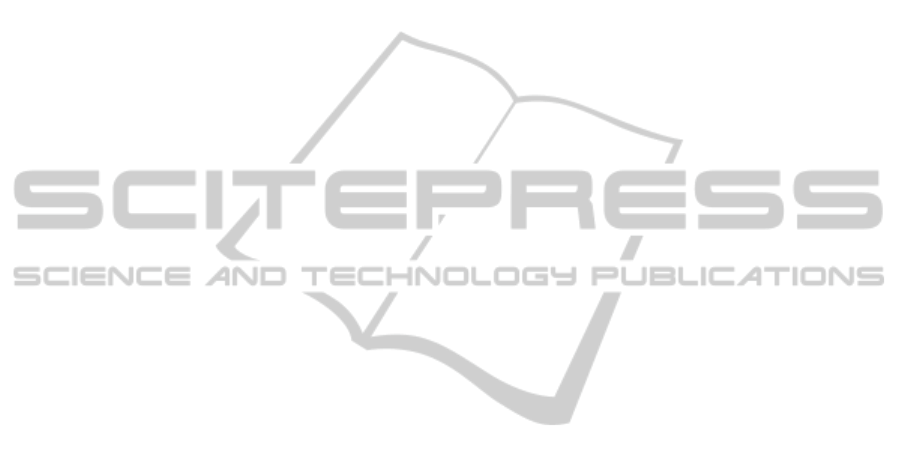
Ashburner, M., Ball, C. A., Blake, J. A., Botstein, D.,
Butler, H., Cherry, J. M., Davis, A. P., Dolinski, K.,
Dwight, S. S., Eppig, J. T., et al. (2000). Gene ontol-
ogy: tool for the unification of biology. Nature genet-
ics, 25(1):25.
Baba, M., Itoh, K., and Tatsuta, M. (2004). Glycine-
extended gastrin induces matrix metalloproteinase-1-
and-3-mediated invasion of human colon cancer cells
through type i collagen gel and matrigel. International
journal of cancer, 111(1):23–31.
Benita, Y., Cao, Z., Giallourakis, C., Li, C., Gardet, A.,
and Xavier, R. J. (2010). Gene enrichment profiles re-
veal t-cell development, differentiation, and lineage-
specific transcription factors including zbtb25 as a
novel nf-at repressor. Blood, 115(26):5376–5384.
Camici, M. (2008). Guanylin peptides and colorectal cancer
(crc). BioMedicine & pharmacotherapy, 62(2):70–76.
Chan, S. K., Griffith, O. L., Tai, I. T., and Jones, S. J. (2008).
Meta-analysis of colorectal cancer gene expression
profiling studies identifies consistently reported can-
didate biomarkers. Cancer Epidemiology Biomarkers
& Prevention, 17(3):543–552.
Cohen, M. B., Hawkins, J. A., and Witte, D. P. (1998).
Guanylin mrna expression in human intestine and col-
orectal adenocarcinoma. Laboratory investigation,
78(1):101–108.
Ferrero, S., Buffa, R., Pruneri, G., Siccardi, A., Pelagi, M.,
Lee, A., Coggi, G., and Bosari, S. (1995). The preva-
lence and clinical significance of chromogranin a and
secretogranin ii immunoreactivity in colorectal adeno-
carcinomas. Virchows Archiv, 426(6):587–592.
GEO ,2013. Gene Expression Omnibus.
GLOBOCAN, 2008.
Harris, M., Clark, J., Ireland, A., Lomax, J., Ashburner,
M., Foulger, R., Eilbeck, K., Lewis, S., Marshall, B.,
Mungall, C., et al. (2004). The gene ontology (go)
database and informatics resource. Nucleic acids re-
search, 32(Database issue):D258.
Hinoue, T., Weisenberger, D. J., Lange, C. P., Shen, H.,
Byun, H.-M., Van Den Berg, D., Malik, S., Pan,
F., Noushmehr, H., van Dijk, C. M., et al. (2012).
Genome-scale analysis of aberrant dna methylation in
colorectal cancer. Genome Research, 22(2):271–282.
Holla, V. R., Backlund, M. G., Yang, P., Newman,
R. A., and DuBois, R. N. (2008). Regulation
of prostaglandin transporters in colorectal neoplasia.
Cancer Prevention Research, 1(2):93–99.
