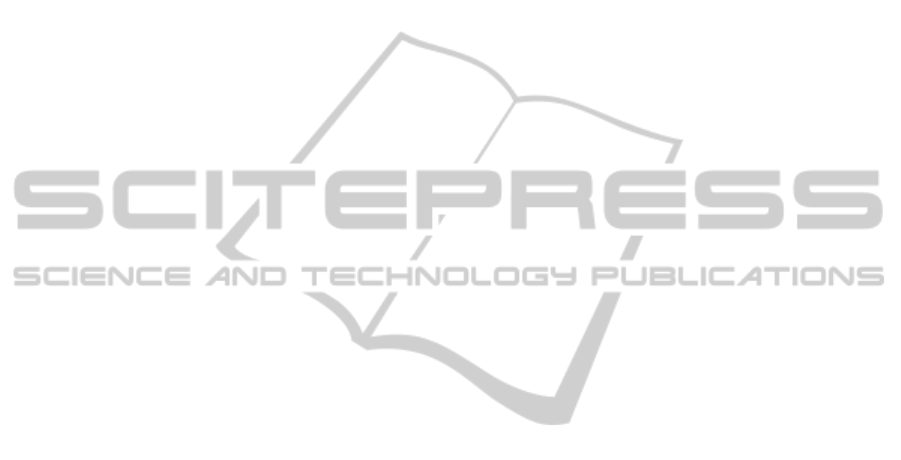
when the number of inputs varies over time. We
then implemented and utilized this network to
extract knowledge from realistic events occurring
within a smart home environment. A set of realistic
synthesized training and testing data have been
employed to observe different scenarios. We show
the structural modifications of the network when the
number of inputs changes for the network during the
training phase. We also show the impact of
removing event inputs from the network during
different testing phases. The results show that the
network has the ability to adapt to the dynamics of
the environment and show its cognitive capability.
ACKNOWLEDGEMENTS
This work is partially supported by the EU FP7
RUBICON project (contract no. 269914) –
www.fp7rubicon.eu.
REFERENCES
Alam, M. S., Reaz, M. B. I., and Ali, M. A. M., 2012.
SPEED: An inhabitant activity prediction algorithm
for smart homes. IEEE Transactions on Systems, Man,
and Cybernetics—Part A: Systems and Humans,
42(4), 985-990.
Bregman, D., 2010. Smart home intelligence - the ehome
that learns. International journal of smart home, 4(4).
Chen, L., and Nugent, C. D., 2010. Situation aware
cognitive assistance in smart homes. Journal of
Mobile Multimedia, 6(3), 263-280.
Chen, L., Nugent, C. D., and Wang, H., 2012. A
knowledge-driven approach to activity recognition in
smart homes. IEEE Transactions on Knowledge and
Data Engineering, 24(6), 961-974.
Chen, Y. H., Lu, C. H., Hsu, K. C., Fu, L. C., Yeh, Y. J.,
and Kuo, L. C., 2009. Preference model assisted
activity recognition learning in a smart home
environment. IEEE/RSJ International Conference on
Intelligent Robots and Systems, 4657 - 4662.
Gaddam, A., Mukhopadhyay, S. C., and Gupta, G. S.,
2011. Elder care based on cognitive sensor network.
IEEE Sensors Journal, 11(3).
Jakkula, V., and Cook, D. J., 2011. Detecting anomalous
sensor events in smart home data for enhancing the
living experience. AAAI Workshop, 33-37.
Leng, G., McGinnity, T. M., and Prasad, G., 2005. An
approach for on-line extraction of fuzzy rules using a
self-organising fuzzy neural network. Fuzzy Sets and
Systems, 150(2), 211-243.
Lin, Z. H., and Fu, L. C., 2007. Multi-user preference
model and service provision in a smart home
environment. IEEE International Conference on
Automation Science and Engineering, 759 – 764.
Mastrogiovanni, F., Sgorbissa, A., and Zaccaria, R., 2010.
A cognitive model for recognizing human behaviours
in smart homes. Ann. Telecommunication, 65, 523–
538.
Ray, A. K., Leng, G., McGinnity, T. M., Coleman, S. A.,
and Maguire, L. P., 2012. Development of cognitive
capabilities for smart home using a self-organizing
fuzzy neural network. 10th IFAC Symposium on Robot
Control, Dubrovnik, Croatia, 447-454.
Roy, P. C., Giroux, S., Bouchard, B., and Bouzouane, A.,
Phua, C., Tolstikov, A., and Biswas, J., 2010.
Possibilistic behavior recognition in smart homes for
cognitive assistance, AAAI Workshop, 53-60.
RUBICON project., 2011. EU FP7 project. FP7 challenge
2, cognitive systems and robotics. Available:
http://www.fp7rubicon.eu.
Son, J. Y., Park, J. H., Moon, K. D., and Lee, Y. H., 2011.
Resource-aware smart home management system by
constructing resource relation graph. IEEE
Transactions on Consumer Electronics, 57(3).
Takagi, T., and Sugeno, M., 1985. Fuzzy identification of
systems and its applications to modeling and control.
IEEE Transactions on Systems, Man, and Cybernetics,
15(1), 116-132.
Wang, W. Y., Chuang, C. C., Lai, Y. S., and Wang, Y. H.,
2005. A context-aware system for smart home
applications. EUC Workshops, LNCS 3823, 298-305.
Youngblood, G. M., Cook, D. J., and Holder, L. B., 2005.
Managing adaptive versatile environments. Pervasive
and Mobile Computing, 1(4), 373-403.
Zhang, S., McClean, S. I., and Scotney, B. W., 2012.
Probabilistic learning from incomplete data for
recognition of activities of daily living in smart homes.
IEEE Transactions on Information Technology in
Biomedicine, 16(3), 454-462.
Zheng, H., Wang, H., and Black, N., 2008. Human
activity detection in smart home environment with self-
adaptive neural networks. IEEE ICNSC, 1505–1510.
IJCCI2013-InternationalJointConferenceonComputationalIntelligence
514