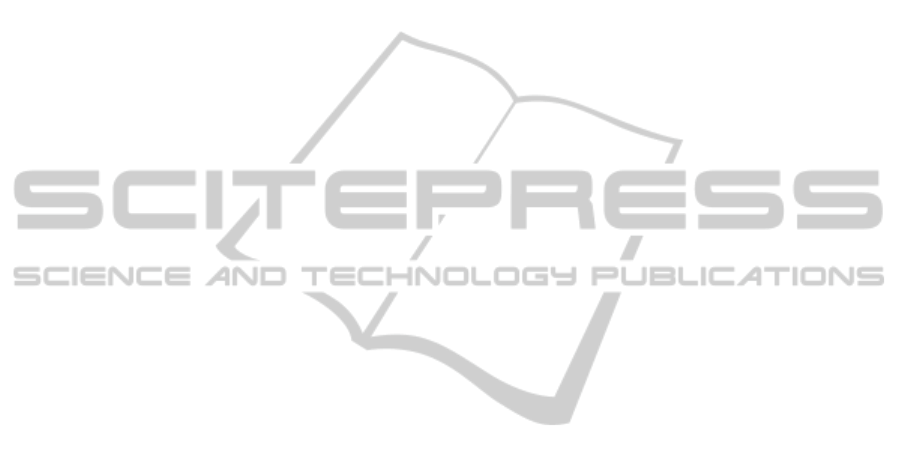
2 BUSINESS ANALYTICS
OF EXCEPTIONS
Modern management system, such as ERP, records
business data in large volume, but overloaded
information poses a problem for human decision
maker, as it confounds him/her from realizing the
true status of the system, causal relationship between
exceptions, and the effect of treatment measures
(Milliken, 1987). To avoid this, reports are
generated by aggregating the data before presented
to the manager. When the manager is examining the
report, he/she is looking for extreme or unexpected
items and try to find explanations using analytics,
i.e. reversing the process of report generation,
drilling down in a managerial model, or using
additional knowledge possibly from external
sources.
The use of analytics in business can be roughly
grouped into two parts. First, descriptive analytics
captures the pattern of systematic emergence in the
company or the environment. The description usual-
ly supports prediction. Examples are the data mining
algorithms like clustering, classification and associa-
tion, applied to identify the events which can possi-
bly lead to disasters. Although descriptive analytics
does not presume any expectations, the analyst usu-
ally looks for “interesting” patterns when interpret-
ing the results. In this process, implicit background
knowledge is applied in searching for (mental) ex-
ceptions (Keil, 2006).
Secondly, diagnostic analytics reason about the
causal relations of those patterns. The goal for this
type of the analysis is to restore or verify the mecha-
nism of a sequence of events (Keil, 2006), e.g. the
operations in the company. The conclusion usually
leads to decisions for adjustment and improvement
of the system. Exemplary analysis questions are
“why the company performance is not as expected”
– for improving performance of the managed sys-
tem, and “why certain exceptions have not been
detected by current monitors” – for adjusting the
management system. Audit analytics also falls in
this category, analysing the risk of fraud and/or
unintentional errors in accounting systems
(Vasarhelyi et al., 2004); (Bay et al., 2006). In the
framework we propose (see Section 2.2), we gener-
alize and combine these two types to the detection
and the diagnosis phases in an integrated process of
business analytics.
We argue that business analytics is a strategic
important process of organizational learning that
extends the philosophy of "management by excep-
tion". The importance of analytics lies in the neces-
sity of "meta-control" to cope with the internal and
external changes. The management system of the
company (ERP) monitors and controls the business
processes, which deliver value to customers and
form competitive competence. It automates the rou-
tine tasks of detecting and treating operational ex-
ceptions, because the business knowledge are codi-
fied into the build-in controls of the system (in form
of business rules or constraints) in a “plan-do-check-
adjust” cycle. With automation, management sys-
tems can help with handling these routine tasks in
large volume data (big data), e.g. managing thou-
sands of accounts in finance and cost accounting
systems. However, their monitor-control capability
is limited to the codified rules, so they cannot deal
with the “new” changes or the exceptions out-of-
scope of the rules. These exceptions are left to the
responsibility of human managers. Though the
“new” exceptions are on a higher system level than
the management system ergo not directly visible,
they affect the performance of the managed system
(the company): therefore, they must be detectable by
analysing the data collected / generated by current
management system. The analysis results in new
business knowledge that equips the management
system for controlling similar exceptions in the
future. Ideally, the managers hope to continuously
meta-control the management system, automating
the process using BI (Vasarhelyi et al., 2004).
2.1 BI Supported Business Analytics
Business Intelligence is the collection of procedures
to reduce the volume of information that the manag-
er need to take into account when making decision.
The information-reduction is done by organising
(extract-transform-load, ETL) transactional data into
a multi-dimensional database (data warehouse or
OLAP), in which large volume of operational details
can be abstracted, aggregated or computed into
business reports, using BI techniques (see Figure 1).
This process involves both the managerial model
and the technical model of information organization.
On one hand, the organising of information is in
essence driven by managerial purpose, i.e. the man-
agerial model. For example, the accounting process,
which in general is a BI process, aggregates transac-
tion records in various documents such as journals,
general ledgers and financial statements for operat-
ing, financing and investing purposes respectively
(Bay et al., 2006). The organization of these docu-
ments codifies the managerial model. For instance,
the general ledger, recorded using double-entry
book-keeping, is a codified management system
DetectingandExplainingBusinessExceptionsforRiskAssessment
531