also be
essential. For this the strategic management template
Business Model Canvas was adapted
(O
STERWALDER; PIGNEUR 2010) delivering a broad
overview of the business model´s key issues (Table
1) as a start and subsequently offering a base of
operation for the deduction of specific requirements
regarding a smart grid integration.
Table 1: Key Issues of Electric Vehicles Aggregator’s
Business model.
Key Partners Key Activities
Individuals
Organisations
Public and private
infrastructure operators
Backup storage for
renewable energy sources
Regulation market
services
Key Resources Value Propositions
Electric vehicle´s
battery capacity
Arbitrage profits due to
variable prices
Customer Segments Revenue Streams
System Operators
Individuals
Organisations
Public and private
infrastructure operators
Arbitrage profits due to
variable prices
Shares of arbitrage profits
User fees
Within this business model the aggregator
represents a crucial instance, which initially divides
the required total power into smaller packages, so-
called load profiles. It then translates them into a
format of time and performance vectors that is
interpreted by the BMS of electric vehicles. Finally,
it sends corresponding inquiries to pool of electric
vehicles. The primary objective is to optimize the
use of the battery by additional cycles for the
provision of balancing power, aside from the single
application for driving, so that the battery´s life is
optimally utilized.
Arbitrage profits due to variable prices are in no
doubt the business model´s crucial revenue stream
with regard to smart grid regulation service market.
The realization of arbitrage profits could allow at
least a partial refinancing of the relatively high
investment costs of the vehicles batteries and as a
OptimizedIntegrationofElectricVehicleswithLithiumIronPhosphateBatteriesintotheRegulationServiceMarketof
SmartGrids-EnhancedVehicle-to-GridBusinessModel
89