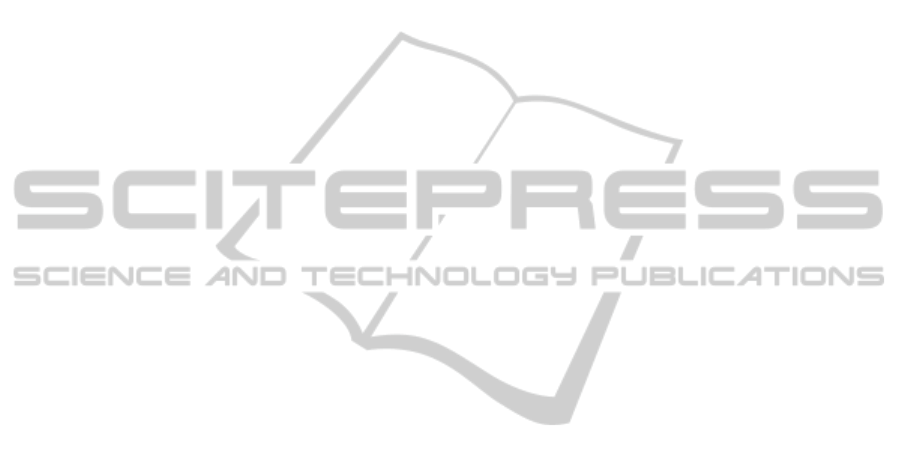
Gonzalez, F. and Dasgupta, D. (2003). Anomaly detec-
tion using real-valued negative selection. In Genetic
Programming and Evolvable Machines. Kluwer Aca-
demic.
Gonzalez, F., Dasgupta, D., and Gomez, J. (2003a). The
effect of binary matching rules in negative selection.
In In Proceedings of the Genetic and Evolutionary
Computation Conference (GECCO 2003). Springer-
Verlag.
Gonzalez, F., Dasgupta, D., and Kozma, R. (2002). Com-
bining negative selection and classification techniques
for anomaly detection. In Proceedings of the 2002
Congress on Evolutionary Computation. IEEE Com-
puter Society.
Gonzalez, F., Dasgupta, D., and Nino, L. F. (2003b). A
randomized real-valued negative selection algorithm.
In In Proceedings of the 2nd International Conference
on Artificial Immune Systems. Springer-Verlag.
Greensmith, J., Aickelin, U., and Twycross, J. (2004). De-
tecting danger: Applying a novel immunological con-
cept to intrusion detection systems. In Proceedings of
the 6th International Conference in Adaptive Comput-
ing in Design and Manufacture. Springer-Verlag.
Hart, E. and Timmis, J. (2008). Application areas of ais:
The past, the present and the future. In Applied Soft
Computing. Elsevier Science.
Hofmeyr, S. A. and Forrest, S. (1999). Immunity by de-
sign: An artificial immune system. In In Proceedings
of the Genetic and Evolutionary Computation Confer-
ence. Morgan Kaufmann.
Hofmeyr, S. A. and Forrest, S. (2000). Architecture for an
artificial immune system. In Evolutionary Computa-
tion Journal. MIT Press.
Jerne, N. (1974). Towards a network theory of the immune
system. In Annals of Immunology. Inst. Pasteur.
Ji, Z. and Dasgupta, D. (2004). Real-valued negative se-
lection algorithm with variable-sized detectors. In In
proceeding of Genetic and Evolutionary Computation.
Springer-Verlag.
Ji, Z. and Dasgupta, D. (2005). A boundary-aware negative
selection algorithm. In In Proceedings of the inter-
national conference on artificial intelligence and soft
computing. ACRA Press.
Ji, Z. and Dasgupta, D. (2007). Revisiting negative selection
algorithms. In MIT Evolutionary Computation. MIT
Press.
Ji, Z. and Dasgupta, D. (2009). V-detector: An efficient
negative selection algorithm with ’probably adequate’
detector coverage. In Information Sciences. Elsevier
Science.
Kim, J. and Bentley, P. J. (2001). An evaluation of negative
selection in an artificial immune system for network
intrusion detection. In Proceedings of the Genetic
and Evolutionary Computation Conference. Morgan
Kaufmann.
Kim, J., Greensmith, J., Twycross, J., and Aickelin, U.
(2005). Malicious code execution detection and re-
sponse immune system inpired by the danger theory.
In Proceedings of the Adaptive and Resilient Comput-
ing Security Workshop (ARCS-05).
L. O. V. B. Oliveira, R. L. M. Motay, D. A. C. B. (2012).
Clonal selection classifier with data reduction: Clas-
sification as an optimization task. In IEEE World
Congress on Computational Intelligence. IEEE.
Li, J., Gao, H., and Wang, S. (2012). A novel clone selec-
tion algorithm with reconfigurable search space ability
and its application. In Fourth International Confer-
ence on Natural Computation. IEEE Computer Soci-
ety.
Liu, L. and Xu, W. (2008). A cooperative artificial immune
network with particle swarm behavior for multimodal
function optimization. In IEEE Congress on Evolu-
tionary Computation. IEEE Press.
Matzinger, P. (2002). The danger model: A renewed sense
of self. In Science.
Powers, S. T. and He, J. (2006). Evolving discrete-valued
anomaly detectors for a network intrusion detection
system using negative selection. In In the 6th Annual
Workshop on Computational Intelligence (UKCI ’06).
University of Leeds.
Roper, M. (2009). Artifical immune systems, danger the-
ory, and the oracle problem. In Testing: Academic and
Industrial Conference - Practice and Research Tech-
niques. IEEE Computer Society.
Stibor, T., Mohr, P., Timmis, J., and Eckert, C. (2005a). Is
negative selection appropriate for anomaly detection?
In Proceedings of the 2005 conference on Genetic and
evolutionary computation. ACM.
Stibor, T., Timmis, J., and Eckert, C. (2005b). A compara-
tive study of real-valued negative selection to statisti-
cal anomaly detection techniques. In Proceedings of
the 4th international conference on Artificial Immune
Systems. Springer-Verlag.
Timmis, J. and Neal, M. (2001). A resource limited artifi-
cial immune system for data analysis. In Knowledge
Based Systems. Elsevier.
Timmis, J., Neal, M., and Hunt, J. (2000). An artificial
immune system for data analysis. In Biosystems. El-
sevier.
X. Yuel, F. Zhang, L. X. and Wangl, D. (2010). Opti-
mization of self set and detector generation base on
real-value negative selection algorithm. In 2010 In-
ternational Conference on Computer and Communi-
cation Technologies in Agriculture Engineering. IEEE
Xplore.
Zeng, J., Liu, X., Li, T., Liu, C., Peng, L., and Sun, F.
(2009). A self-adaptive negative selection algorithm
used for anomaly detection. In Progress in Natural
Science. Elsevier.
Zhong, Y. and Zhang, L. (2012). An adaptive artifi-
cial immune network for supervised classification
of multi-/hyperspectral remote sensing imagery. In
IEEE Transactions on Geoscience and Remote Sens-
ing. IEEE.
Zhu, Y. and Tan, Y. (2011). A danger theory inspired learn-
ing model and its application to spam detection. In
Proceedings of the second international conference on
advances in swarm intelligence. Springer-Verlag.
AReviewofArtificialImmuneSystems
135