Holmans, P., Green, E. K., Pahwa, J. S., Ferreira, M. A.,
Purcell, S. M., Sklar, P., et al. (2009). Gene ontology
analysis of gwa study data sets provides insights into
the biology of bipolar disorder. American journal of
human genetics, 85(1):13.
Hong, Y., Downey, T., Eu, K., Koh, P., and Cheah, P.
(2010). A metastasis-prone signature for early-stage
mismatch-repair proficient sporadic colorectal cancer
patients and its implications for possible therapeutics.
Clinical and Experimental Metastasis, 27(2):83–90.
Hui, Y., Kang, T., Xie, L., and Yuan-Yuan, L. (2010).
Digout: Viewing differential expression genes as out-
liers. Journal of Bioinformatics and Computational
Biology, 8(supp01):161–175.
Imai, K., Hirata, S., Irie, A., Senju, S., Ikuta, Y., Yokomine,
K., Harao, M., Inoue, M., Tsunoda, T., Nakatsuru,
S., et al. (2008). Identification of a novel tumor-
associated antigen, cadherin 3/p-cadherin, as a pos-
sible target for immunotherapy of pancreatic, gastric,
and colorectal cancers. Clinical Cancer Research,
14(20):6487–6495.
Jain, K. (2004). Applications of biochips: From diagnos-
tics to personalized medicine. Current opinion in drug
discovery & development, 7(3):285–289.
Jette, C., Peterson, P. W., Sandoval, I. T., Manos, E. J.,
Hadley, E., Ireland, C. M., and Jones, D. A. (2004).
The tumor suppressor adenomatous polyposis coli and
caudal related homeodomain protein regulate expres-
sion of retinol dehydrogenase l. Journal of Biological
Chemistry, 279(33):34397–34405.
Jia, P., Wang, L., Meltzer, H. Y., and Zhao, Z. (2010). Com-
mon variants conferring risk of schizophrenia: a path-
way analysis of gwas data. Schizophrenia research,
122(1):38–42.
Jiang, W., Li, X., Rao, S., Wang, L., Du, L., Li, C., Wu,
C., Wang, H., Wang, Y., and Yang, B. (2008). Con-
structing disease-specific gene networks using pair-
wise relevance metric: application to colon cancer
identifies interleukin 8, desmin and enolase 1 as the
central elements. BMC systems biology, 2(1):72.
Jorissen, R., Lipton, L., Gibbs, P., Chapman, M., Desai,
J., Jones, I., Yeatman, T., East, P., Tomlinson, I.,
Verspaget, H., et al. (2008). Dna copy-number alter-
ations underlie gene expression differences between
microsatellite stable and unstable colorectal cancers.
Clinical Cancer Research, 14(24):8061–8069.
Kinzler, K. W. and Vogelstein, B. (1996). Lessons from
hereditary review colorectal cancer. Cell, 87:159–170.
Kling, K., Kim, F., Cole, M., and McFadden, D. (1999). B-
cell leukemia protein-2 and peptide yy chemotherapy
resistance in colon cancer. The American journal of
surgery, 178(5):411–414.
Lascorz, J., Chen, B., Hemminki, K., and F
¨
orsti, A.
(2011a). Consensus pathways implicated in progno-
sis of colorectal cancer identified through systematic
enrichment analysis of gene expression profiling stud-
ies. PloS one, 6(4):e18867.
Lascorz, J., Hemminki, K., F
¨
orsti, A., et al. (2011b). Sys-
tematic enrichment analysis of gene expression profil-
ing studies identifies consensus pathways implicated
in colorectal cancer development. Journal of carcino-
genesis, 10(1):7.
Li, P., Lin, J., Marszlowicz, G., Valentino, M., Chang, C.,
Schulz, S., Pitari, G., and Waldman, S. (2009). Gcc
signaling in colorectal cancer: Is colorectal cancer a
paracrine deficiency syndrome? Drug news & per-
spectives, 22(6):313.
Masaki, T., Matsuoka, H., Sugiyama, M., Abe, N., Goto,
A., Sakamoto, A., and Atomi, Y. (2001). Matrilysin
(mmp-7) as a significant determinant of malignant po-
tential of early invasive colorectal carcinomas. British
journal of cancer, 84(10):1317.
GeneOntologyAnalysisofColorectalCancerBiomarkersProbedwithAffymetrixandIlluminaMicroarrays
405