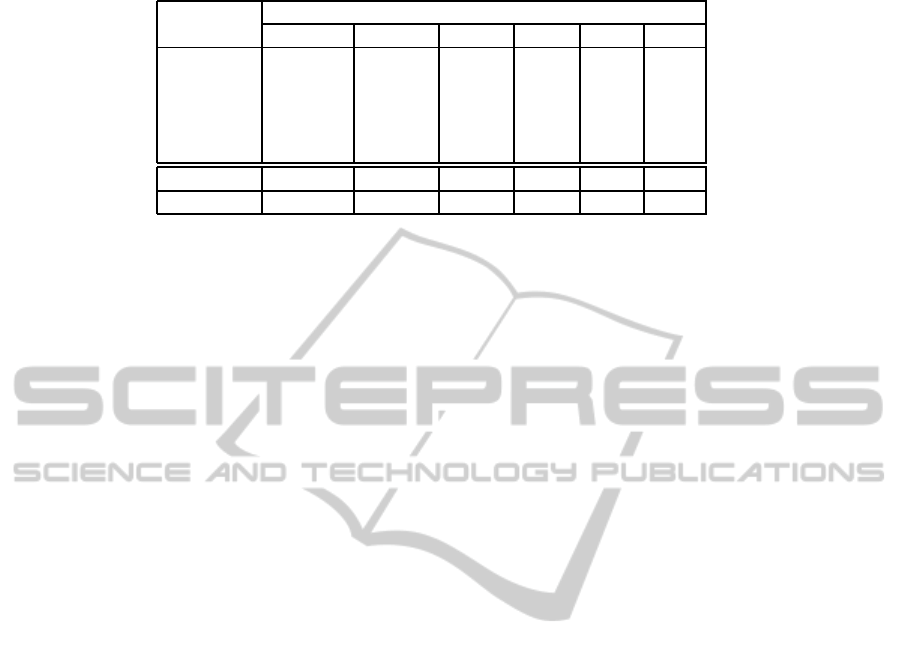
Table 1: MAP on the various test sets.
Test Score
set α
i
= 1∀i α
booking
α
phone
α
URL
α
map
α
title
1 0.75 0.75 0.73 0.76 0.74 0.70
2 0.81 0.73 0.77 0.79 0.77 0.76
3 0.78 0.77 0.79 0.80 0.76 0.79
4 0.75 0.75 0.81 0.76 0.73 0.77
5 0.79 0.74 0.81 0.76 0 .75 0.79
Average 0.78 0.75 0.78 0.77 0.75 0.76
Coverage 0.06 0.04 0.08 0.04 0.04 0.09
REFERENCES
Borlund, P. (2003). The iir evaluation model: a framework
for evaluation of interactive information retrieval sys-
tems. Information research, 8(3):8–3.
Burges, C., Shaked, T., Renshaw, E., Lazier, A., Deeds, M.,
Hamilton, N., and Hullender, G. (2005). Learning to
rank using gradient descent. In Proceedings of the
22nd international conference on Machine learning,
ICML ’05, pages 89–96.
Cao, Z., Qin, T., Liu, T.-Y., Tsai, M.-F., and Li, H. (2007).
Learning to rank: from pairwise approach to listwise
approach. In Proceedings of the 24th international
conference on Machine learning, ICML ’07, pages
129–136.
Chapelle, O. and Zhang, Y. (2009). A dynamic bayesian
network click model for web search ranking. In Pro-
ceedings of 18th International Conference on World
Wide Web, WWW’09, pages 1–10.
Cleverdon, C. W., Mills, J., and Keen, M. (1966). Factors
determining the performance of indexing systems.
Cossock, D. and Zhang, T. (2006). Subset ranking using
regression. In Proceedings of the 19th annual confer-
ence on Learning Theory, COLT’06, pages 605–619.
Springer-Verlag.
Craswell, N., Zoeter, O., Taylor, M., and Ramsey,
B. (2008). An experimental comparison of click
position-bias models. In Proceedings of the 2008 In-
ternational Conference on Web Search and Data Min-
ing, pages 87–94.
Dupret, G., Murdock, V., and Piwowarski, B. (2007). Web
search engine evaluation using clickthrough data and a
user model. In WWW2007 workshop Query Log Anal-
ysis: Social and Technological Challenges.
Freund, Y., Iyer, R., Schapire, R. E., and Singer, Y. (2003).
An efficient boosting algorithm for combining prefer-
ences. Journal of Machine Learning Research, 4:933–
969.
Guo, F., Liu, C., and Wang, Y. M. (2009). Efficient
multiple-click models in web search. In Proceedings
of the Second ACM International Conference on Web
Search and Data Mining, WSDM ’09, pages 124–131.
Harman, D. (2010). Is the cranfield paradigm outdated.
In Proc. 33rd Annual International ACM SIGIR Con-
ference on Research and Development in Information
Retrieval, page 1.
He, J., Zhao, W. X., Shu, B., Li, X., and Yan, H. (2011). Ef-
ficiently collecting relevance information from click-
throughs for web retrieval system evaluation. In Pro-
ceedings of the 34th international ACM SIGIR con-
ference on Research and development in Information
Retrieval, SIGIR ’11, pages 275–284.
Joachims, T. (2002). Optimizing search engines using click-
through data. In Proceedings of the eighth ACM
SIGKDD international conference on Knowledge dis-
covery and data mining, KDD ’02, pages 133–142.
Joachims, T., Granka, L., Pan, B., Hembrooke, H., and
Gay, G. (2005). Accurately interpreting clickthrough
data as implicit feedback. In Proceedings of the 28th
annual international ACM SIGIR conference on Re-
search and development in information retrieval, SI-
GIR ’05, pages 154–161.
Laporte, L., Candillier, L., D´ejean, S., and Mothe, J.
(2012).
´
Evaluation de la pertinence dans les mo-
teurs de recherche g´eor´ef´erenc´es. In Actes du 30`eme
Congr`es INFORSID, pages 281–298.
Liu, C., Guo, F., and Faloutsos, C. (2010). Bayesian
browsing model: Exact inference of document rele-
vance from petabyte-scale data. ACM Transaction on
Knowledge Discovery Data, 4(4):19:1–19:26.
Liu, T.-Y. (2011). Learning to rank for information re-
trieval. Springer.
Nallapati, R. (2004). Discriminative models for information
retrieval. In Proceedings of the 27th annual interna-
tional ACM SIGIR conference on Research and de-
velopment in information retrieval, SIGIR ’04, pages
64–71.
Radlinski, F. and Joachims, T. (2005). Query chains: learn-
ing to rank from implicit feedback. In Proceedings
of the eleventh ACM SIGKDD international confer-
ence on Knowledge discovery in data mining, KDD
’05, pages 239–248.
Yue, Y., Finley, T., Radlinski, F., and Joachims, T. (2007).
A support vector method for optimizing average preci-
sion. In Proceedings of the 30th annual international
ACM SIGIR conference on Research and development
in information retrieval, SIGIR ’07, pages 271–278.
Yue, Y., Gao, Y., Chapelle, O., Zhang, Y., and Joachims,
T. (2010). Learning more powerful test statistics for
click-based retrieval evaluation. In Proceedings of the
33rd annual international ACM SIGIR conference on
Research and development in information retrieval,
SIGIR’10, pages 507–514.
MultipleClicksModelforWebSearchofMulti-clickableDocuments
303