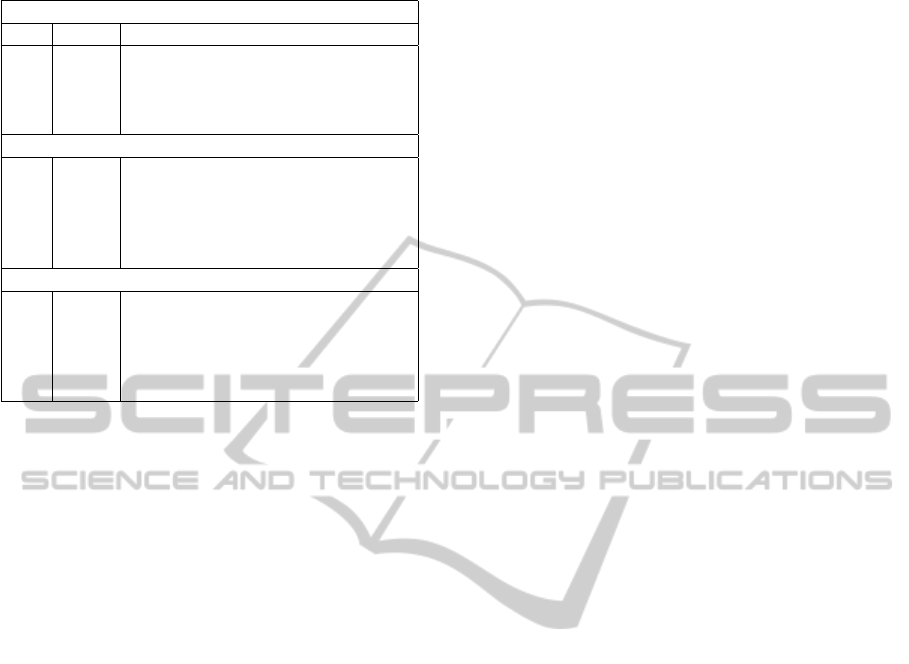
Table 3: Seconds to Reach Accuracy Level.
Dexter
Acc. TEDA UMDA2 FDC GA UMDA1
70.0 0.29 0.3 0.31 0.34 1.65*
76.0 0.4 0.43 0.43 0.54* 2.73*
82.0 0.6 0.63 0.65 0.82* 4.02*
88.0 0.81 1.1* 0.92* 1.46* 6.16*
Arcene
70.0 2.06 3.44* 2.07 3.03* 26.42*
74.0 2.08 3.49* 2.11 3.11* 26.53*
78.0 2.12 3.49* 2.16 3.27* 26.68*
82.0 2.18 3.58* 2.21 3.38* -
86.0 2.28 3.69* 2.35 3.63* -
Madelon
70.0 23.54 24.79 24.02 23.52 30.67
74.0 39.98 35.74 50.0 52.19* 50.01*
78.0 52.43 67.28* 69.27 92.89* 96.97*
82.0 74.17 123.1* 106.58* 168.88* 175.93*
86.0 136.32 210.82* 200.73* 343.32* 270.93*
REFERENCES
Cantu-Paz, E. (2002). Feature subset selection by estima-
tion of distribution algorithms. In Proc. of Genetic and
Evolutionary Computation Conf. MIT Press.
Chang, C. C. and Lin, C. J. (2011). Libsvm: a library for
support vector machines. ACM Trans. on Intelligent
Systems and Technology (TIST), 2(3):27.
Dash, M., Liu, H., and Manoranjan (1997). Feature se-
lection for classification. Intelligent data analysis,
1:131–156.
Frank, A. and Asuncion, A. (2010). UCI machine learning
repository.
Godley, P., Cairns, D., Cowie, J., and McCall, J. (2008).
Fitness directed intervention crossover approaches ap-
plied to bio-scheduling problems. In Symp. on Com-
putational Intelligence in Bioinformatics and Compu-
tational Biology, pages 120–127. IEEE.
Guyon, I., Gunn, S., Ben-Hur, A., and Dror, G. (2004). Re-
sult analysis of the nips 2003 feature selection chal-
lenge. Advances in Neural Information Processing
Systems, 17:545–552.
Inza, I., Larranaga, P., Etxeberria, R., and Sierra, B.
(2000). Feature subset selection by bayesian networks
based on optimization. Artificial Intelligence, 123(1–
2):157–184.
Inza, I., Larranaga, P., and Sierra, B. (2001). Feature sub-
set selection by bayesian networks: a comparison with
genetic and sequential algorithms. Int. Journ. of Ap-
proximate Reasoning, 27(2):143–164.
Keller, J., Gray, M., and Givens, J. (1985). A fuzzy k-
nearest neighbor algorithm. IEEE Trans. on Systems,
Man and Cybernetics, 4:580–585.
Lai, C., Reinders, M., and Wessels, L. (2006). Random sub-
space method for multivariate feature selection. Pat-
tern Recognition Letters, 27(10):1067–1076.
Larranaga, P. and Lozano., J. A. (2002). Estimation of
distribution algorithms: A new tool for evolutionary
computation, volume 2. Springer.
Muhlenbein, H. and Paass, G. (1996). PPSN, volume IV,
chapter From recombination of genes to the estima-
tion of distributions: I. binary parameters., pages 178–
187. Springer, Berlin.
Neumann, G. and Cairns, D. (2012a). Targeted eda adapted
for a routing problem with variable length chromo-
somes. In IEEE Congress on Evolutionary Computa-
tion (CEC), pages 220–225.
Neumann, G. K. and Cairns, D. E. (2012b). Introducing in-
tervention targeting into estimation of distribution al-
gorithms. In Proc. of the 27th ACM Symp. on Applied
Computing, pages 334–341.
Pena, J., V. Robles, V., Larranaga, P., Herves, V., Rosales,
F., and Perez, M. (2004). Ga-eda: Hybrid evolutionary
algorithm using genetic and estimation of distribution
algorithms. Innovations in Applied Artificial Intelli-
gence, pages 361–371.
Pudil, P., J., Novovicova, and Kittler, J. (1994). Floating
search methods in feature selection. Pattern recogni-
tion letters, 15(11):1119–1125.
Saeys, Y., Degroeve, S., Aeyels, D., de Peer, Y. V., and
Rouz, P. (2003). Fast feature selection using a sim-
ple estimation of distribution algorithm: a case study
on splice site prediction. Bioinformatics, 19(suppl
2):179–188.
Siegel, S. and Jr., N. J. C. (1988). Nonparametric Statistics
for The Behavioral Sciences. McGraw-Hill, NY.
ApplyingaHybridTargetedEstimationofDistributionAlgorithmtoFeatureSelectionProblems
143